StainGAN: Learning a structural preserving translation for white blood cell images
JOURNAL OF BIOPHOTONICS(2023)
摘要
Analysis of white blood cells in blood smear images plays a vital role in computer-aided diagnosis for the analysis and treatment of many diseases. However, different techniques for blood smear preparation result in images with large appearance variations, which limits the performance of large-scale machine learning algorithms. In this paper, we propose StainGAN, an image translation framework to transform the conventional Wright-stained white blood cell images into their rapidly-stained counterpart. Moreover, we designed a cluster-based learning strategy that does not require manual annotations and a multi-scale discriminator that incorporates a richer hierarchy of the spatial context to generate sharper images with better semantic consistency. Experimental results on multiple real-world datasets prove the effectiveness of our proposed framework. Moreover, we show that the transformed images from StainGAN can be used to boost the downstream segmentation performance under the label-limiting scenario.
更多查看译文
关键词
white blood cell images
AI 理解论文
溯源树
样例
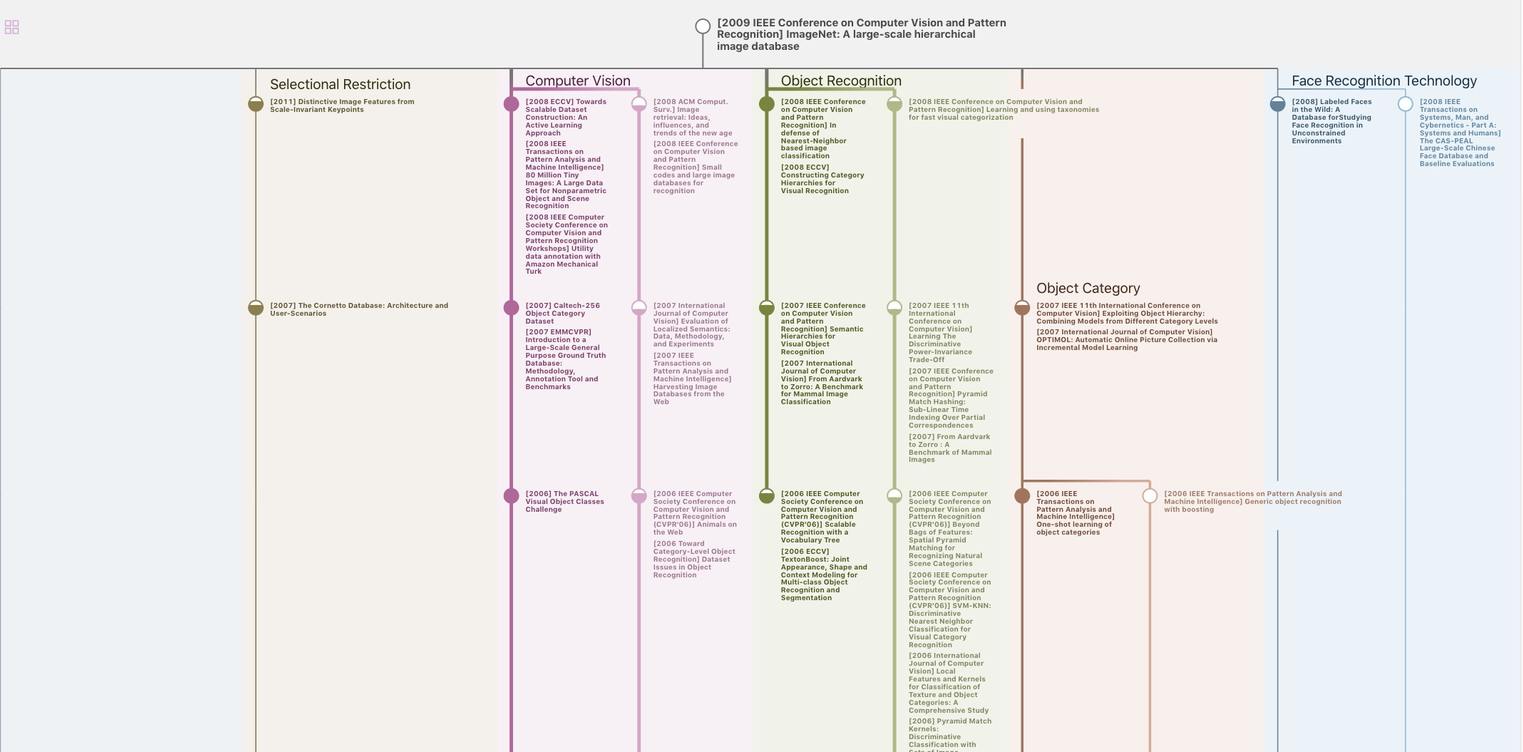
生成溯源树,研究论文发展脉络
Chat Paper
正在生成论文摘要