Estimating entanglement entropy via variational quantum circuits with classical neural networks.
Physical review. E(2024)
摘要
Entropy plays a crucial role in both physics and information science, encompassing classical and quantum domains. In this paper, we present the quantum neural entropy estimator (QNEE), an approach that combines classical neural network (NN) with variational quantum circuits to estimate the von Neumann and Rényi entropies of a quantum state. QNEE provides accurate estimates of entropy while also yielding the eigenvalues and eigenstates of the input density matrix. Leveraging the capabilities of classical NN, QNEE can classify different phases of quantum systems that accompany the changes of entanglement entropy. Our numerical simulation demonstrates the effectiveness of QNEE by applying it to the 1D XXZ Heisenberg model. In particular, QNEE exhibits high sensitivity in estimating entanglement entropy near the phase transition point. We expect that QNEE will serve as a valuable tool for quantum entropy estimation and phase classification.
更多查看译文
AI 理解论文
溯源树
样例
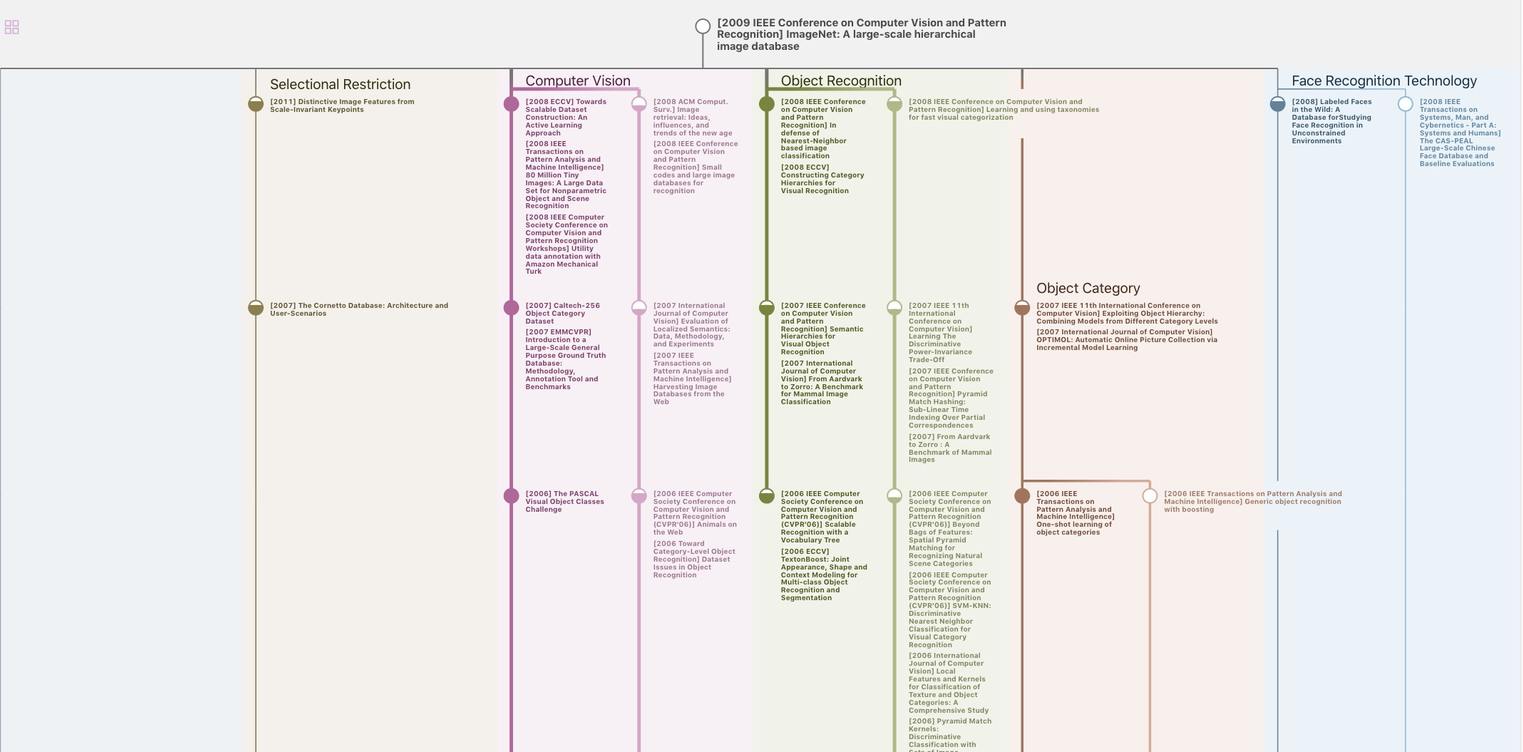
生成溯源树,研究论文发展脉络
Chat Paper
正在生成论文摘要