Neural Latent Aligner: Cross-trial Alignment for Learning Representations of Complex, Naturalistic Neural Data
ICML 2023(2023)
摘要
Understanding the neural implementation of complex human behaviors is one of the major goals in neuroscience. To this end, it is crucial to find a true representation of the neural data, which is challenging due to the high complexity of behaviors and the low signal-to-ratio (SNR) of the signals. Here, we propose a novel unsupervised learning framework, Neural Latent Aligner (NLA), to find well-constrained, behaviorally relevant neural representations of complex behaviors. The key idea is to align representations across repeated trials to learn cross-trial consistent information. Furthermore, we propose a novel, fully differentiable time warping model (TWM) to resolve the temporal misalignment of trials. When applied to intracranial electrocorticography (ECoG) of natural speaking, our model learns better representations for decoding behaviors than the baseline models, especially in lower dimensional space. The TWM is empirically validated by measuring behavioral coherence between aligned trials. The proposed framework learns more cross-trial consistent representations than the baselines, and when visualized, the manifold reveals shared neural trajectories across trials.
更多查看译文
关键词
alignment,learning
AI 理解论文
溯源树
样例
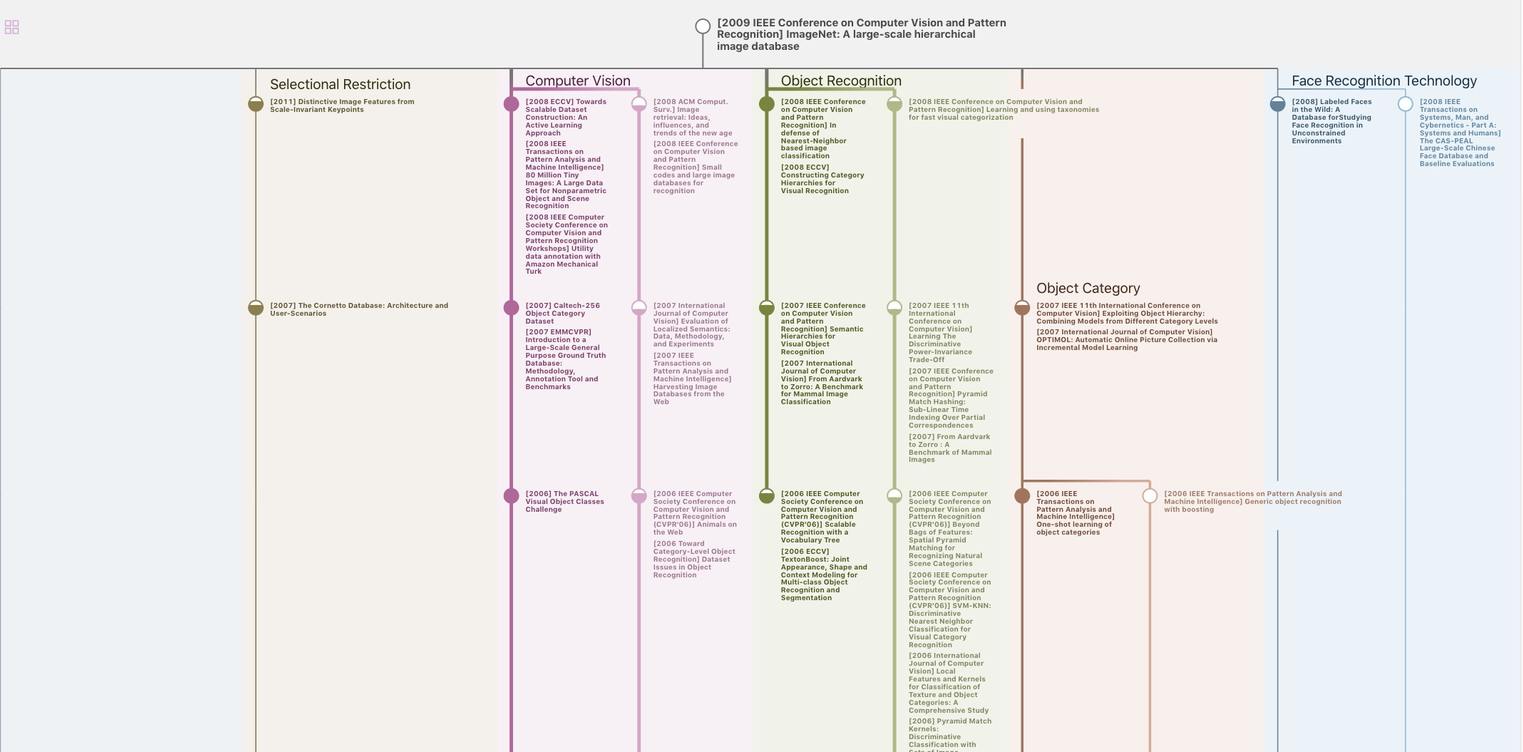
生成溯源树,研究论文发展脉络
Chat Paper
正在生成论文摘要