Learning-based Control for PMSM Using Distributed Gaussian Processes with Optimal Aggregation Strategy
CoRR(2023)
摘要
The growing demand for accurate control in varying and unknown environments has sparked a corresponding increase in the requirements for power supply components, including permanent magnet synchronous motors (PMSMs). To infer the unknown part of the system, machine learning techniques are widely employed, especially Gaussian process regression (GPR) due to its flexibility of continuous system modeling and its guaranteed performance. For practical implementation, distributed GPR is adopted to alleviate the high computational complexity. However, the study of distributed GPR from a control perspective remains an open problem. In this paper, a control-aware optimal aggregation strategy of distributed GPR for PMSMs is proposed based on the Lyapunov stability theory. This strategy exclusively leverages the posterior mean, thereby obviating the need for computationally intensive calculations associated with posterior variance in alternative approaches. Moreover, the straightforward calculation process of our proposed strategy lends itself to seamless implementation in high-frequency PMSM control. The effectiveness of the proposed strategy is demonstrated in the simulations.
更多查看译文
关键词
Gaussian Process Regression,Learning-based Control,Distributed Learning,Optimal Aggregation,Permanent Magnet Synchronous Motor
AI 理解论文
溯源树
样例
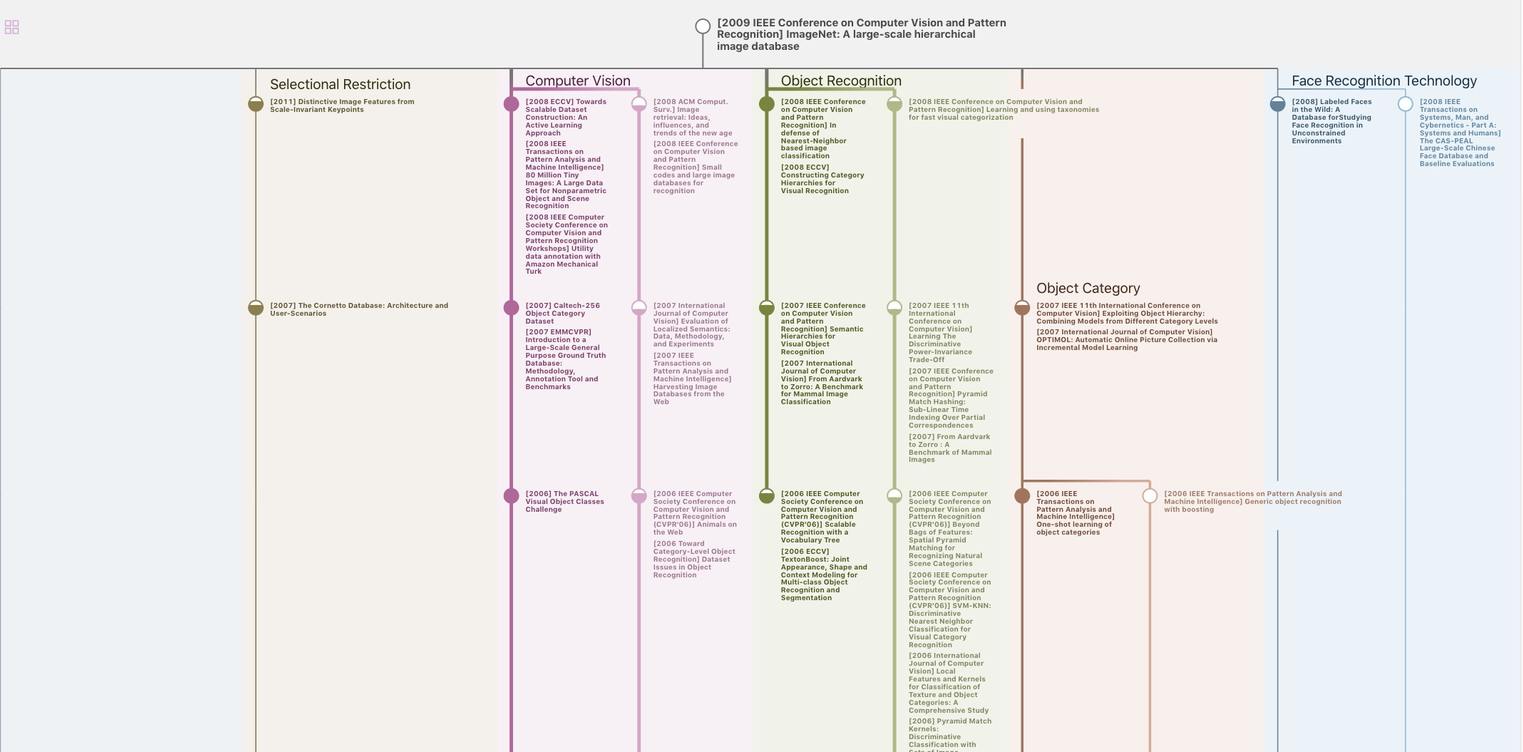
生成溯源树,研究论文发展脉络
Chat Paper
正在生成论文摘要