GeoCoDA: Recognizing and validating structural processes in geochemical data. A workflow on compositional data analysis in lithogeochemistry
APPLIED COMPUTING AND GEOSCIENCES(2024)
摘要
Geochemical data are compositional in nature and are subject to the problems typically associated with data that are restricted to the real non-negative number space with constant-sum constraint, that is, the simplex. Geochemistry can be considered a proxy for mineralogy, comprised of atomically ordered structures that define the placement and abundance of elements in the mineral lattice structure. Based on the innovative contributions of John Aitchison, who introduced the logratio transformation into compositional data analysis, this contribution provides a systematic workflow for assessing geochemical data in a simple and efficient way, such that significant geochemical (mineralogical) processes can be recognized and validated. This workflow, called Geo CoDA and presented here in the form of a tutorial, enables the recognition of processes from which models can be constructed based on the associations of elements that reflect mineralogy. Both the originalcompositional values and their transformation to logratios are considered. These models can reflect rock-forming processes, metamorphism, alteration and ore mineralization. Moreover, machine learning methods, both unsupervised and supervised, applied to an optimized set of subcompositions of the data, provide a systematic, accurate, efficient and defensible approach to geochemical data analysis. The workflow is illustrated on lithogeochemical data from exploration of the Star kimberlite, consisting of a series of eruptions with five recognized phases
更多查看译文
关键词
Geochemistry,Logratio analysis,Classification,Lithologic prediction,Compositional data analysis,Machine learning
AI 理解论文
溯源树
样例
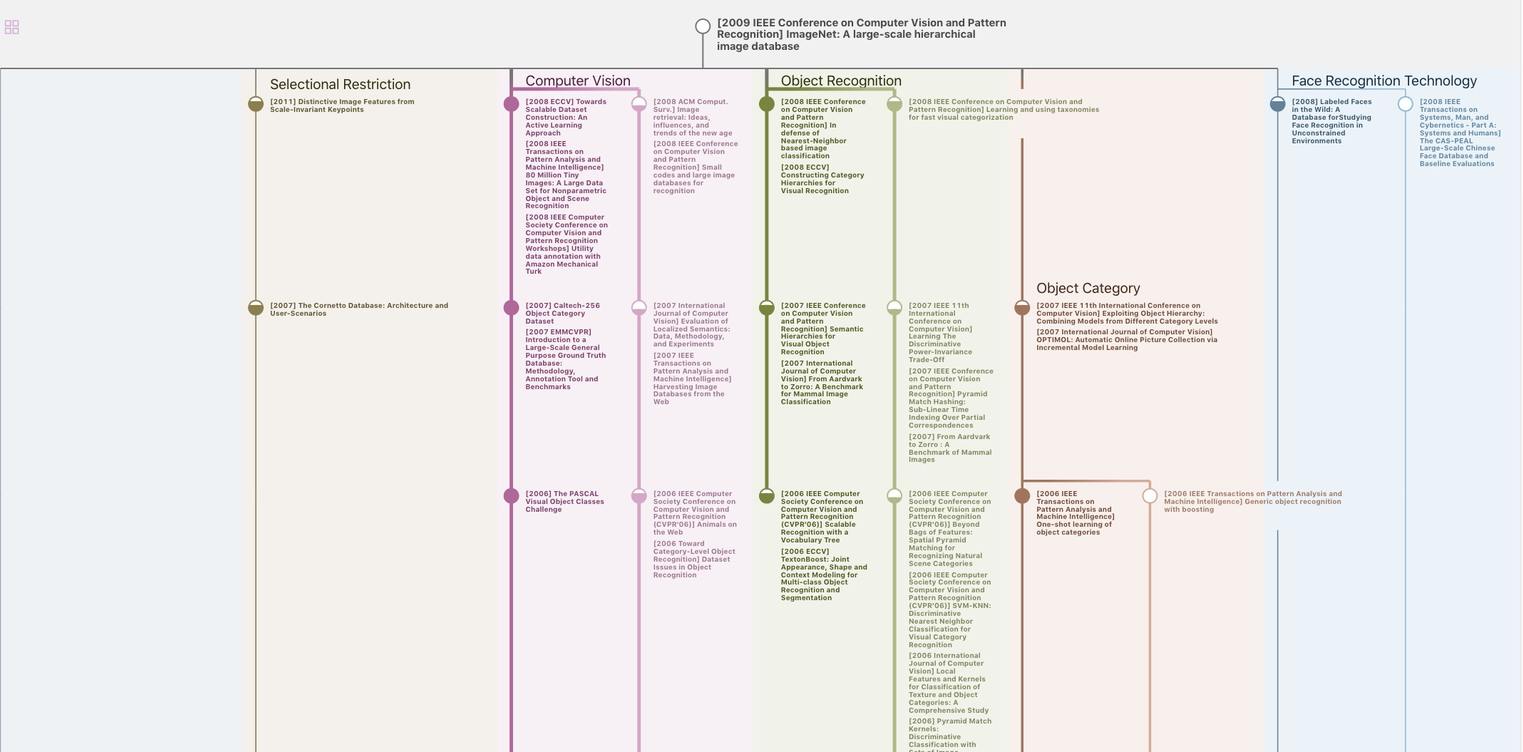
生成溯源树,研究论文发展脉络
Chat Paper
正在生成论文摘要