ClusterSeq: Enhancing Sequential Recommender Systems with Clustering based Meta-Learning
CoRR(2023)
摘要
In practical scenarios, the effectiveness of sequential recommendation systems is hindered by the user cold-start problem, which arises due to limited interactions for accurately determining user preferences. Previous studies have attempted to address this issue by combining meta-learning with user and item-side information. However, these approaches face inherent challenges in modeling user preference dynamics, particularly for "minor users" who exhibit distinct preferences compared to more common or "major users." To overcome these limitations, we present a novel approach called ClusterSeq, a Meta-Learning Clustering-Based Sequential Recommender System. ClusterSeq leverages dynamic information in the user sequence to enhance item prediction accuracy, even in the absence of side information. This model preserves the preferences of minor users without being overshadowed by major users, and it capitalizes on the collective knowledge of users within the same cluster. Extensive experiments conducted on various benchmark datasets validate the effectiveness of ClusterSeq. Empirical results consistently demonstrate that ClusterSeq outperforms several state-of-the-art meta-learning recommenders. Notably, compared to existing meta-learning methods, our proposed approach achieves a substantial improvement of 16-39% in Mean Reciprocal Rank (MRR).
更多查看译文
关键词
sequential recommender systems,clusterseq,clustering
AI 理解论文
溯源树
样例
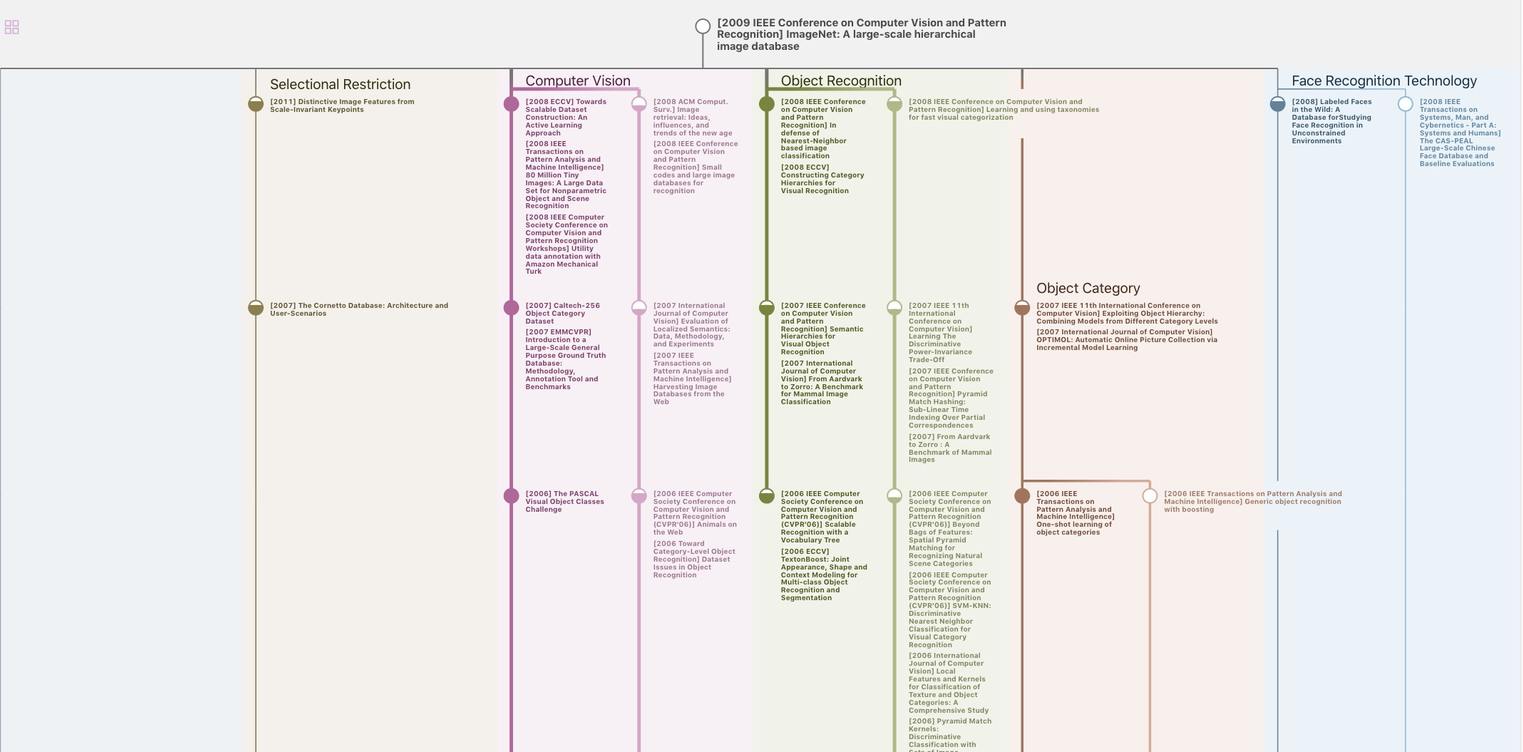
生成溯源树,研究论文发展脉络
Chat Paper
正在生成论文摘要