Homophily-Driven Sanitation View for Robust Graph Contrastive Learning
CoRR(2023)
摘要
We investigate adversarial robustness of unsupervised Graph Contrastive Learning (GCL) against structural attacks. First, we provide a comprehensive empirical and theoretical analysis of existing attacks, revealing how and why they downgrade the performance of GCL. Inspired by our analytic results, we present a robust GCL framework that integrates a homophily-driven sanitation view, which can be learned jointly with contrastive learning. A key challenge this poses, however, is the non-differentiable nature of the sanitation objective. To address this challenge, we propose a series of techniques to enable gradient-based end-to-end robust GCL. Moreover, we develop a fully unsupervised hyperparameter tuning method which, unlike prior approaches, does not require knowledge of node labels. We conduct extensive experiments to evaluate the performance of our proposed model, GCHS (Graph Contrastive Learning with Homophily-driven Sanitation View), against two state of the art structural attacks on GCL. Our results demonstrate that GCHS consistently outperforms all state of the art baselines in terms of the quality of generated node embeddings as well as performance on two important downstream tasks.
更多查看译文
关键词
sanitation view,learning,homophily-driven
AI 理解论文
溯源树
样例
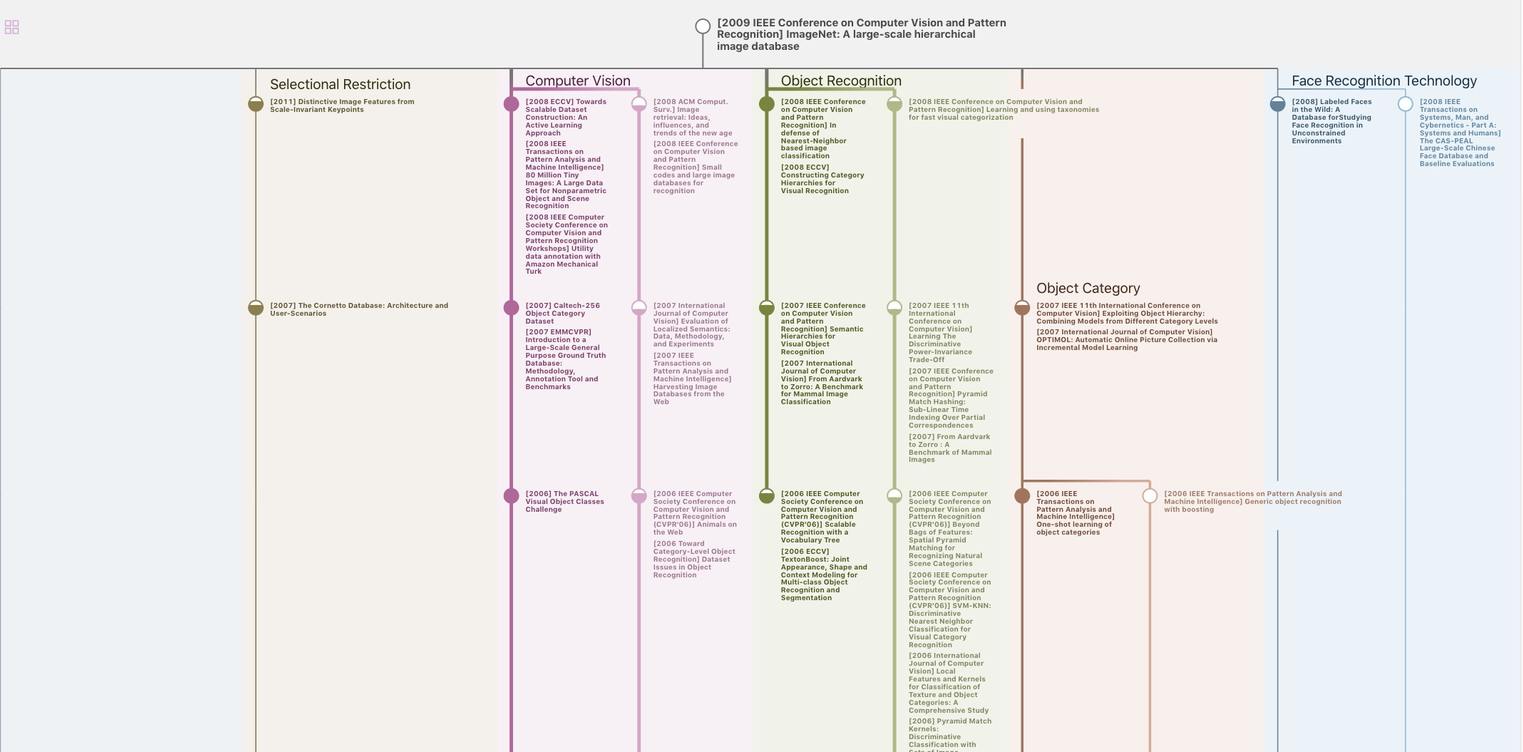
生成溯源树,研究论文发展脉络
Chat Paper
正在生成论文摘要