Forecasting Early with Meta Learning
CoRR(2023)
摘要
In the early observation period of a time series, there might be only a few historic observations available to learn a model. However, in cases where an existing prior set of datasets is available, Meta learning methods can be applicable. In this paper, we devise a Meta learning method that exploits samples from additional datasets and learns to augment time series through adversarial learning as an auxiliary task for the target dataset. Our model (FEML), is equipped with a shared Convolutional backbone that learns features for varying length inputs from different datasets and has dataset specific heads to forecast for different output lengths. We show that FEML can meta learn across datasets and by additionally learning on adversarial generated samples as auxiliary samples for the target dataset, it can improve the forecasting performance compared to single task learning, and various solutions adapted from Joint learning, Multi-task learning and classic forecasting baselines.
更多查看译文
关键词
Early Time series forecasting, Meta Learning
AI 理解论文
溯源树
样例
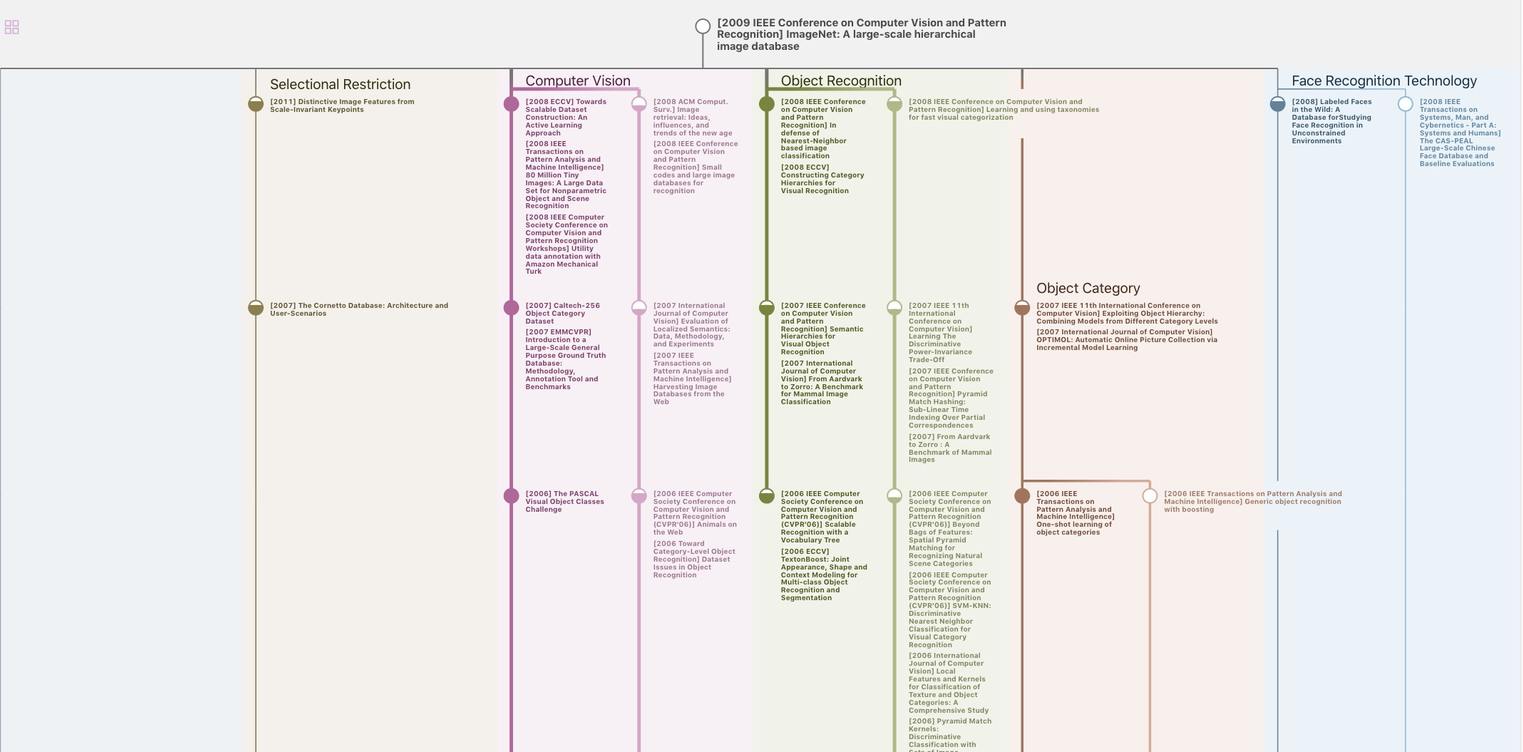
生成溯源树,研究论文发展脉络
Chat Paper
正在生成论文摘要