GaitMorph: Transforming Gait by Optimally Transporting Discrete Codes
2023 IEEE INTERNATIONAL JOINT CONFERENCE ON BIOMETRICS, IJCB(2023)
摘要
Gait, the manner of walking, has been proven to be a reliable biometric with uses in surveillance, marketing and security. A promising new direction for the field is training gait recognition systems without explicit human annotations, through self-supervised learning approaches. Such methods are heavily reliant on strong augmentations for the same walking sequence to induce more data variability and to simulate additional walking variations. Current data augmentation schemes are heuristic and cannot provide the necessary data variation as they are only able to provide simple temporal and spatial distortions. In this work, we propose GaitMorph, a novel method to modify the walking variation for an input gait sequence. Our method entails the training of a high-compression model for gait skeleton sequences that leverages unlabelled data to construct a discrete and interpretable latent space, which preserves identity-related features. Furthermore, we propose a method based on optimal transport theory to learn latent transport maps on the discrete codebook that morph gait sequences between variations. We perform extensive experiments and show that our method is suitable to synthesize additional views for an input sequence.
更多查看译文
关键词
Codebook,Data Augmentation,Biometric,Latent Space,Discrete Space,Optimal Transport,Self-supervised Learning,Spatial Distortion,Low-pass,Batch Normalization,Diffusion Model,Walking Speed,Action Recognition,Gait Analysis,Variational Autoencoder,Test Split,Graph Convolution,Morphing,Normal Walking,Cost Matrix,Dictionary Size,Fréchet Inception Distance,Earth Mover’s Distance,Pre-training Dataset,Latent Embedding,Vector Quantization,Changing Clothes,Sequence Of Tokens,Degree Of Compression,Latent Code
AI 理解论文
溯源树
样例
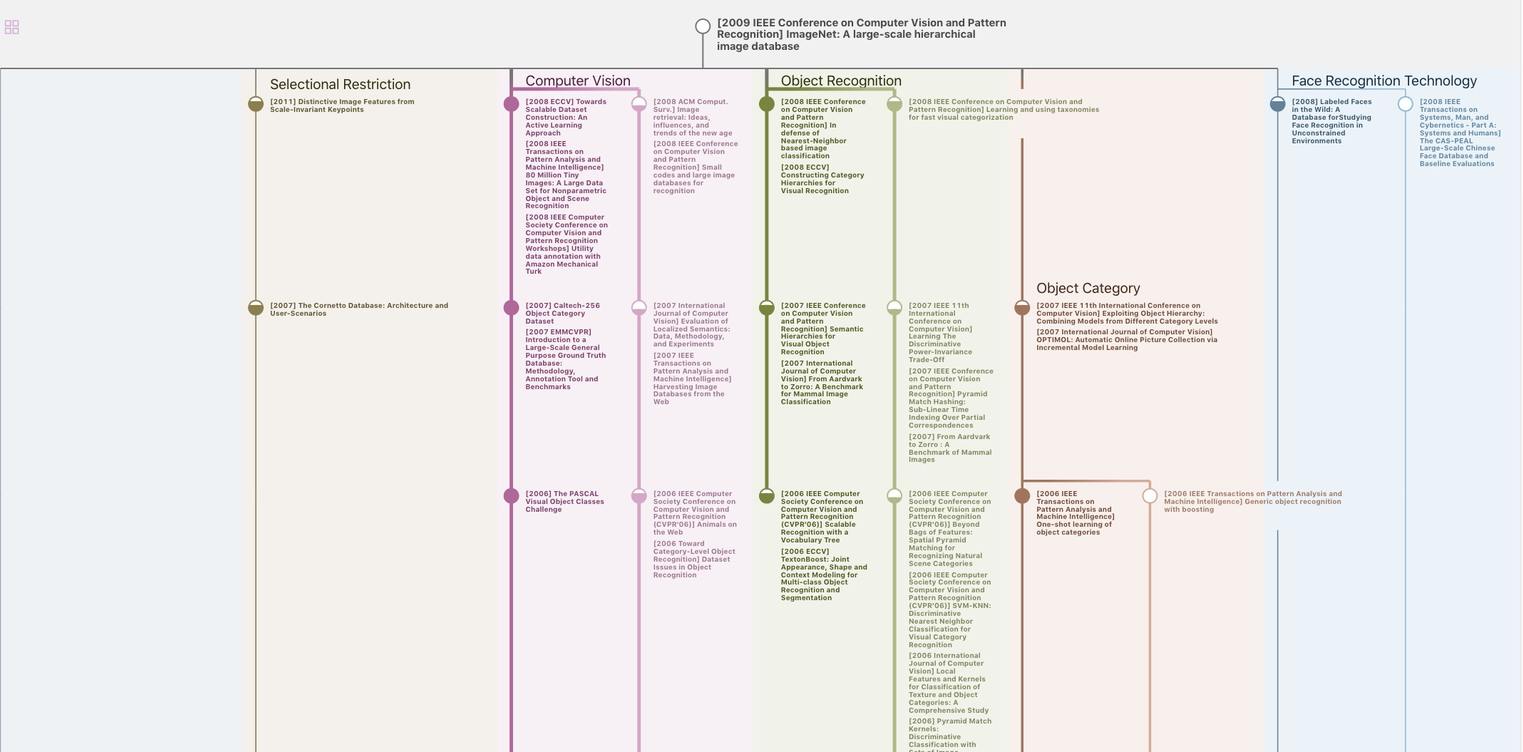
生成溯源树,研究论文发展脉络
Chat Paper
正在生成论文摘要