This is not correct! Negation-aware Evaluation of Language Generation Systems
CoRR(2023)
摘要
Large language models underestimate the impact of negations on how much they change the meaning of a sentence. Therefore, learned evaluation metrics based on these models are insensitive to negations. In this paper, we propose NegBLEURT, a negation-aware version of the BLEURT evaluation metric. For that, we designed a rule-based sentence negation tool and used it to create the CANNOT negation evaluation dataset. Based on this dataset, we fine-tuned a sentence transformer and an evaluation metric to improve their negation sensitivity. Evaluating these models on existing benchmarks shows that our fine-tuned models outperform existing metrics on the negated sentences by far while preserving their base models' performances on other perturbations.
更多查看译文
关键词
language generation
AI 理解论文
溯源树
样例
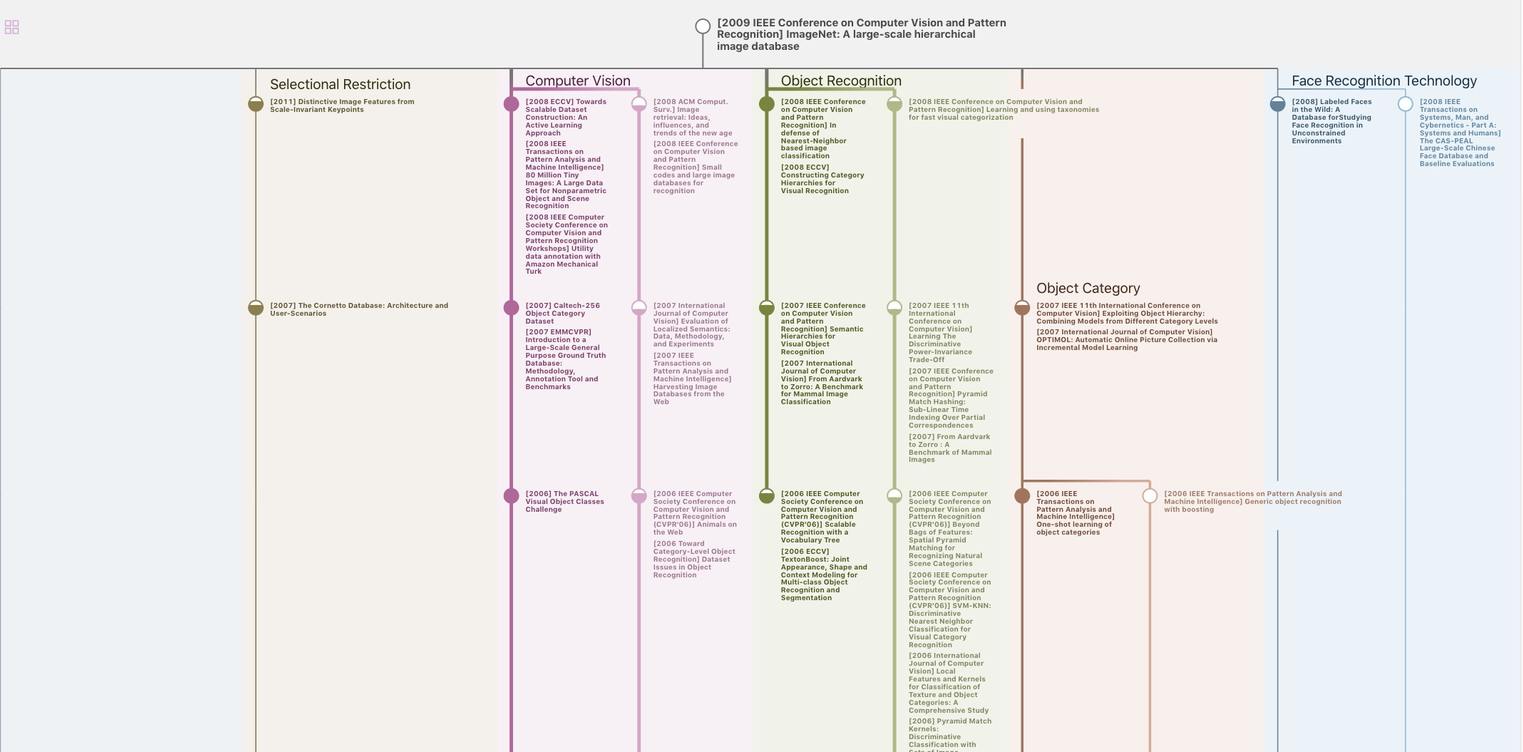
生成溯源树,研究论文发展脉络
Chat Paper
正在生成论文摘要