Triple Alliance Prototype Orthotist Network for Long-Tailed Multi-Label Text Classification.
IEEE ACM Trans. Audio Speech Lang. Process.(2023)
摘要
Multi-label text classification (MLTC) aims to tag the most relevant labels for the given document. Compared to the standard multi-class case where each document has only one label, it is considerably more difficulty to annotate new coming documents for multi-label text classification. Furthermore, it also suffers from the challenge of highly skewed long-tailed label distribution. Due to the relative infrequency of tail labels, this leads to an imbalance that biases towards predicting more head labels. To address the challenge, we propose a Triple Alliance Prototype Orthotist Network (TAPON) to build a generic meta-mapping from few-shot prototypes to many-shot classifier parameters, which aims to promote the generalizability of tail classifiers. To be specific, TAPON is a two-stage method. At the first stage, TAPON obtains the meta-knowledge between many-shot classifier parameters and few-shot prototype of head labels. Meanwhile, the triple alliance prototype is obtained by adopting an Attentive Prototype with the aid of few-shot documents, label semantic information and label correlation. Additionally, a Prototype Orthotist module is especially designed to capture the meta-knowledge between the many-shot classifier and few-shot prototype. At the second stage of transferring, TAPON aims to transfer the generic meta-mapping from head labels to tail labels. It first uses Attentive Prototype to obtain triple alliance prototype for tail labels, and then uses the meta-knowledge obtained from the first stage to get many-shot classifiers for tail labels. By conducting extensive experiments on benchmark datasets, we show that the proposed TAPON significantly outperforms other state-of-the-art methods for long-tailed multi-label text classification.
更多查看译文
关键词
classification,text,network,long-tailed,multi-label
AI 理解论文
溯源树
样例
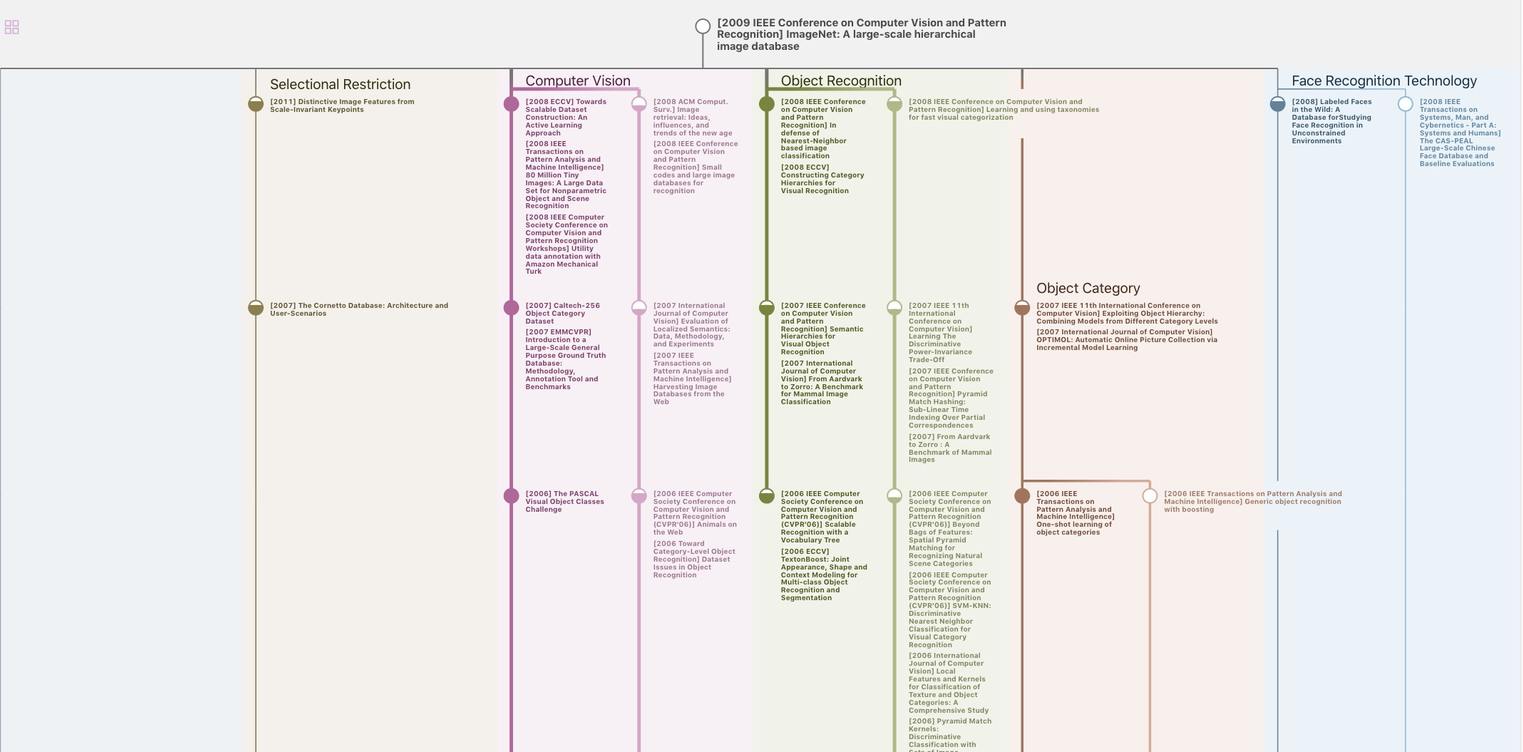
生成溯源树,研究论文发展脉络
Chat Paper
正在生成论文摘要