Autocalibrating Gaze Tracking: A Demonstration through Gaze Typing
CoRR(2023)
摘要
Miscalibration of gaze tracking devices and the resulting need for repeat calibration are a significant barrier to use. As devices miscalibrate, people tend to auto-correct by gazing at neighboring targets, which makes it difficult to detect miscalibration from eye signals. To address this problem, we provide a novel and simple insight for autocalibrating eye trackers during gaze typing: the eyes are used as both input (i.e. typing) and output (i.e. reading) signals, but auto-correction by users only occurs when eye gaze is functioning as input. Thus, output eye gaze signals during reading can help systems detect the miscalibration offset and enable autocalibration. To demonstrate the potential for this type of approach, we designed and built an auto-calibration system for gaze typing and ran a user study with 15 able-bodied participants. Results from our user study suggest that such an implicit approach to autocalibration can significantly improve typing speed and overall user experience for gaze typing interfaces. Insights from our work are applicable to a broad set of gaze tracking technologies and may help create more seamless user experiences in a variety of domains.
更多查看译文
AI 理解论文
溯源树
样例
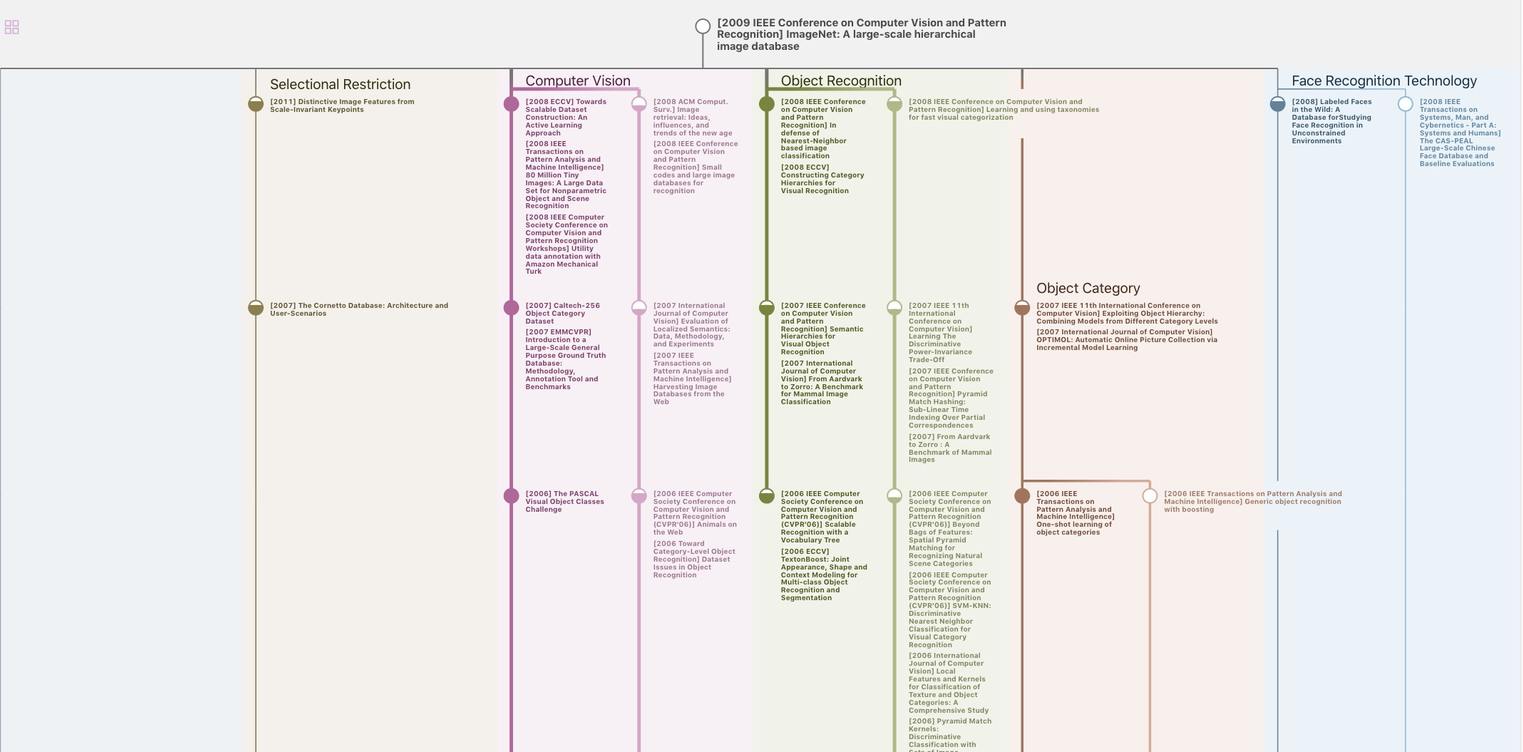
生成溯源树,研究论文发展脉络
Chat Paper
正在生成论文摘要