NormAUG: Normalization-Guided Augmentation for Domain Generalization
IEEE TRANSACTIONS ON IMAGE PROCESSING(2024)
摘要
Deep learning has made significant advancements in supervised learning. However, models trained in this setting often face challenges due to domain shift between training and test sets, resulting in a significant drop in performance during testing. To address this issue, several domain generalization methods have been developed to learn robust and domain-invariant features from multiple training domains that can generalize well to unseen test domains. Data augmentation plays a crucial role in achieving this goal by enhancing the diversity of the training data. In this paper, inspired by the observation that normalizing an image with different statistics generated by different batches with various domains can perturb its feature, we propose a simple yet effective method called NormAUG (Normalization-guided Augmentation). Our method includes two paths: the main path and the auxiliary (augmented) path. During training, the auxiliary path includes multiple sub-paths, each corresponding to batch normalization for a single domain or a random combination of multiple domains. This introduces diverse information at the feature level and improves the generalization of the main path. Moreover, our NormAUG method effectively reduces the existing upper boundary for generalization based on theoretical perspectives. During the test stage, we leverage an ensemble strategy to combine the predictions from the auxiliary path of our model, further boosting performance. Extensive experiments are conducted on multiple benchmark datasets to validate the effectiveness of our proposed method.
更多查看译文
关键词
Training,Training data,Data augmentation,Data models,Feature extraction,Ensemble learning,Task analysis,Normalization-guided augmentation,domain generalization,domain-shift
AI 理解论文
溯源树
样例
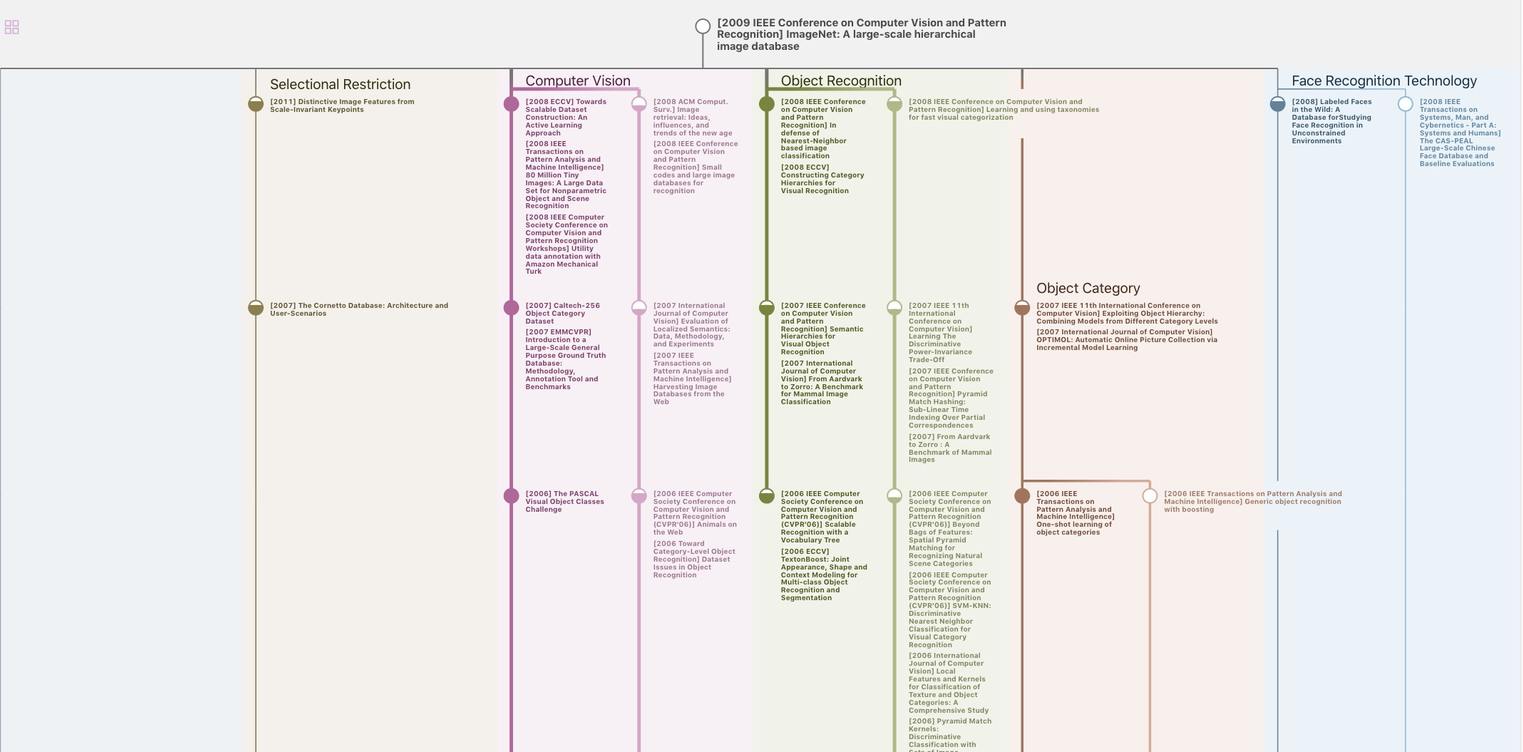
生成溯源树,研究论文发展脉络
Chat Paper
正在生成论文摘要