Semi-supervised seismic attenuation compensation method based on GRU dominated deep neural network
Chinese Journal of Geophysics(2023)
摘要
Seismic wave propagation in the subsurface is characterized by energy attenuation and nonstationarity, which reduces the seismic resolution and is not conducive to the fine description of reservoir characteristics. To solve this problem, it is necessary to compensate for the seismic attenuation to eliminate nonstationarity. Considering the advantages of GRU (Gated Recurrent Unit) in long-term and short-term memory when processing time sequence data, this paper constructs a neural network structure composed mainly of GUR (abbreviated as GRU neural network) and proposes a deep learning seismic attenuation compensation method based on GRU neural network. Log data is often very limited in the field seismic data processing, resulting in less labeled data. To solve the small sample size problem, inspired by autoencoder, we use synthetic seismic records and log data as labeled data and the remaining seismic records as unlabeled data for training to learn the nonlinear mapping from nonstationary data to stationary data in a semi-supervised manner to compensate for the seismic attenuation. Finally, the proposed method is tested by using noisy synthetic seismic data and field seismic data and its effectiveness is proved.
更多查看译文
关键词
Seismic attenuation compensation,Deep learning,Semi-supervised learning
AI 理解论文
溯源树
样例
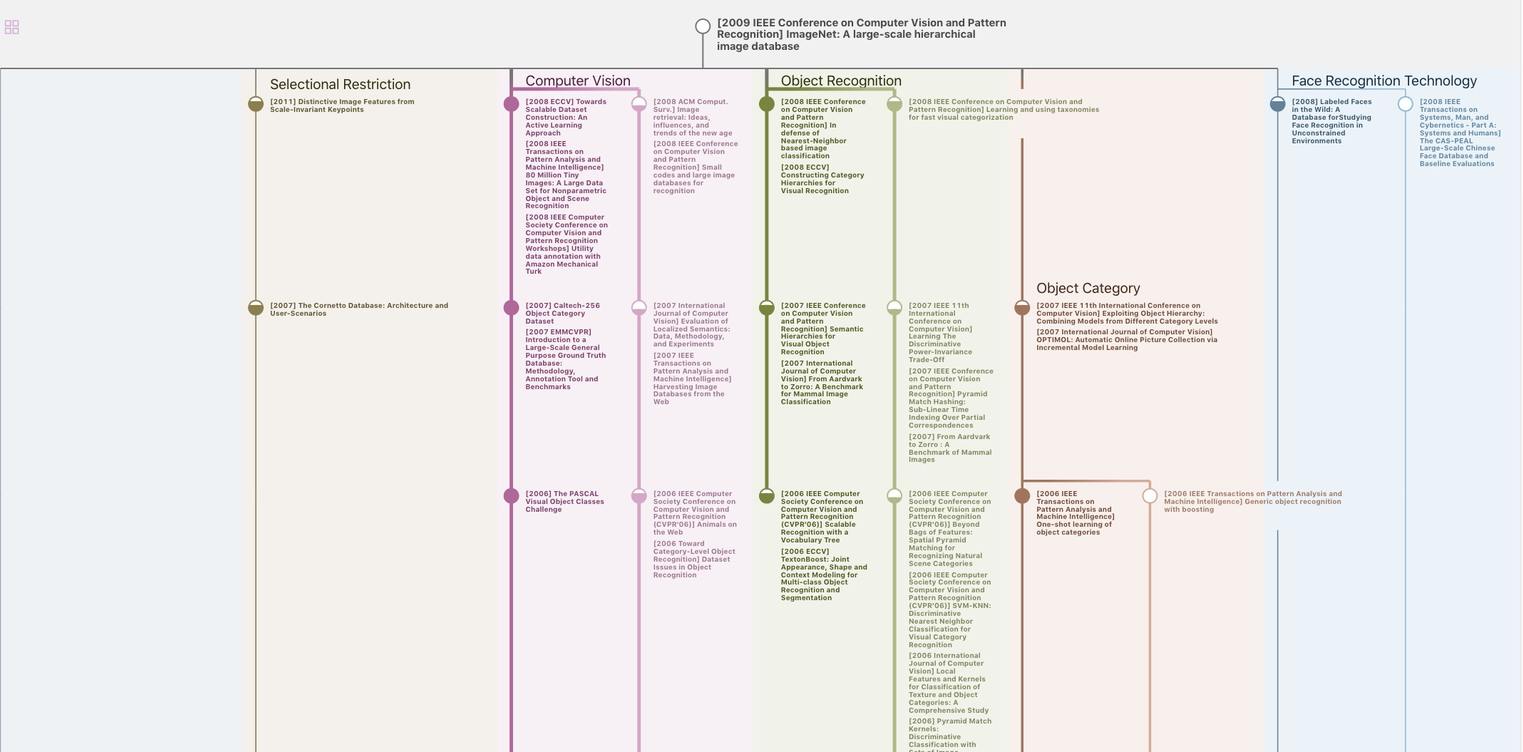
生成溯源树,研究论文发展脉络
Chat Paper
正在生成论文摘要