Lookahead data-gathering strategies for online adaptive model reduction of transport-dominated problems
CHAOS(2023)
摘要
Online adaptive model reduction efficiently reduces numerical models of transport-dominated problems by updating reduced spaces over time, which leads to nonlinear approximations on latent manifolds that can achieve a faster error decay than classical linear model reduction methods that keep reduced spaces fixed. Critical for online adaptive model reduction is coupling the full and reduced model to judiciously gather data from the full model for adapting the reduced spaces so that accurate approximations of the evolving full-model solution fields can be maintained. In this work, we introduce lookahead data-gathering strategies that predict the next state of the full model for adapting reduced spaces toward dynamics that are likely to be seen in the immediate future. Numerical experiments demonstrate that the proposed lookahead strategies lead to accurate reduced models even for problems where previously introduced data-gathering strategies that look back in time fail to provide predictive models. The proposed lookahead strategies also improve the robustness and stability of online adaptive reduced models.
更多查看译文
关键词
online adaptive model reduction,data-gathering,transport-dominated
AI 理解论文
溯源树
样例
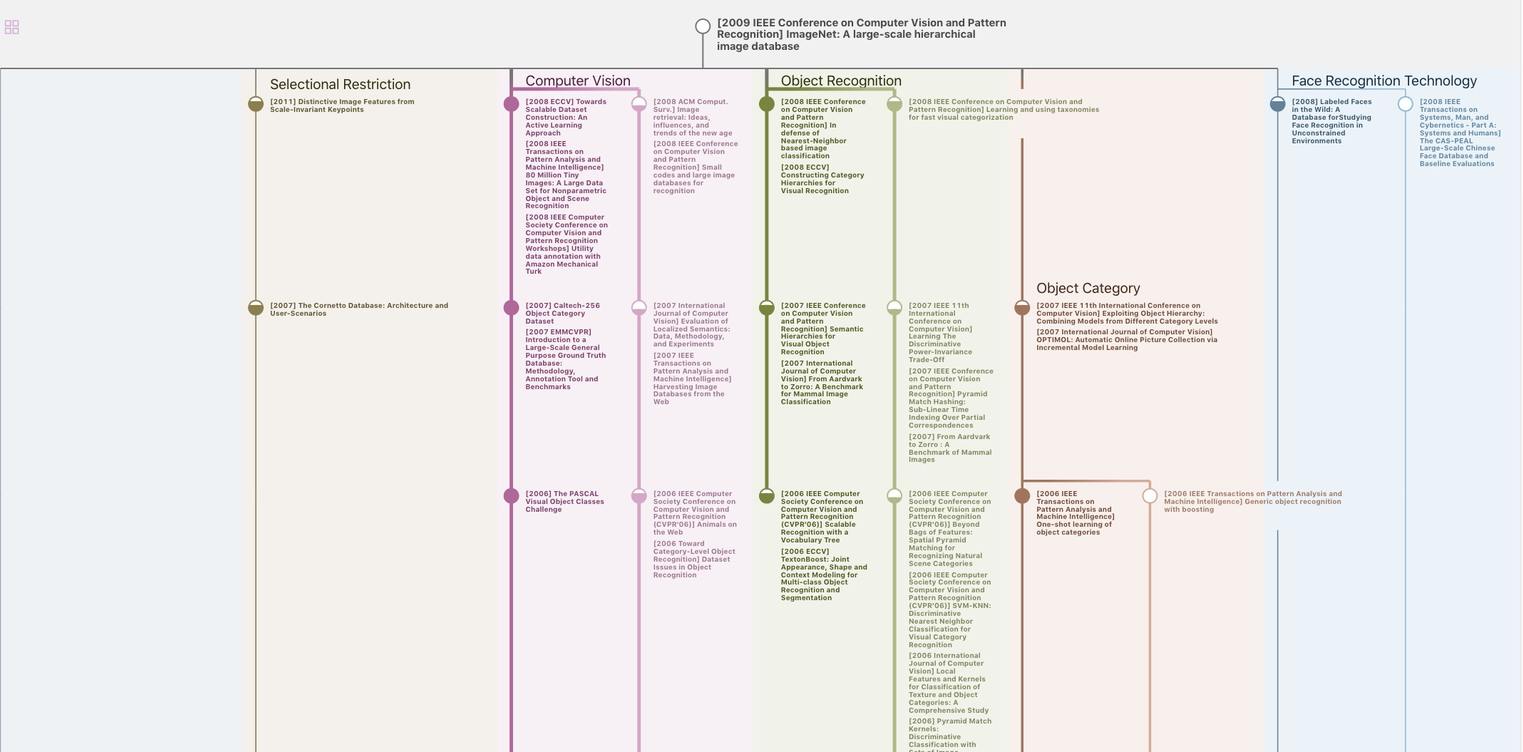
生成溯源树,研究论文发展脉络
Chat Paper
正在生成论文摘要