A Transmission Design in Dynamic Heterogeneous V2V Networks Through Multi-Agent Deep Reinforcement Learning
China Communications(2023)
摘要
In highly dynamic and heterogeneous vehicular communication networks,it is challeng-ing to efficiently utilize network resources and en-sure demanding performance requirements of safety-related applications.This paper investigates machine-learning-assisted transmission design in a typical multi-user vehicle-to-vehicle(V2V)communication scenario.The transmission process proceeds sequen-tially along the discrete time steps,where several source nodes intend to deliver multiple different types of messages to their respective destinations within the same spectrum.Due to rapid movement of vehicles,real-time acquirement of channel knowledge and cen-tral coordination of all transmission actions are in gen-eral hard to realize.We consider applying multi-agent deep reinforcement learning(MADRL)to handle this issue.By transforming the transmission design prob-lem into a stochastic game,a multi-agent proximal policy optimization(MAPPO)algorithm under a cen-tralized training and decentralized execution frame-work is proposed such that each source decides its own transmission message type,power level,and data rate,based on local observations of the environment and feedback,to maximize its energy efficiency.Via simulations we show that our method achieves better performance over conventional methods.
更多查看译文
关键词
V2V communication networks,sequential transmission decision-making,multi-agent deep reinforcement learning
AI 理解论文
溯源树
样例
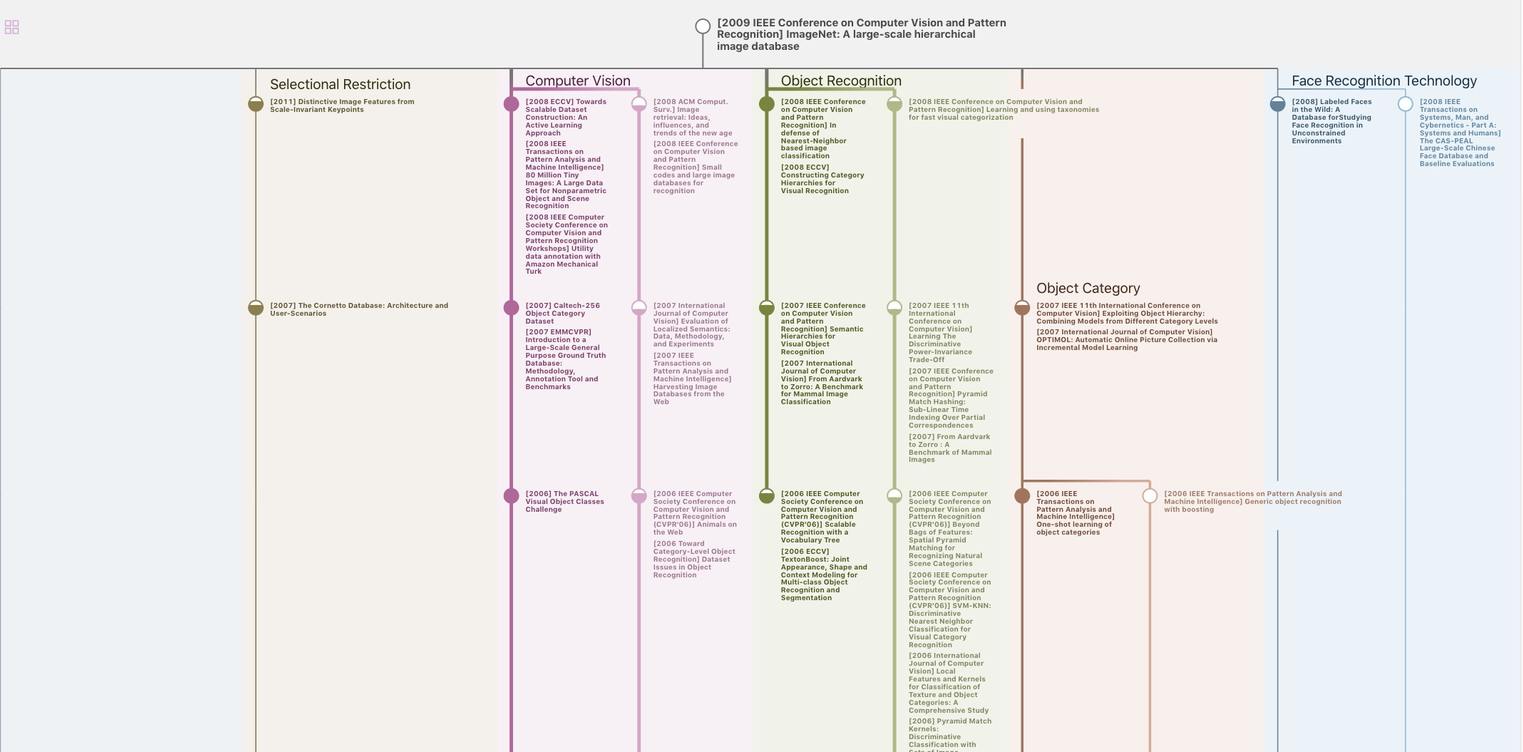
生成溯源树,研究论文发展脉络
Chat Paper
正在生成论文摘要