Fast detection of slender bodies in high density microscopy data
Communications biology(2023)
摘要
Computer-aided analysis of biological microscopy data has seen a massive improvement with the utilization of general-purpose deep learning techniques. Yet, in microscopy studies of multi-organism systems, the problem of collision and overlap remains challenging. This is particularly true for systems composed of slender bodies such as swimming nematodes, swimming spermatozoa, or the beating of eukaryotic or prokaryotic flagella. Here, we develop a end-to-end deep learning approach to extract precise shape trajectories of generally motile and overlapping slender bodies. Our method works in low resolution settings where feature keypoints are hard to define and detect. Detection is fast and we demonstrate the ability to track thousands of overlapping organisms simultaneously. While our approach is agnostic to area of application, we present it in the setting of and exemplify its usability on dense experiments of swimming Caenorhabditis elegans . The model training is achieved purely on synthetic data, utilizing a physics-based model for nematode motility, and we demonstrate the model’s ability to generalize from simulations to experimental videos.
更多查看译文
关键词
Data processing,Machine learning,Software,Life Sciences,general
AI 理解论文
溯源树
样例
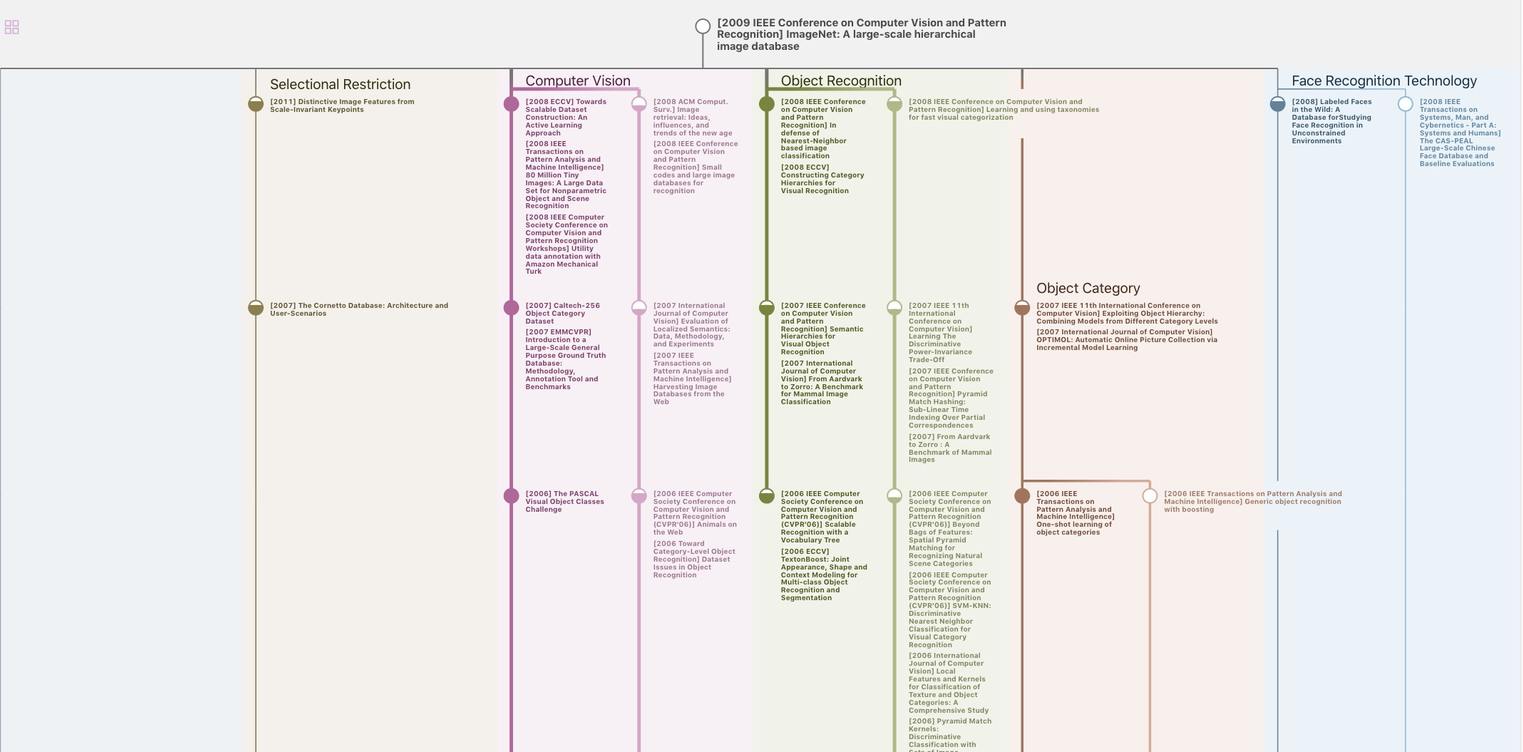
生成溯源树,研究论文发展脉络
Chat Paper
正在生成论文摘要