A Federated Framework for Edge Computing Devices with Collaborative Fairness and Adversarial Robustness
J. Grid Comput.(2023)
摘要
Federated learning is a distributed machine learning framework for edge computing devices that provides several benefits, such as eliminating over-fitting and protecting privacy. However, the majority of federated learning paradigms have not taken fairness into account. Since the quality and quantity of the data held by each participant varies, their contributions are always diverse. In other words, the fact that all devices receive the same model as a reward, regardless of their various contributions, is unfair to those who contribute the most. In this work, we provide s-CFFL, a federated framework for edge computing devices that ingeniously combines the reputation mechanism with distributed selective stochastic gradient descent (DSSGD) to achieve collaborative fairness. In addition, we investigate the resistance of the framework against free-riders and several other common adversaries. We perform comprehensive trials comparing our framework to FedAvg, DSSGD, and other related approaches. The results indicate that our strategy strikes a compromise between models’ prediction accuracy and collaborative fairness while simultaneously boosting model robustness.
更多查看译文
关键词
Federated learning, Fair, Robust, DSSGD, Reputation
AI 理解论文
溯源树
样例
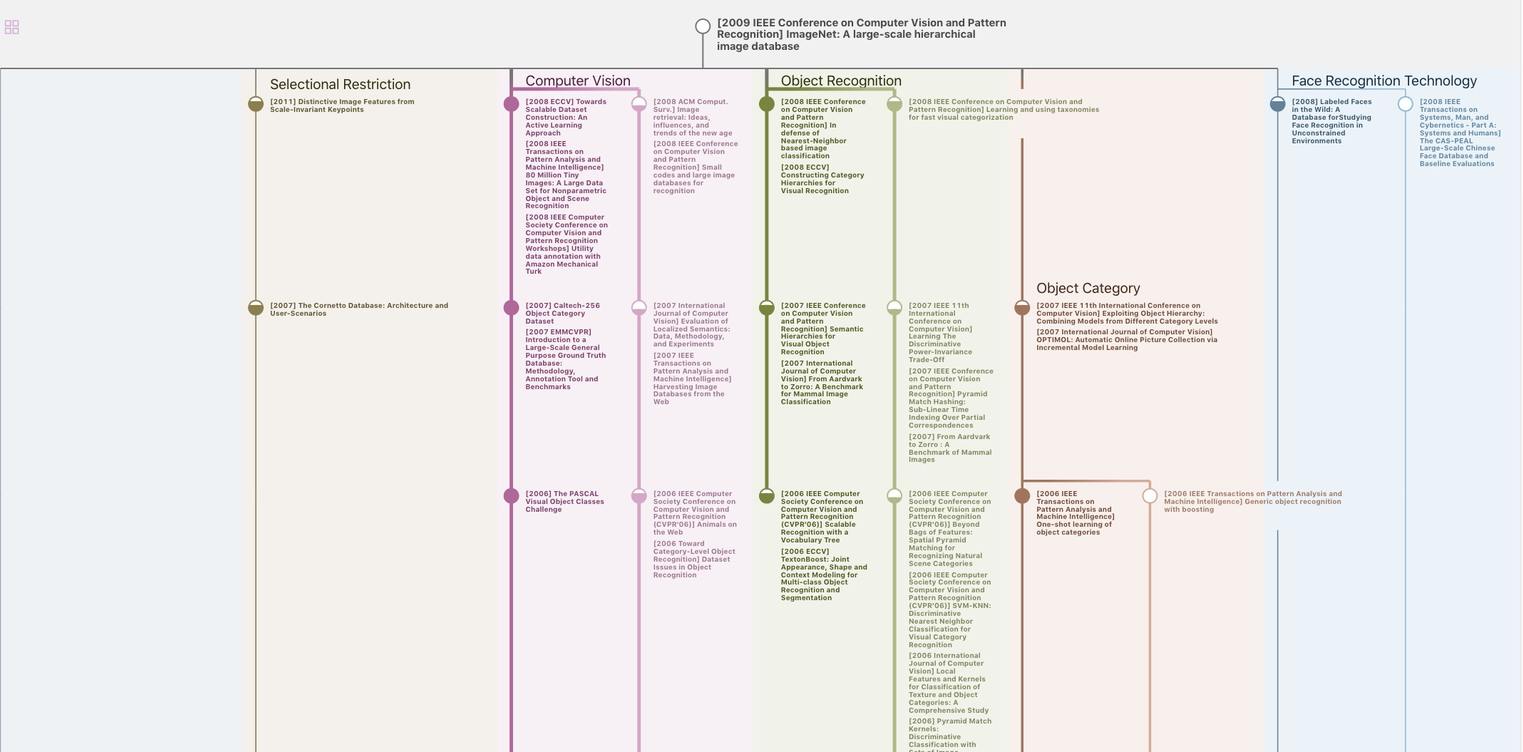
生成溯源树,研究论文发展脉络
Chat Paper
正在生成论文摘要