DIYlandcover: Crowdsourcing the creation of systematic, accurate landcover maps
crossref(2015)
摘要
Accurate landcover maps are fundamental to understanding socio-economic and environmental patterns and processes, but existing datasets contain substantial errors. Crowdsourcing map creation may substantially improve accuracy, but the quality and representativeness of crowdsourced data is hard to verify. We present an open-sourced platform, DIYlandcover, that systematically serves samples of high resolution imagery to an online job market, where workers delineate landcover features of interest. Worker mapping skill is frequently assessed, providing estimates of overall map accuracy and a basis for performance-based payments. An initial trial of DIYlandcover showed that novice workers delineated South African cropland with 91% accuracy, which exceeds current generation global landcover products, while capturing important field geometry data. Given worker payment costs, large area, wall-to-wall mapping may be cost prohibitive, but a potentially promising use of DIYlandcover is to iteratively train and test emerging computer vision algorithms adapted for landcover mapping.
更多查看译文
AI 理解论文
溯源树
样例
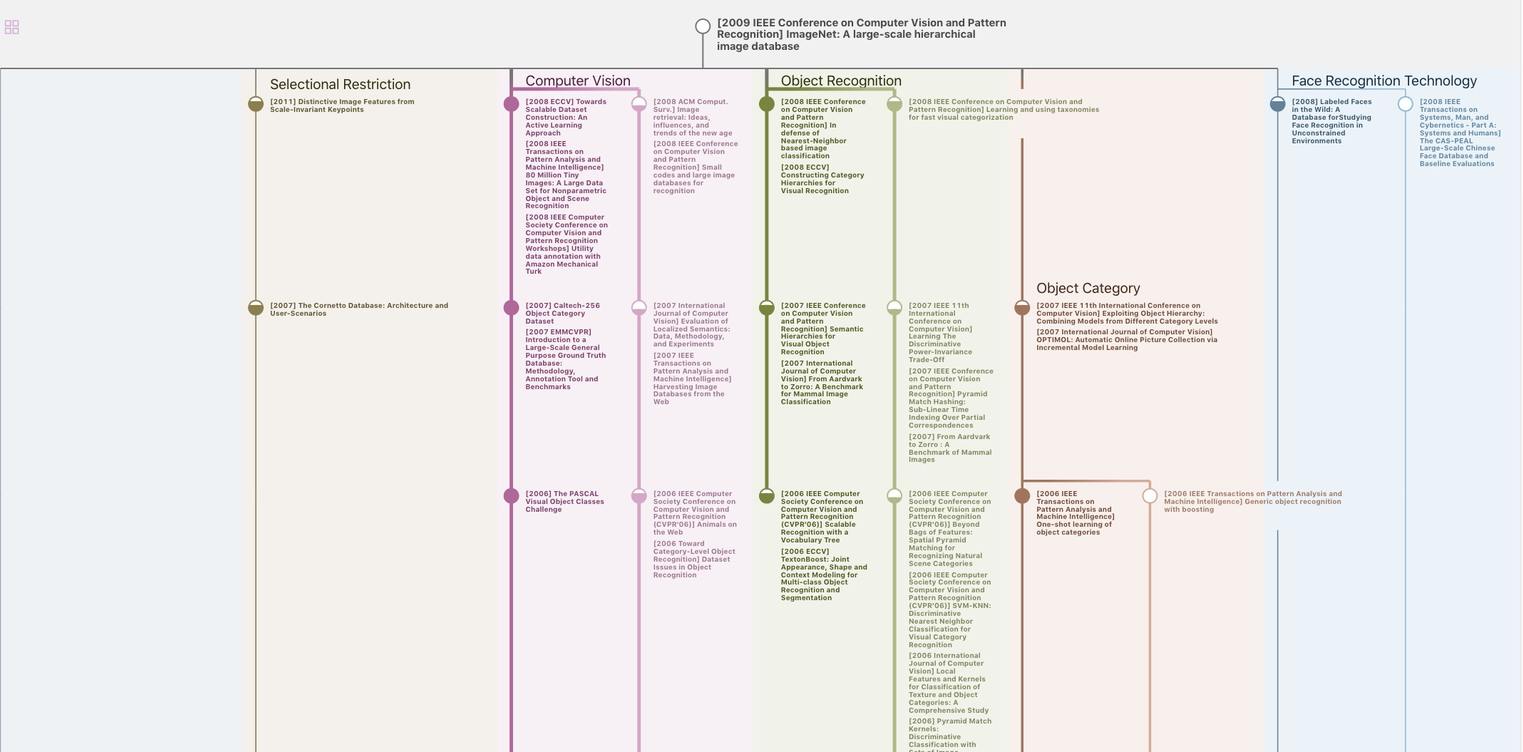
生成溯源树,研究论文发展脉络
Chat Paper
正在生成论文摘要