Preparation of Entangled Many-Body States with Machine Learning
arXiv (Cornell University)(2023)
摘要
Preparation of a target quantum many-body state on quantum simulators is one of the significant steps in quantum science and technology. With a small number of qubits, a few quantum states, such as the Greenberger-Horne-Zeilinger state, have been prepared, but fundamental difficulties in systems with many qubits remain, including the Lieb-Robinson bounds for the number of quantum operations. Here, we provide one algorithm with an implementation of a deep learning process and achieve to prepare the target ground states with many qubits. Our strategy is to train a machine-learning model and predict parameters with many qubits by utilizing a pattern of quantum states from the corresponding quantum states with small numbers of qubits. For example, we demonstrate that our algorithm with the Quantum Approximate Optimization Ansatz can effectively generate the ground state for a 1D XY model with 64 spins. We also demonstrate that the reduced density operator of two qubits can be utilized to capture the pattern of quantum many-body states such as correlation lengths even for quantum critical states.
更多查看译文
关键词
entangled,many-body
AI 理解论文
溯源树
样例
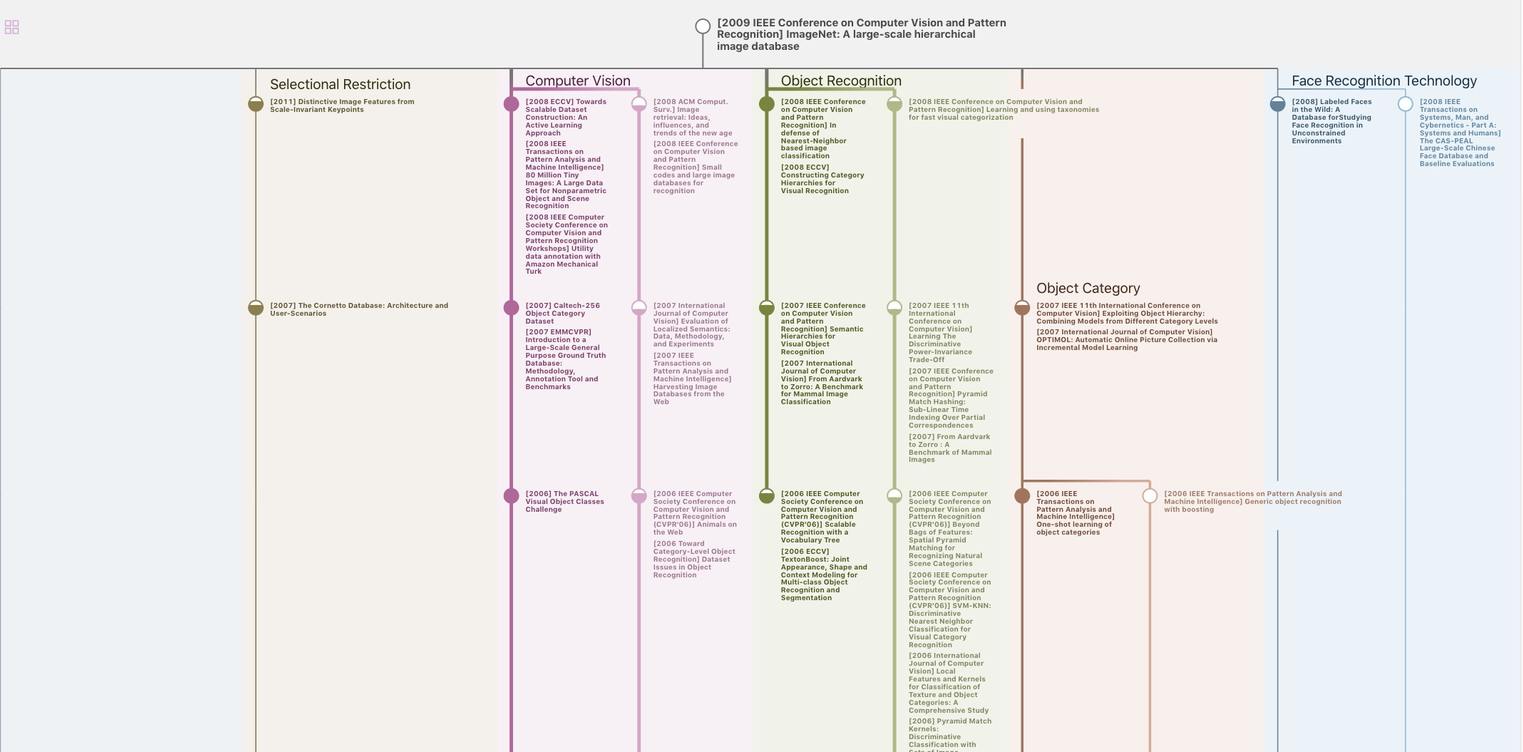
生成溯源树,研究论文发展脉络
Chat Paper
正在生成论文摘要