RACE: Improve Multi-Agent Reinforcement Learning with Representation Asymmetry and Collaborative Evolution
ICML 2023(2023)
摘要
Multi-Agent Reinforcement Learning (MARL) has demonstrated its effectiveness in learning collaboration, but it often struggles with low-quality reward signals and high non-stationarity. In contrast, Evolutionary Algorithm (EA) has shown better convergence, robustness, and signal quality insensitivity. This paper introduces a hybrid framework, Representation Asymmetry and Collaboration Evolution (RACE), which combines EA and MARL for efficient collaboration. RACE maintains a MARL team and a population of EA teams. To enable efficient knowledge sharing and policy exploration, RACE decomposes the policies of different teams controlling the same agent into a shared nonlinear observation representation encoder and individual linear policy representations. To address the partial observation issue, we introduce Value-Aware Mutual Information Maximization to enhance the shared representation with useful information about superior global states. EA evolves the population using novel agent-level crossover and mutation operators, offering diverse experiences for MARL. Concurrently, MARL optimizes its policies and injects them into the population for evolution. The experiments on challenging continuous and discrete tasks demonstrate that RACE significantly improves the basic algorithms, consistently outperforming other algorithms. Our code is available at https://github.com/yeshenpy/RACE.
更多查看译文
AI 理解论文
溯源树
样例
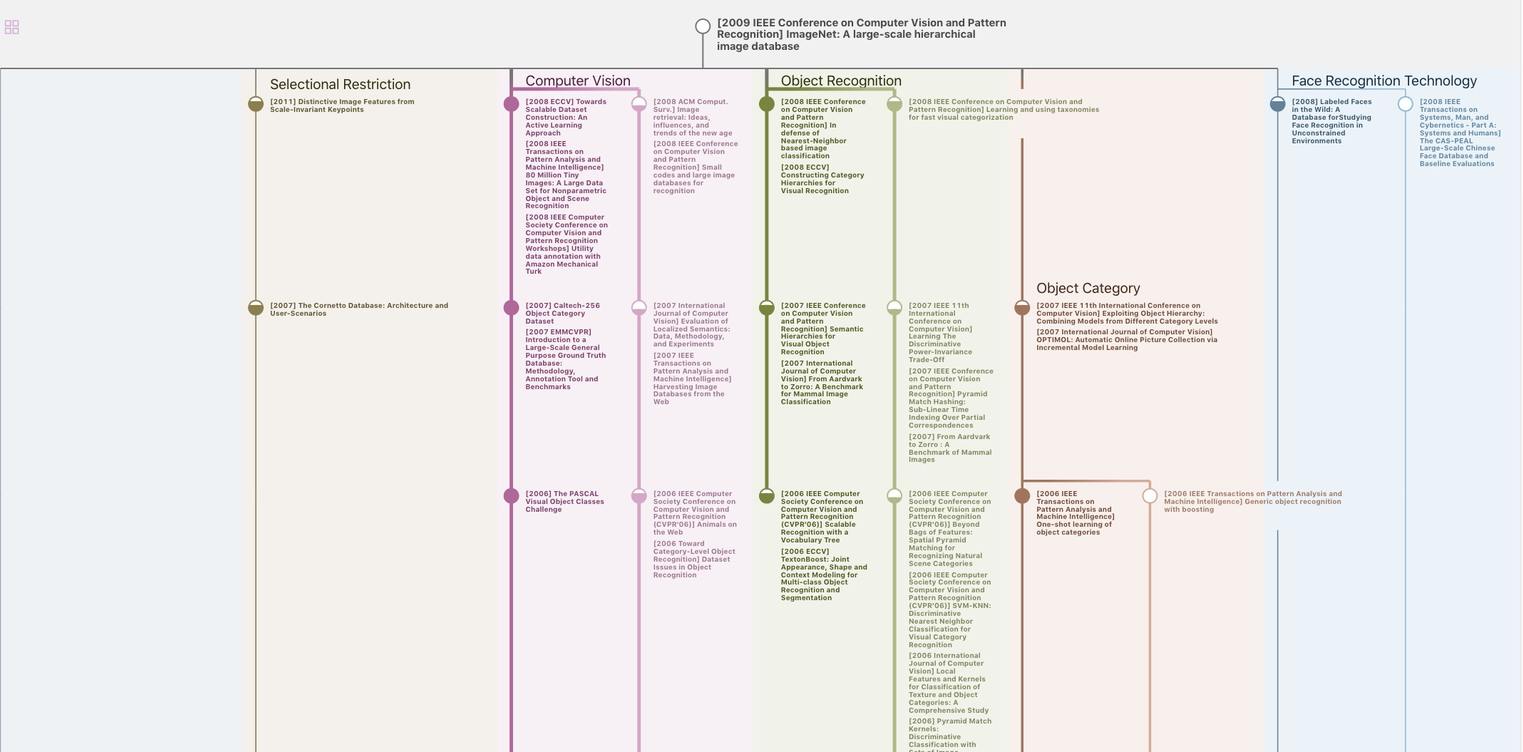
生成溯源树,研究论文发展脉络
Chat Paper
正在生成论文摘要