Joint Dropout: Improving Generalizability in Low-Resource Neural Machine Translation through Phrase Pair Variables
CoRR(2023)
摘要
Despite the tremendous success of Neural Machine Translation (NMT), its performance on low-resource language pairs still remains subpar, partly due to the limited ability to handle previously unseen inputs, i.e., generalization. In this paper, we propose a method called Joint Dropout, that addresses the challenge of low-resource neural machine translation by substituting phrases with variables, resulting in significant enhancement of compositionality, which is a key aspect of generalization. We observe a substantial improvement in translation quality for language pairs with minimal resources, as seen in BLEU and Direct Assessment scores. Furthermore, we conduct an error analysis, and find Joint Dropout to also enhance generalizability of low-resource NMT in terms of robustness and adaptability across different domains
更多查看译文
关键词
phrase pair variables,joint dropout,translation,low-resource
AI 理解论文
溯源树
样例
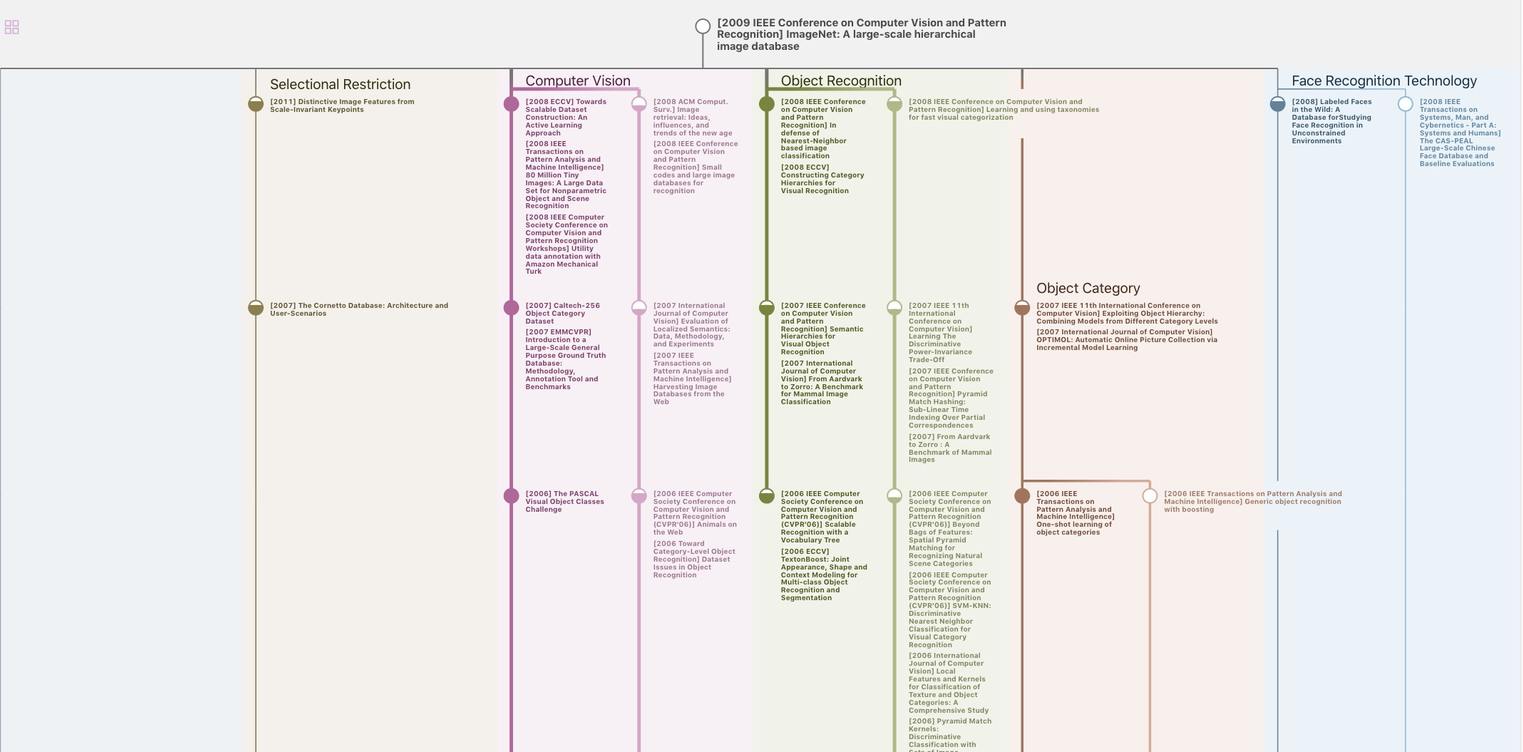
生成溯源树,研究论文发展脉络
Chat Paper
正在生成论文摘要