AdjointDPM: Adjoint Sensitivity Method for Gradient Backpropagation of Diffusion Probabilistic Models
ICLR(2024)
摘要
This paper considers a ubiquitous problem underlying several applications of DPMs, i.e., optimizing the parameters of DPMs when the objective is a differentiable metric defined on the generated contents. Since the sampling procedure of DPMs involves recursive calls to the denoising UNet, naive gradient backpropagation requires storing the intermediate states of all iterations, resulting in extremely high memory consumption. To overcome this issue, we propose a novel method AdjointDPM, which first generates new samples from diffusion models by solving the corresponding probability-flow ODEs. It then uses the adjoint sensitivity method to backpropagate the gradients of the loss to the models' parameters (including conditioning signals, network weights, and initial noises) by solving another augmented ODE. To reduce numerical errors in both the forward generation and gradient backpropagation processes, we further reparameterize the probability-flow ODE and augmented ODE as simple non-stiff ODEs using exponential integration. AdjointDPM can effectively compute the gradients of all types of parameters in DPMs, including the network weights, conditioning text prompts, and noisy states. Finally, we demonstrate the effectiveness of AdjointDPM on several interesting tasks: guided generation via modifying sampling trajectories, finetuning DPM weights for stylization, and converting visual effects into text embeddings.
更多查看译文
关键词
Diffusion Probabilistic Model,Neural ODE,Adjoint Sensitivity Method
AI 理解论文
溯源树
样例
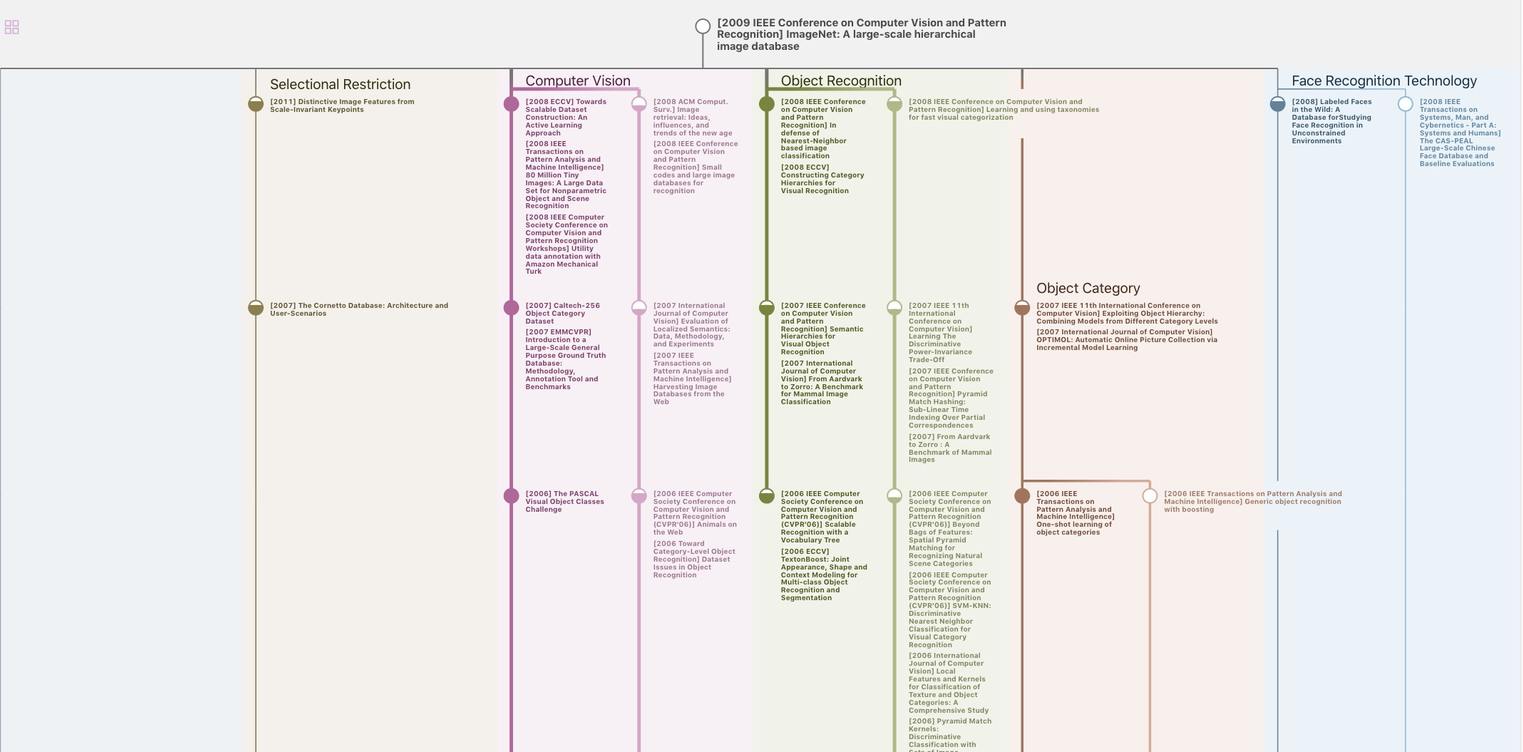
生成溯源树,研究论文发展脉络
Chat Paper
正在生成论文摘要