High-dimensional Probabilistic Fingerprinting in Wireless Sensor Networks based on a Multivariate Gaussian Mixture Model
crossref(2018)
摘要
The extensive deployment of wireless infrastructure provides a low-cost way to track mobile users in indoor environment. This paper demonstrates a prototype model of an accurate and reliable room location awareness system in a real public environment, where three typical problems arise. First, a massive number of access points (APs) can be sensed leading to a high-dimensional classification problem. Second, heterogeneous devices record different received signal strength (RSS) levels due to the variations in chip-set and antenna attenuation. Third, APs are not necessarily visible in every scanning cycle leading to missing data. This paper presents a probabilistic Wi-Fi fingerprinting method in a hidden Markov model (HMM) framework for mobile user tracking. Considering the spatial correlation of the signal strengths from multiple APs, a Multivariate Gaussian Mixture Model (MVGMM) is fitted to model the probability distribution of RSS measurements in each cell. Furthermore, the unseen property of invisible AP has been investigated in this research, and demonstrated the efficiency of differentiation between cells. The proposed system is able to achieve comparable localization performance. The filed test results present a reliable 97% localization room level accuracy of multiple mobile users in a real university campus WiFi network without any prior knowledge of the environment.
更多查看译文
AI 理解论文
溯源树
样例
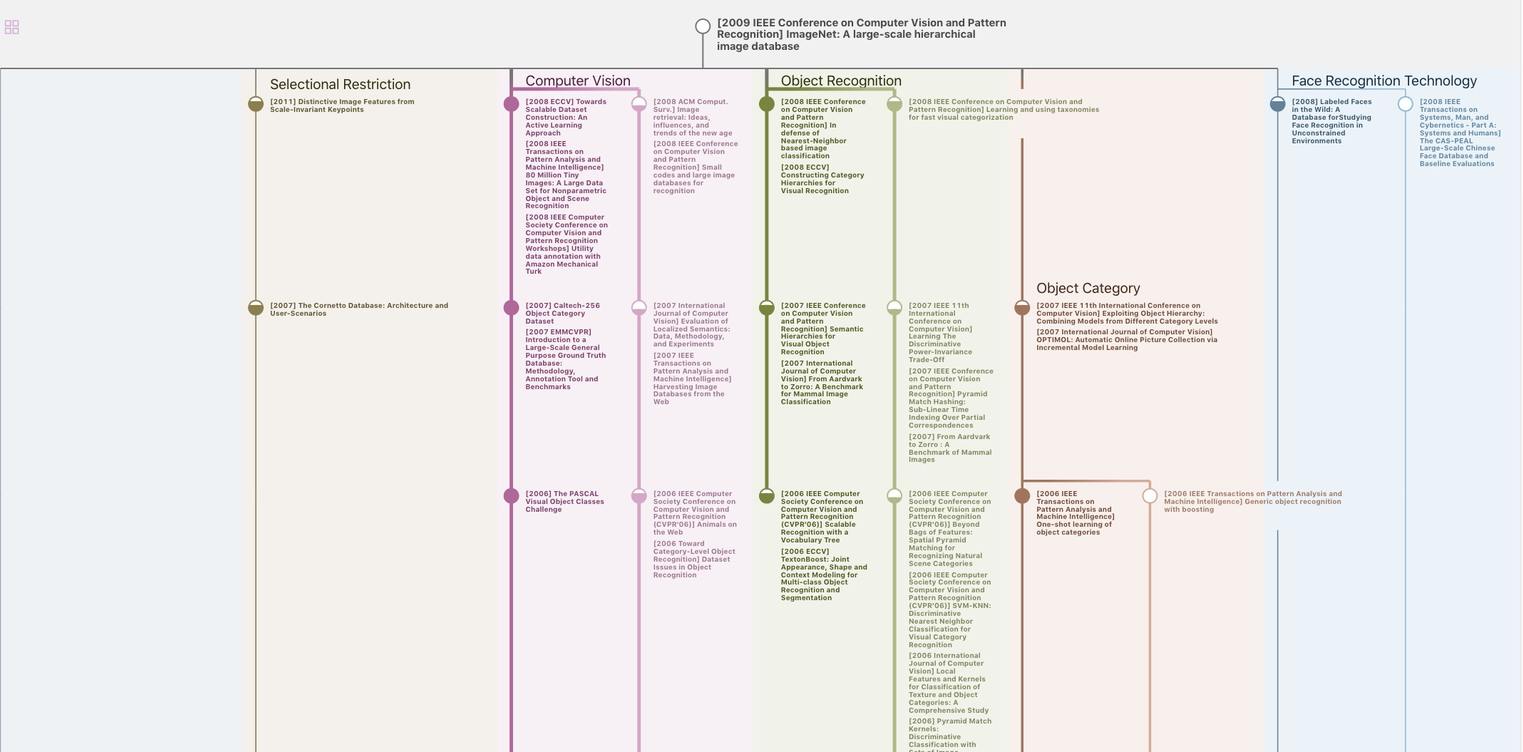
生成溯源树,研究论文发展脉络
Chat Paper
正在生成论文摘要