SG-Det: Shuffle-GhostNet-Based Detector for Real-Time Maritime Object Detection in UAV Images.
Remote. Sens.(2023)
摘要
Maritime search and rescue is a crucial component of the national emergency response system, which mainly relies on unmanned aerial vehicles (UAVs) to detect objects. Most traditional object detection methods focus on boosting the detection accuracy while neglecting the detection speed of the heavy model. However, improving the detection speed is essential, which can provide timely maritime search and rescue. To address the issues, we propose a lightweight object detector named Shuffle-GhostNet-based detector (SG-Det). First, we construct a lightweight backbone named Shuffle-GhostNet, which enhances the information flow between channel groups by redesigning the correlation group convolution and introducing the channel shuffle operation. Second, we propose an improved feature pyramid model, namely BiFPN-tiny, which has a lighter structure capable of reinforcing small object features. Furthermore, we incorporate the Atrous Spatial Pyramid Pooling module (ASPP) into the network, which employs atrous convolution with different sampling rates to obtain multi-scale information. Finally, we generate three sets of bounding boxes at different scales-large, medium, and small-to detect objects of different sizes. Compared with other lightweight detectors, SG-Det achieves better tradeoffs across performance metrics and enables real-time detection with an accuracy rate of over 90% for maritime objects, showing that it can better meet the actual requirements of maritime search and rescue.
更多查看译文
关键词
object detection, UAV images, lightweight network, maritime search and rescue
AI 理解论文
溯源树
样例
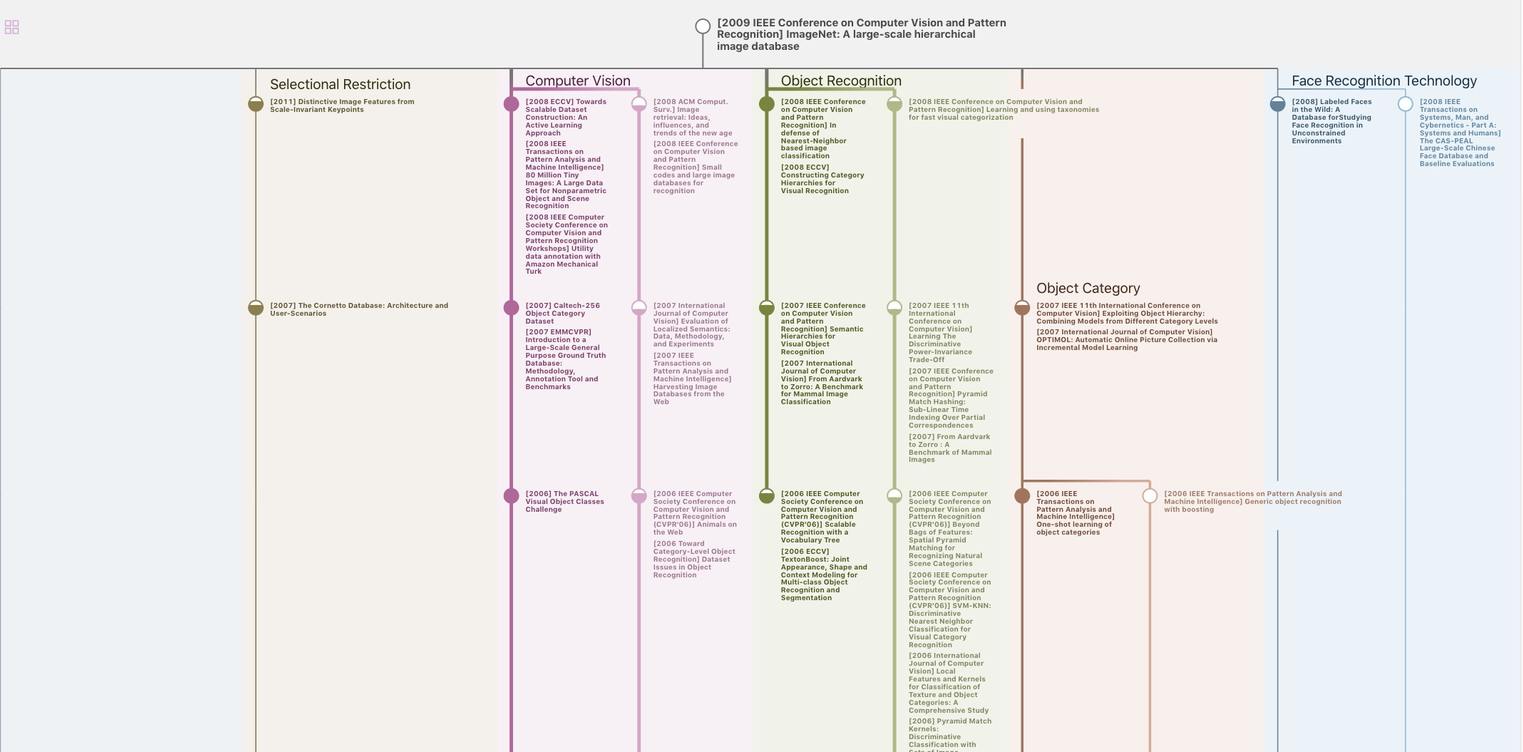
生成溯源树,研究论文发展脉络
Chat Paper
正在生成论文摘要