Decision-Focused Learning: Foundations, State of the Art, Benchmark and Future Opportunities
CoRR(2023)
摘要
Decision-focused learning (DFL) is an emerging paradigm in machine learning which trains a model to optimize decisions, integrating prediction and optimization in an end-to-end system. This paradigm holds the promise to revolutionize decision-making in many real-world applications which operate under uncertainty, where the estimation of unknown parameters within these decision models often becomes a substantial roadblock. This paper presents a comprehensive review of DFL. It provides an in-depth analysis of the various techniques devised to integrate machine learning and optimization models introduces a taxonomy of DFL methods distinguished by their unique characteristics, and conducts an extensive empirical evaluation of these methods proposing suitable benchmark dataset and tasks for DFL. Finally, the study provides valuable insights into current and potential future avenues in DFL research.
更多查看译文
关键词
learning,decision-focused
AI 理解论文
溯源树
样例
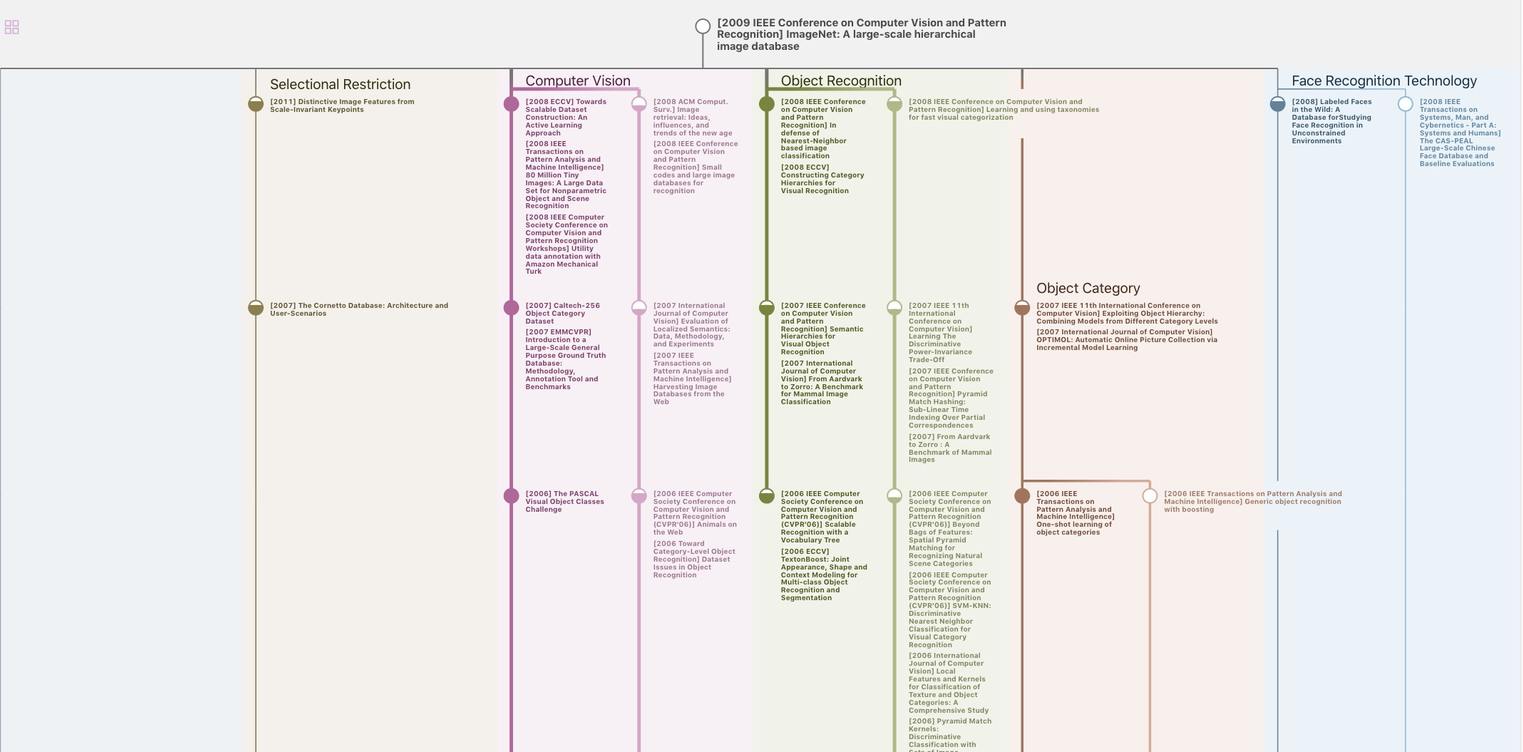
生成溯源树,研究论文发展脉络
Chat Paper
正在生成论文摘要