DeepBoot: Dynamic Scheduling System for Training and Inference Deep Learning Tasks in GPU Cluster
IEEE Transactions on Parallel and Distributed Systems(2023)
摘要
Deep learning tasks (DLT) include training and inference tasks, where training DLTs have requirements on minimizing average job completion time (JCT) and inference tasks need sufficient GPUs to meet real-time performance. Unfortunately, existing work separately deploys multi-tenant training and inference GPU cluster, leading to the high JCT of training DLTs with limited GPUs when the inference cluster is under insufficient GPU utilization due to the periodic inference workload. DeepBoot solves the challenges by utilizing idle GPUs in the inference cluster for the training DLTs. Specifically, 1) DeepBoot designs
adaptive task scaling
(ATS) algorithm to allocate GPUs in the training and inference clusters for training DLTs and minimize the performance loss when reclaiming inference GPUs. 2) DeepBoot implements
auto-fast elastic
(AFE) training based on Pollux to reduce the restart overhead by inference GPU reclaiming. Our implementation on the testbed and large-scale simulation in Microsoft deep learning workload shows that DeepBoot can achieve 32% and 38% average JCT reduction respectively compared with the scheduler without utilizing idle GPUs in the inference cluster.
更多查看译文
关键词
Deep learning system,distributed training,elastic deep learning,GPU cluster scheduling
AI 理解论文
溯源树
样例
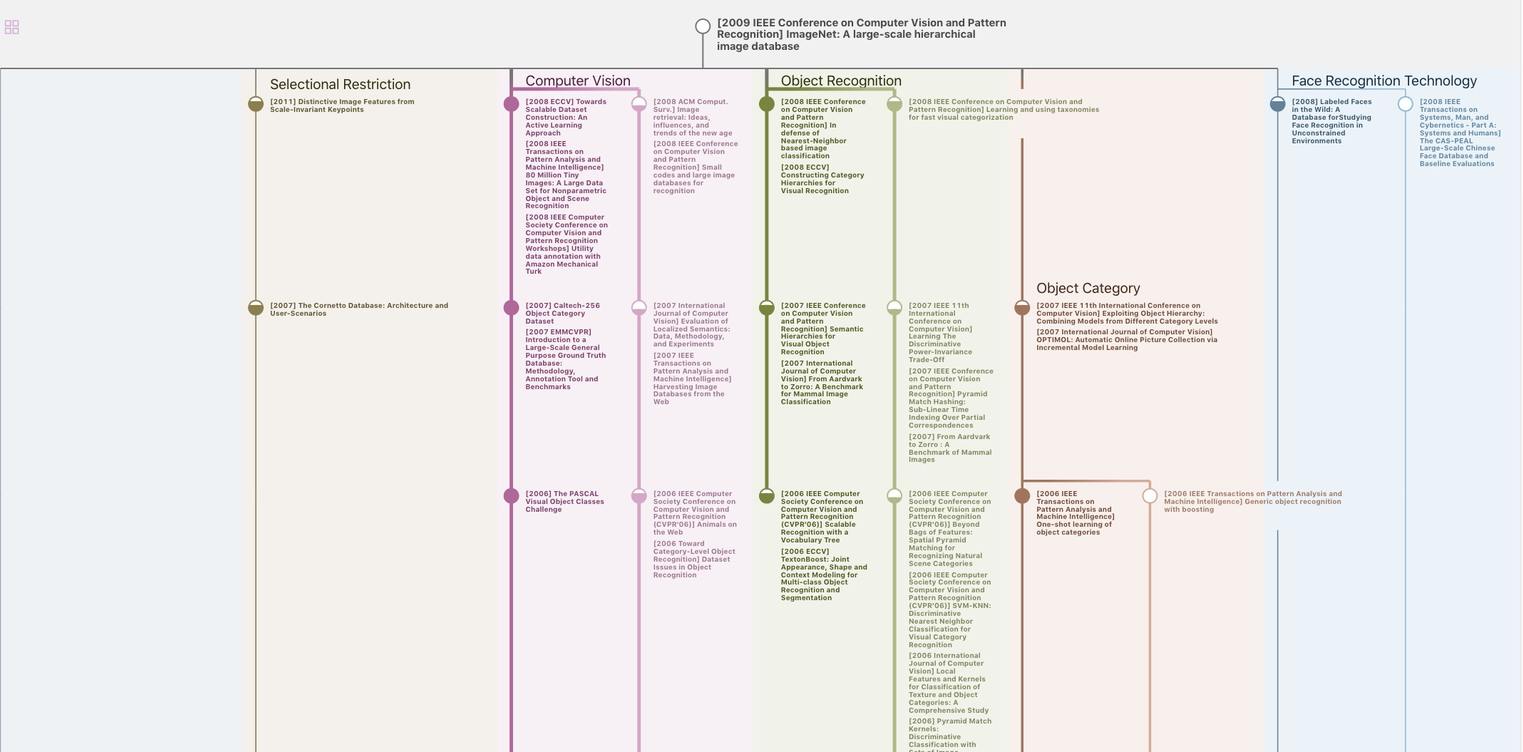
生成溯源树,研究论文发展脉络
Chat Paper
正在生成论文摘要