CLIP-KD: An Empirical Study of CLIP Model Distillation
arxiv(2023)
摘要
Contrastive Language-Image Pre-training (CLIP) has become a promising
language-supervised visual pre-training framework. This paper aims to distill
small CLIP models supervised by a large teacher CLIP model. We propose several
distillation strategies, including relation, feature, gradient and contrastive
paradigms, to examine the effectiveness of CLIP-Knowledge Distillation (KD). We
show that a simple feature mimicry with Mean Squared Error loss works
surprisingly well. Moreover, interactive contrastive learning across teacher
and student encoders is also effective in performance improvement. We explain
that the success of CLIP-KD can be attributed to maximizing the feature
similarity between teacher and student. The unified method is applied to
distill several student models trained on CC3M+12M. CLIP-KD improves student
CLIP models consistently over zero-shot ImageNet classification and cross-modal
retrieval benchmarks. When using ViT-L/14 pretrained on Laion-400M as the
teacher, CLIP-KD achieves 57.5% and 55.4% zero-shot top-1 ImageNet accuracy
over ViT-B/16 and ResNet-50, surpassing the original CLIP without KD by 20.5%
and 20.1% margins, respectively. Our code is released on
https://github.com/winycg/CLIP-KD.
更多查看译文
AI 理解论文
溯源树
样例
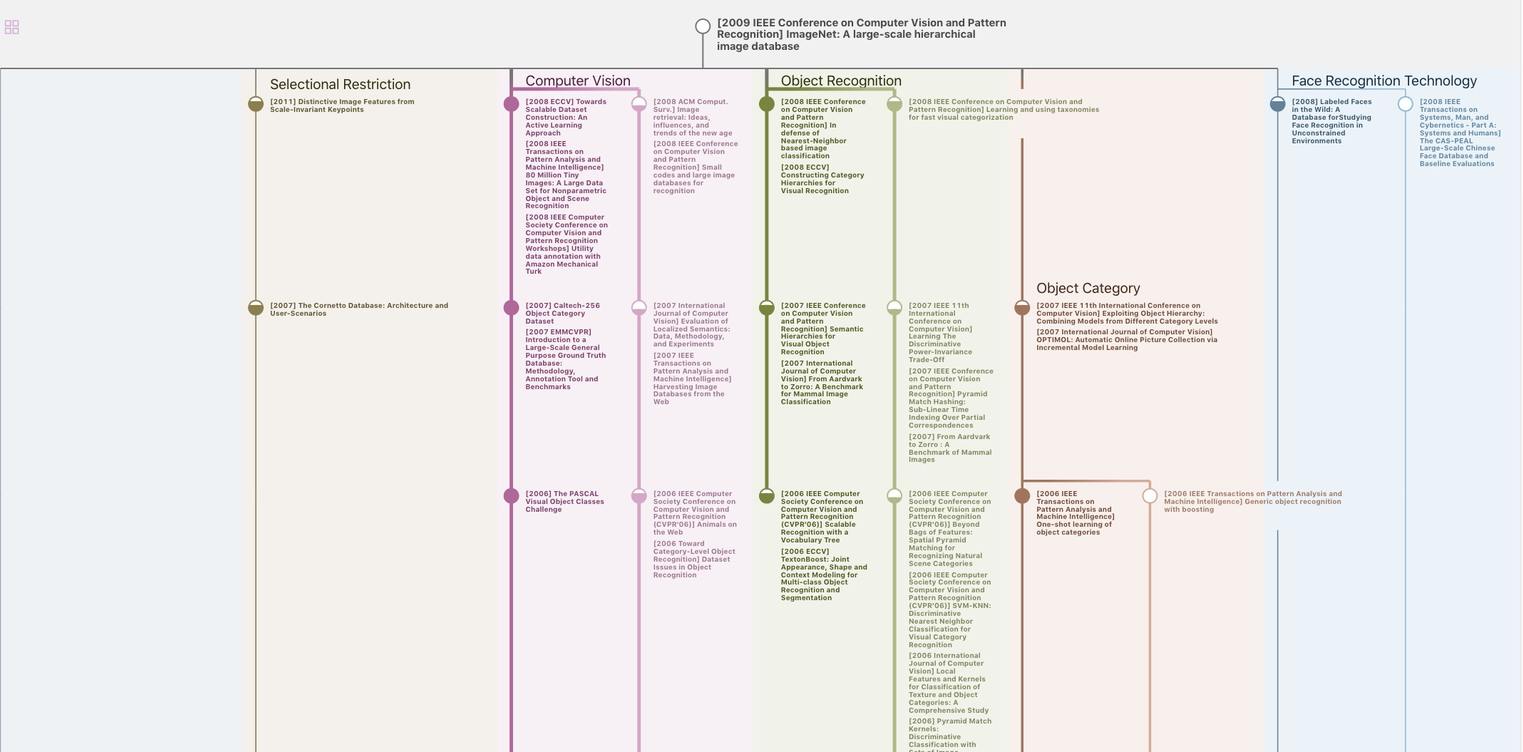
生成溯源树,研究论文发展脉络
Chat Paper
正在生成论文摘要