Automatic Infant Respiration Estimation from Video: A Deep Flow-Based Algorithm and a Novel Public Benchmark
PERINATAL, PRETERM AND PAEDIATRIC IMAGE ANALYSIS, PIPPI 2023(2023)
摘要
Respiration is a critical vital sign for infants, and continuous respiratory monitoring is particularly important for newborns. However, neonates are sensitive and contact-based sensors present challenges in comfort, hygiene, and skin health, especially for preterm babies. As a step toward fully automatic, continuous, and contactless respiratory monitoring, we develop a deep-learning method for estimating respiratory rate and waveform from plain video footage in natural settings. Our automated infant respiration flow-based network (AIRFlowNet) combines video-extracted optical flow input and spatiotemporal convolutional processing tuned to the infant domain. We support our model with the first public annotated infant respiration dataset with 125 videos (AIR-125), drawn from eight infant subjects, set varied pose, lighting, and camera conditions. We include manual respiration annotations and optimize AIRFlowNet training on them using a novel spectral band-pass loss function. When trained and tested on the AIR-125 infant data, our method significantly outperforms other state-of-the-art methods in respiratory rate estimation, achieving a mean absolute error of similar to 2.9 breaths per minute, compared to similar to 4.7-6.2 for other public models designed for adult subjects and more uniform environments. (Our code and the manually annotated respiration (AIR-125) dataset can be found at https://github.com/ostadabbas/Infant-Respiration-Estimation. Supported by MathWorks and NSF-CAREER Grant #2143882.)
更多查看译文
关键词
Infant respiration measurement,Spatio-temporal neural network,Spectral loss,Vital sign monitoring
AI 理解论文
溯源树
样例
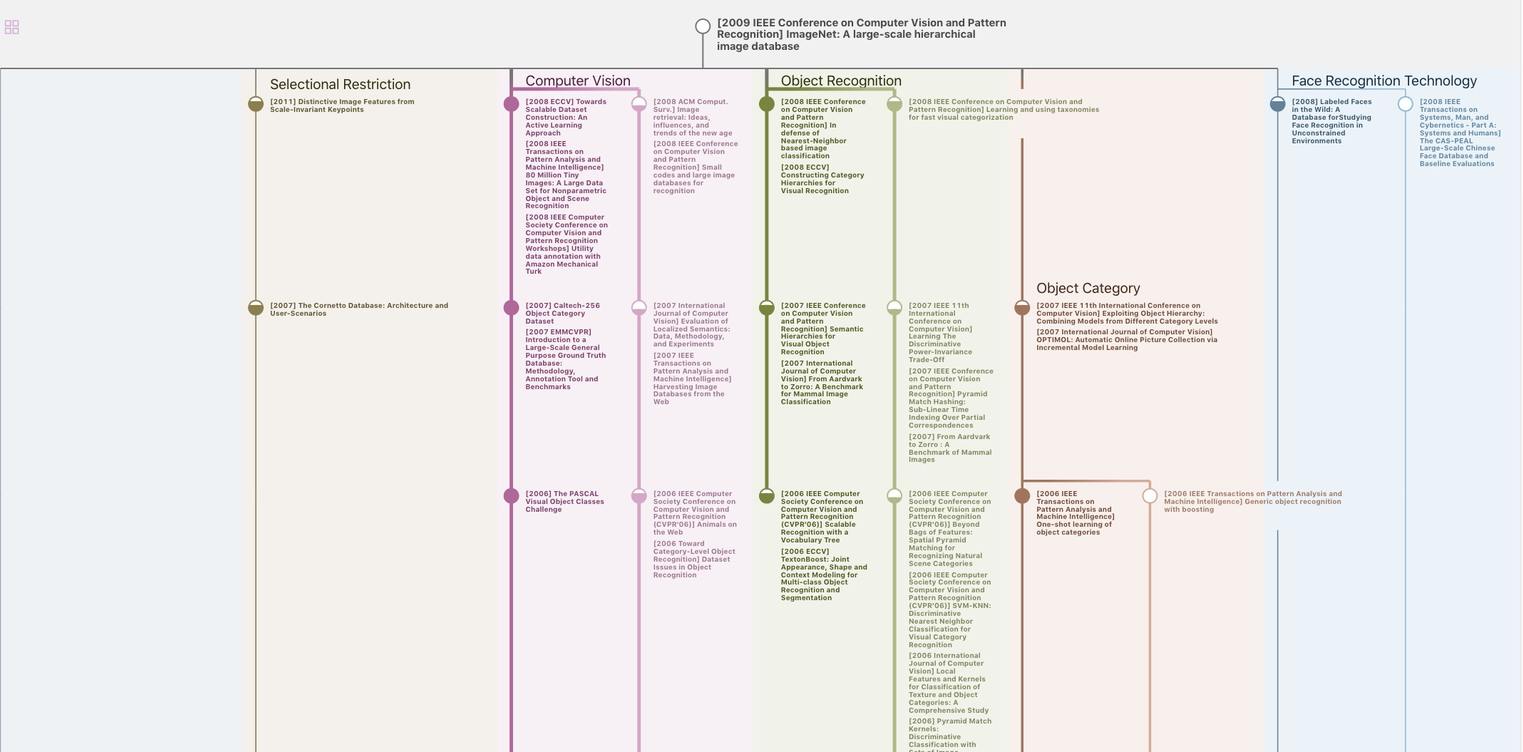
生成溯源树,研究论文发展脉络
Chat Paper
正在生成论文摘要