ESAMask: Real-Time Instance Segmentation Fused with Efficient Sparse Attention.
Sensors(2023)
摘要
Instance segmentation is a challenging task in computer vision, as it requires distinguishing objects and predicting dense areas. Currently, segmentation models based on complex designs and large parameters have achieved remarkable accuracy. However, from a practical standpoint, achieving a balance between accuracy and speed is even more desirable. To address this need, this paper presents ESAMask, a real-time segmentation model fused with efficient sparse attention, which adheres to the principles of lightweight design and efficiency. In this work, we propose several key contributions. Firstly, we introduce a dynamic and sparse Related Semantic Perceived Attention mechanism (RSPA) for adaptive perception of different semantic information of various targets during feature extraction. RSPA uses the adjacency matrix to search for regions with high semantic correlation of the same target, which reduces computational cost. Additionally, we design the GSInvSAM structure to reduce redundant calculations of spliced features while enhancing interaction between channels when merging feature layers of different scales. Lastly, we introduce the Mixed Receptive Field Context Perception Module (MRFCPM) in the prototype branch to enable targets of different scales to capture the feature representation of the corresponding area during mask generation. MRFCPM fuses information from three branches of global content awareness, large kernel region awareness, and convolutional channel attention to explicitly model features at different scales. Through extensive experimental evaluation, ESAMask achieves a mask AP of 45.4 at a frame rate of 45.2 FPS on the COCO dataset, surpassing current instance segmentation methods in terms of the accuracy-speed trade-off, as demonstrated by our comprehensive experimental results. In addition, the high-quality segmentation results of our proposed method for objects of various classes and scales can be intuitively observed from the visualized segmentation outputs.
更多查看译文
关键词
attention,real-time
AI 理解论文
溯源树
样例
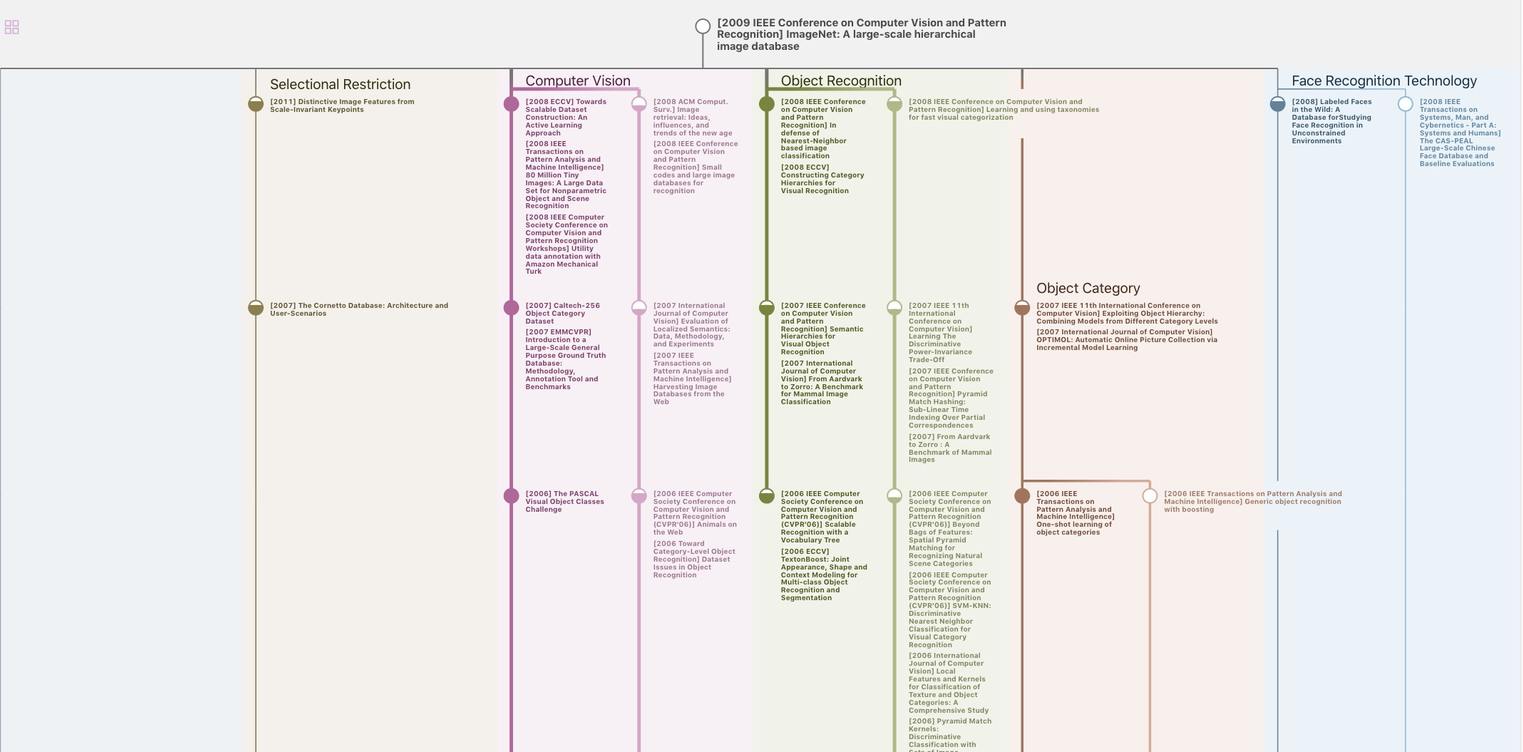
生成溯源树,研究论文发展脉络
Chat Paper
正在生成论文摘要