Sparse model-based clustering of three-way data via lasso-type penalties
arXiv (Cornell University)(2023)
摘要
Mixtures of matrix Gaussian distributions provide a probabilistic framework for clustering continuous matrix-variate data, which are becoming increasingly prevalent in various fields. Despite its widespread adoption and successful application, this approach suffers from over-parameterization issues, making it less suitable even for matrix-variate data of moderate size. To overcome this drawback, we introduce a sparse model-based clustering approach for three-way data. Our approach assumes that the matrix mixture parameters are sparse and have different degree of sparsity across clusters, allowing to induce parsimony in a flexible manner. Estimation of the model relies on the maximization of a penalized likelihood, with specifically tailored group and graphical lasso penalties. These penalties enable the selection of the most informative features for clustering three-way data where variables are recorded over multiple occasions and allow to capture cluster-specific association structures. The proposed methodology is tested extensively on synthetic data and its validity is demonstrated in application to time-dependent crime patterns in different US cities.
更多查看译文
关键词
clustering,model-based,three-way,lasso-type
AI 理解论文
溯源树
样例
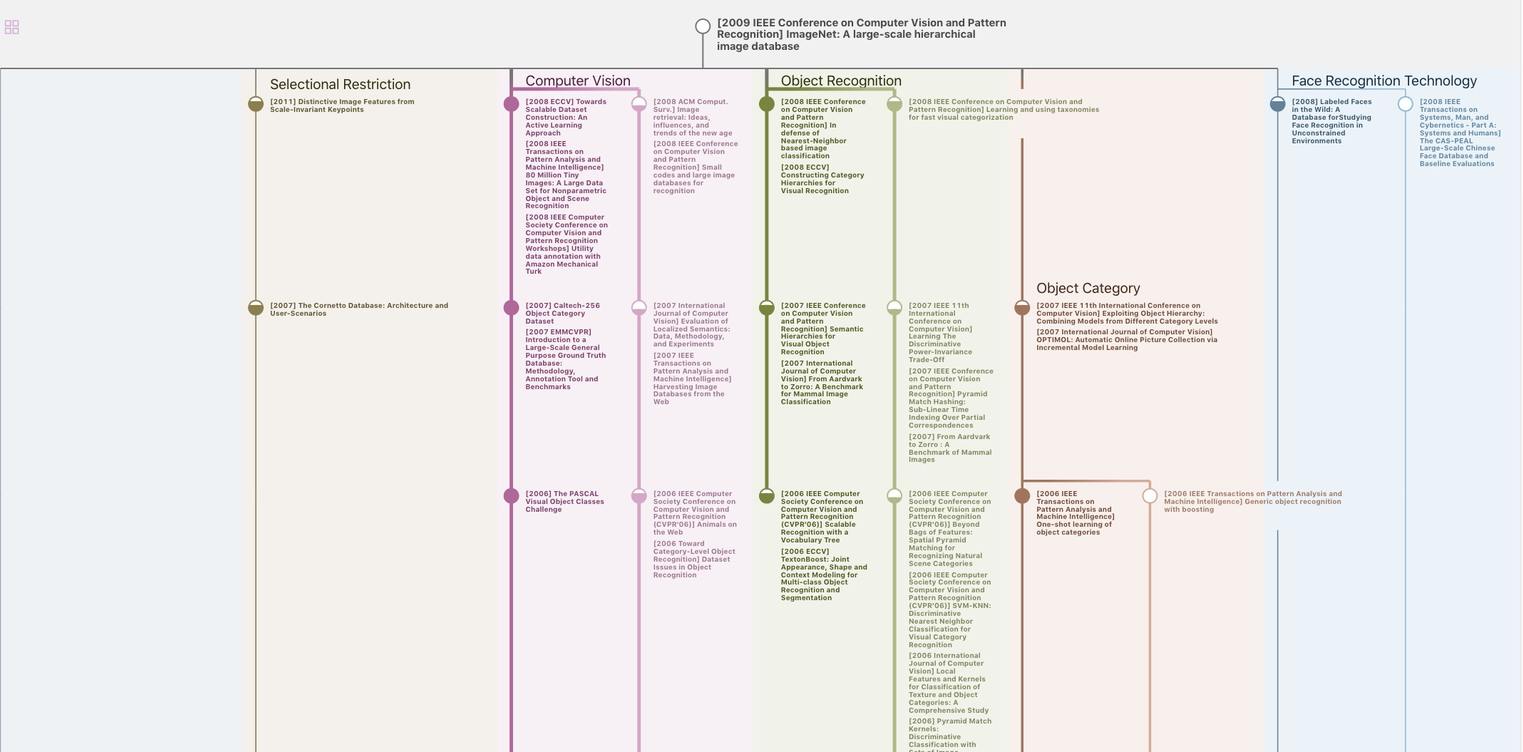
生成溯源树,研究论文发展脉络
Chat Paper
正在生成论文摘要