Simulation of Complex Geological Architectures Based on Multistage Generative Adversarial Networks Integrating With Attention Mechanism and Spectral Normalization.
IEEE Trans. Geosci. Remote. Sens.(2023)
摘要
The geostatistics stimulation method, as an important tool in subsurface modeling, is crucial for hydrocarbon reservoir characterization. Using geostatistical methods to reproduce complex heterogeneous structures is still challenging because of nonstationarity and computational consumption. We develop a stabilized stochastic simulation method by introducing the generative adversarial network based on a single image (SinGAN). It can preserve multiscale features contained in an individual training image by using a multistage training framework. In order to stabilize the training of the discriminator, the spectral normalization is integrated. We also introduce spatial attention and channel attention mechanism into the network to focus on the most significant features in each training stage, so that these features can be reproduced in the realizations. An adaptive strategy is adopted to automatically choose training stages, which balances the diversity and quality of simulation results and decreases the man-made factor on SinGAN. Several experiments are tested on synthetic and actual training images, respectively. We evaluate the simulation results from many perspectives, including variability, connectivity, probability density distribution, and time-consuming. The successful application of the new method on both categorical and continuous variables indicates that it has a strong ability to reproduce complex subsurface models, even for nonstationary geologic phenomena.
更多查看译文
关键词
complex geological architectures,networks,simulation,attention mechanism,multi-stage
AI 理解论文
溯源树
样例
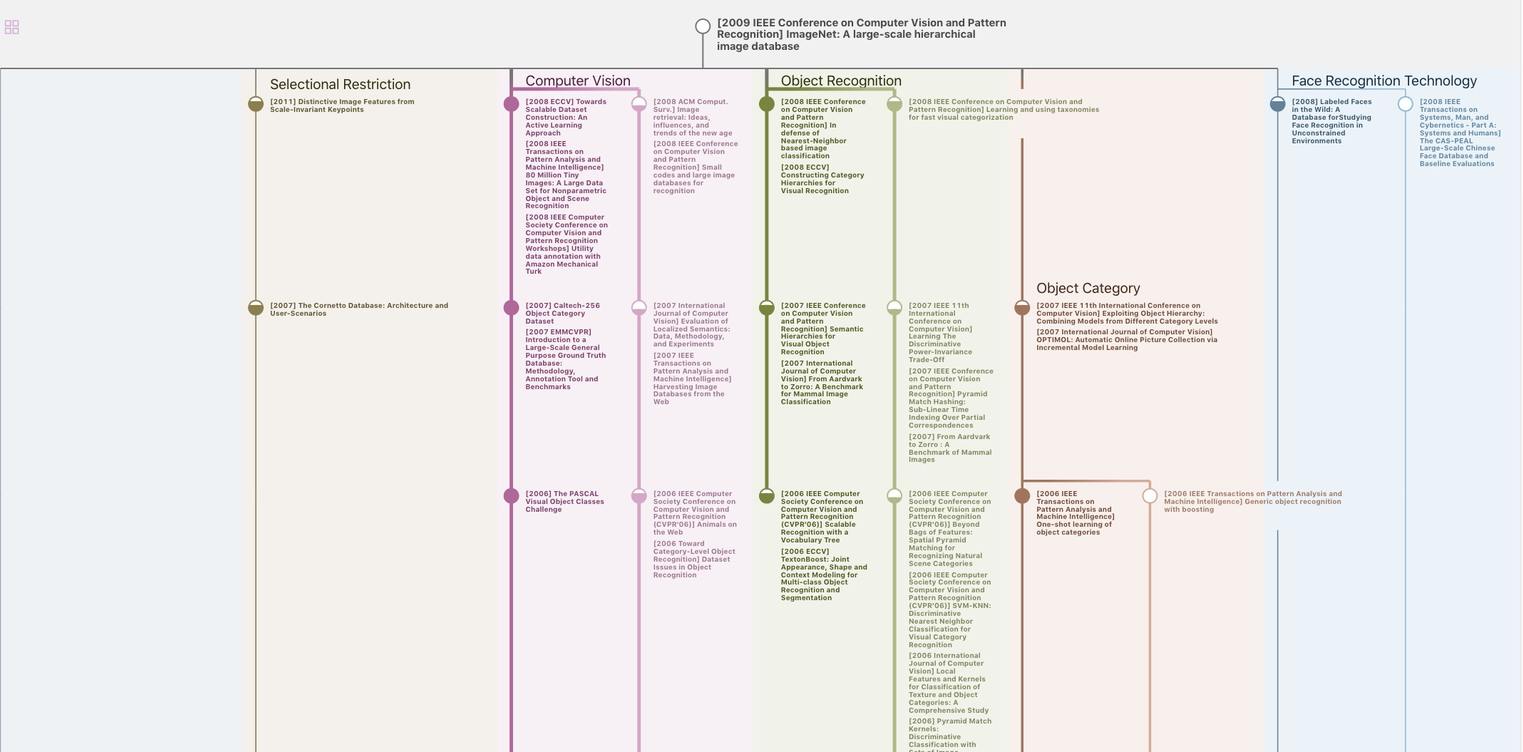
生成溯源树,研究论文发展脉络
Chat Paper
正在生成论文摘要