Prior Based Online Lane Graph Extraction from Single Onboard Camera Image
CoRR(2023)
摘要
The local road network information is essential for autonomous navigation. This information is commonly obtained from offline HD-Maps in terms of lane graphs. However, the local road network at a given moment can be drastically different than the one given in the offline maps; due to construction works, accidents etc. Moreover, the autonomous vehicle might be at a location not covered in the offline HD-Map. Thus, online estimation of the lane graph is crucial for widespread and reliable autonomous navigation. In this work, we tackle online Bird's-Eye-View lane graph extraction from a single onboard camera image. We propose to use prior information to increase quality of the estimations. The prior is extracted from the dataset through a transformer based Wasserstein Autoencoder. The autoencoder is then used to enhance the initial lane graph estimates. This is done through optimization of the latent space vector. The optimization encourages the lane graph estimation to be logical by discouraging it to diverge from the prior distribution. We test the method on two benchmark datasets, NuScenes and Argoverse. The results show that the proposed method significantly improves the performance compared to state-of-the-art methods.
更多查看译文
关键词
Lane Graph,Single Image,Local Network,Road Network,Latent Space,Autonomous Vehicles,Latent Vector,Autonomous Navigation,Loss Function,Extensive Studies,Posterior Probability,Input Image,Uncertainty Estimation,Control Points,Input Sequence,Methods In The Literature,Model Discrimination,Single Vector,Vertices,Reconstruction Loss,Latent Code,Query Vector,Bezier Curve,Maximum Mean Discrepancy,Univariate Normal Distribution,L1 Loss,Transformer Decoder,Softmax Operation,Aerial Images,Gradient Descent
AI 理解论文
溯源树
样例
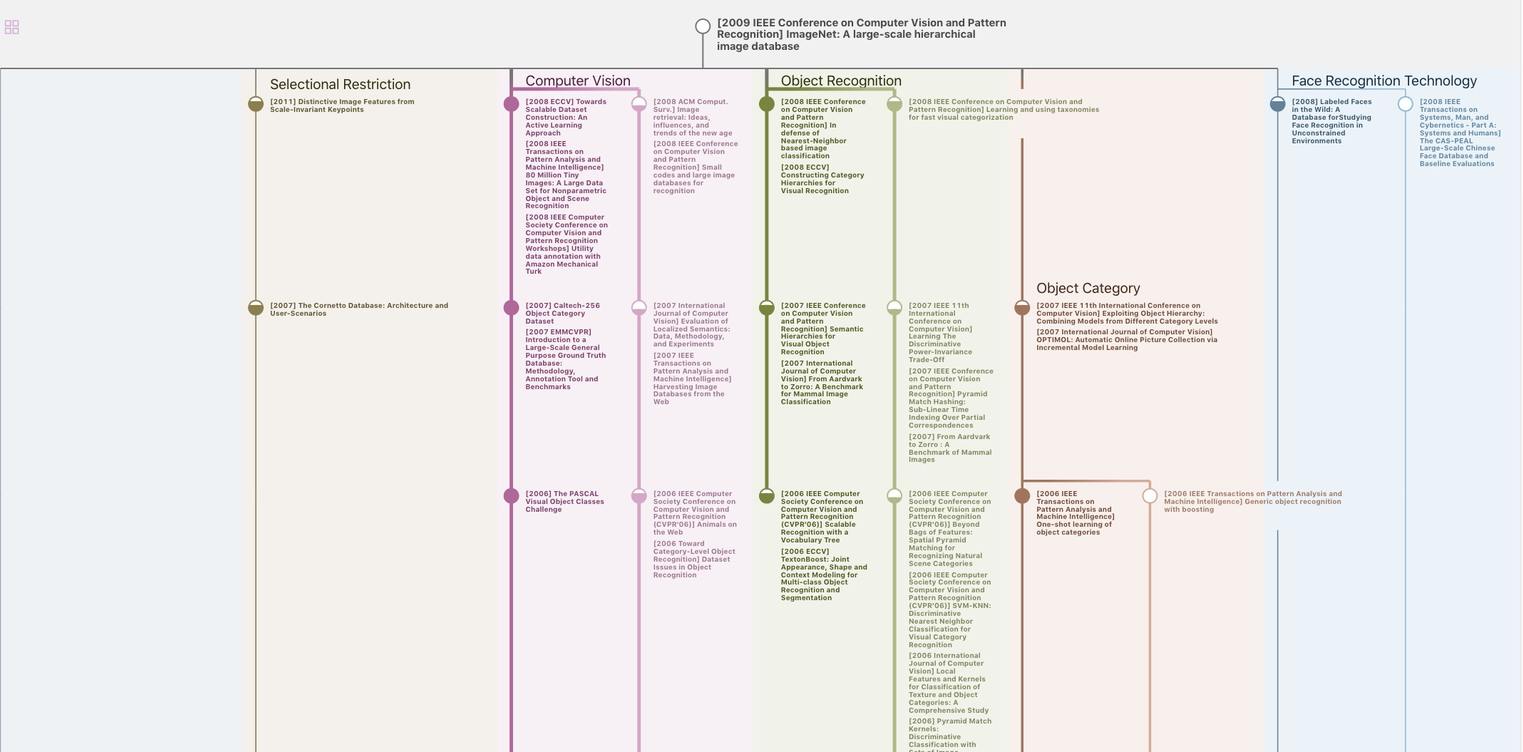
生成溯源树,研究论文发展脉络
Chat Paper
正在生成论文摘要