DNN-MG: A hybrid neural network/finite element method with applications to 3D simulations of the Navier-Stokes equations
CoRR(2024)
摘要
We extend and analyze the deep neural network multigrid solver (DNN-MG) for the Navier- Stokes equations in three dimensions. The idea of the method is to augment a finite element simulation on coarse grids with fine scale information obtained using deep neural networks. The neural network operates locally on small patches of grid elements. The local approach proves to be highly efficient, since the network can be kept (relatively) small and since it can be applied in parallel on all grid patches. However, the main advantage of the local approach is the inherent generalizability of the method. Since the network only processes data of small sub-areas, it never "sees"the global problem and thus does not learn false biases. We describe the method with a focus on the interplay between the finite element method and deep neural networks. Further, we demonstrate with numerical examples the excellent efficiency of the hybrid approach, which allows us to achieve very high accuracy with a coarse grid and thus reduce the computation time by orders of magnitude.
更多查看译文
关键词
Geometric multigrid methods,Deep neural network,Navier-Stokes equations,Machine learning
AI 理解论文
溯源树
样例
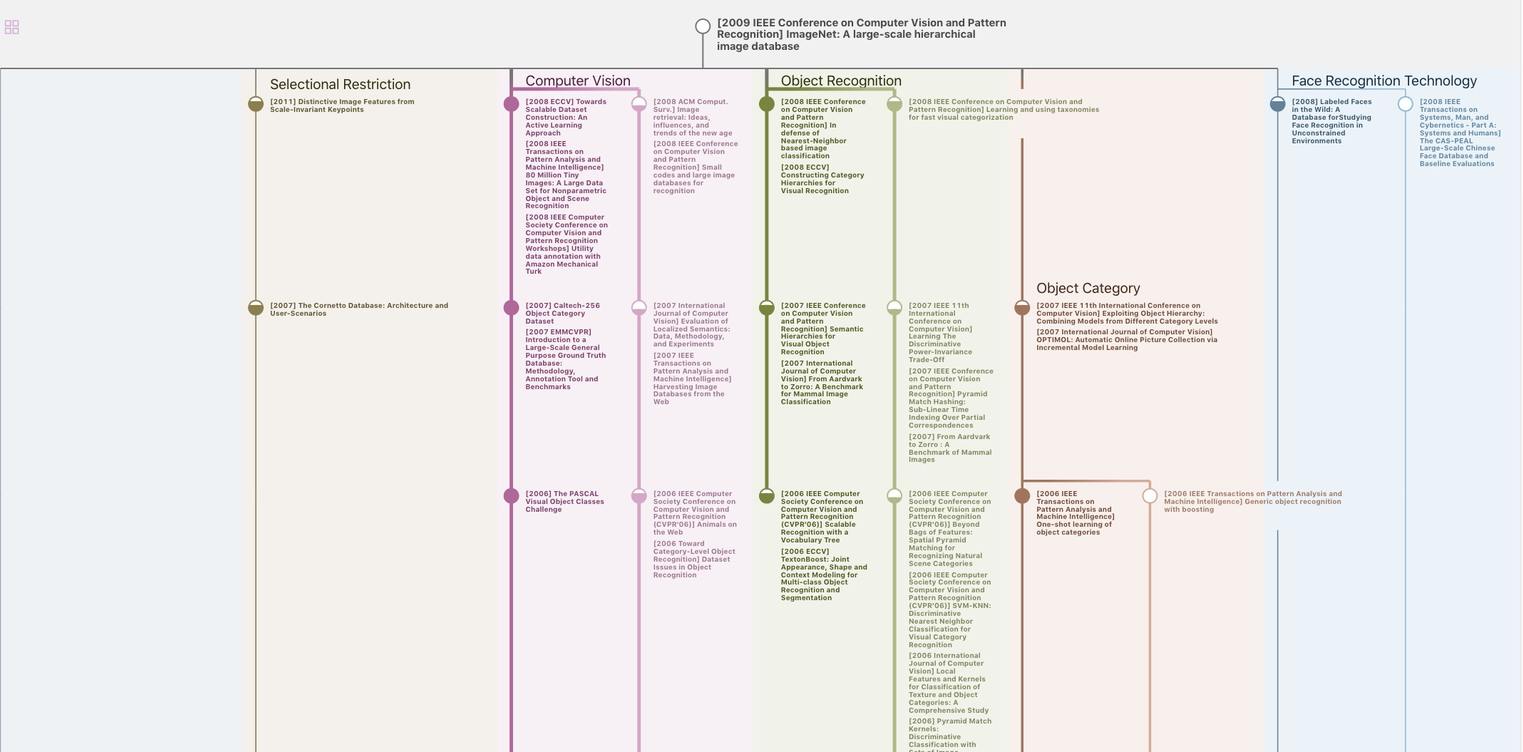
生成溯源树,研究论文发展脉络
Chat Paper
正在生成论文摘要