Sparse estimation of parameter support sets for generalized vector autoregressions by resampling and model aggregation
arXiv (Cornell University)(2023)
摘要
The central problem we address in this work is estimation of the parameter support set S, the set of indices corresponding to nonzero parameters, in the context of a sparse parametric likelihood model for count-valued multivariate time series. We develop a computationally-intensive algorithm that performs the estimation by aggregating support sets obtained by applying the LASSO to data subsamples. Our approach is to identify several well-fitting candidate models and estimate S by the most frequently-used parameters, thus \textit{aggregating} candidate models rather than selecting a single candidate deemed optimal in some sense. While our method is broadly applicable to any selection problem, we focus on the generalized vector autoregressive model class, and in particular the Poisson case, due to (i) the difficulty of the support estimation problem due to complex dependence in the data, (ii) recent work applying the LASSO in this context, and (iii) interesting applications in network recovery from discrete multivariate time series. We establish benchmark methods based on the LASSO and present empirical results demonstrating the superior performance of our method. Additionally, we present an application estimating ecological interaction networks from paleoclimatology data.
更多查看译文
关键词
sparse estimation,autoregressions,parameter support sets,generalized vector
AI 理解论文
溯源树
样例
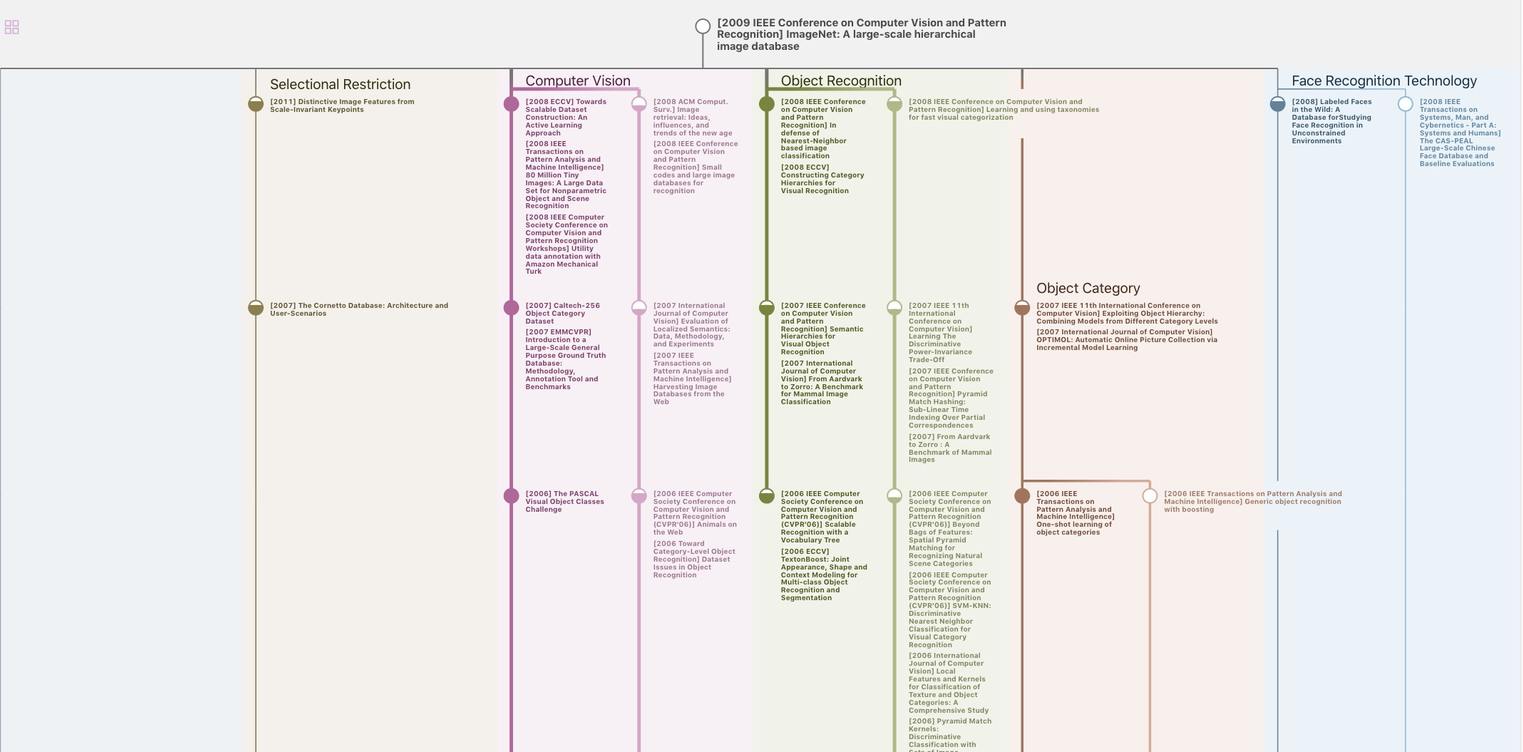
生成溯源树,研究论文发展脉络
Chat Paper
正在生成论文摘要