Ipadd: A Computational Tool for Predicting Potential Antidiabetic Drugs Using Machine Learning Algorithms.
JOURNAL OF CHEMICAL INFORMATION AND MODELING(2023)
摘要
Diabetes mellitus is a chronic metabolic disease, which causes an imbalance in blood glucose homeostasis and further leads to severe complications. With the increasing population of diabetes, there is an urgent need to develop drugs to treat diabetes. The development of artificial intelligence provides a powerful tool for accelerating the discovery of antidiabetic drugs. This work aims to establish a predictor called iPADD for discovering potential antidiabetic drugs. In the predictor, we used four kinds of molecular fingerprints and their combinations to encode the drugs and then adopted minimum-redundancy-maximum-relevance (mRMR) combined with an incremental feature selection strategy to screen optimal features. Based on the optimal feature subset, eight machine learning algorithms were applied to train models by using 5-fold cross-validation. The best model could produce an accuracy (Acc) of 0.983 with the area under the receiver operating characteristic curve (auROC) value of 0.989 on an independent test set. To further validate the performance of iPADD, we selected 65 natural products for case analysis, including 13 natural products in clinical trials as positive samples and 52 natural products as negative samples. Except for abscisic acid, our model can give correct prediction results. Molecular docking illustrated that quercetin and resveratrol stably bound with the diabetes target NR1I2. These results are consistent with the model prediction results of iPADD, indicating that the machine learning model has a strong generalization ability. The source code of iPADD is available at https://github.com/llllxw/iPADD.
更多查看译文
关键词
Drug Target Identification,Heart Disease Prediction
AI 理解论文
溯源树
样例
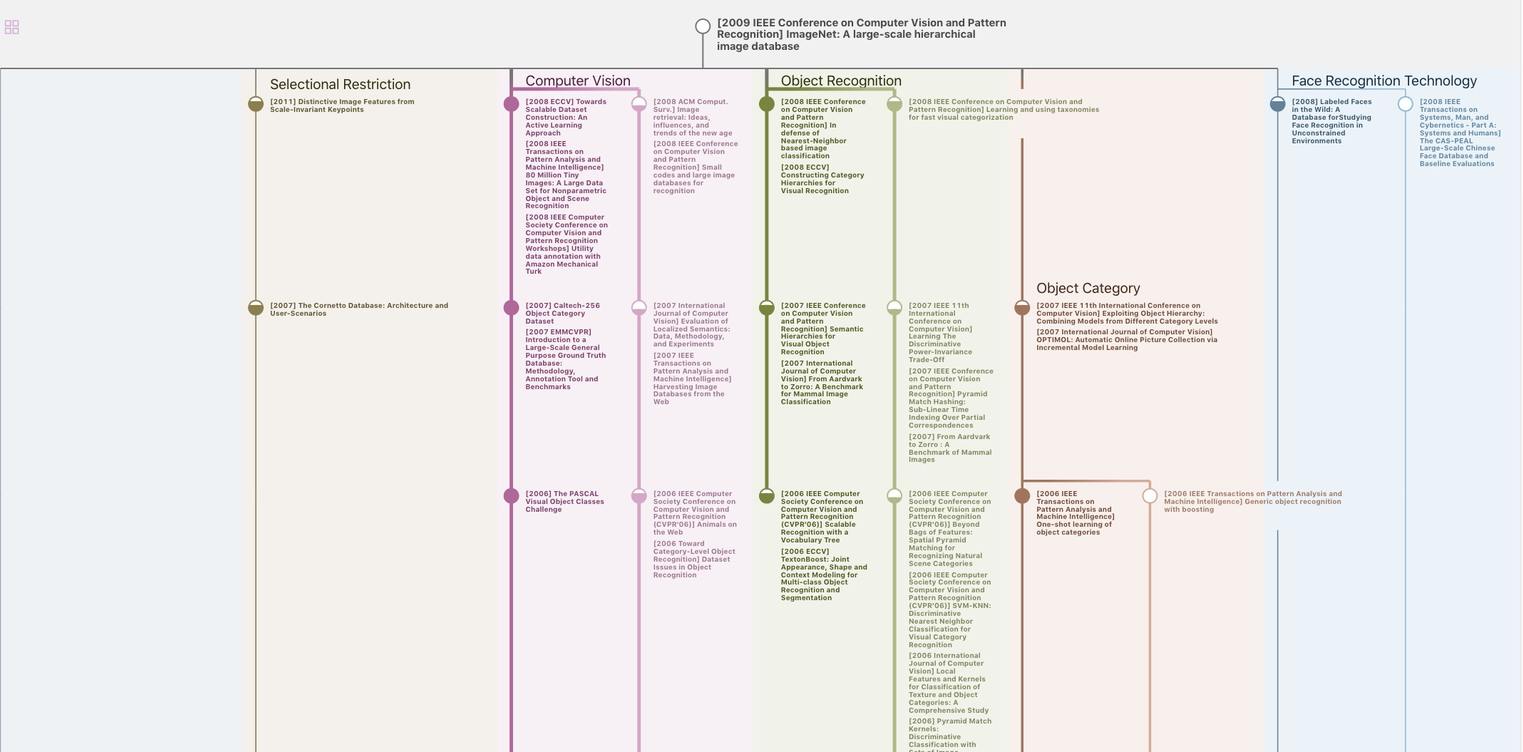
生成溯源树,研究论文发展脉络
Chat Paper
正在生成论文摘要