A New GNSS TEC Neural Network Prediction Algorithm With the Data Fusion of Physical Observation.
IEEE Trans. Geosci. Remote. Sens.(2023)
摘要
This work models and predicts anomalous jumps in global navigation satellite system (GNSS) total electron content (TEC) caused by geomagnetic disturbance or solar radiation by introducing F10.7 and regional Dst indices, which are used to characterize solar radiation and geomagnetic disturbance status, respectively. We then introduced long-distance constraint stations to restrain ionospheric variations during high solar activity, which reduced the influence of unmodeled factors on prediction accuracy. In our experimental work, global ionosphere map (GIM) TEC data from 11 stations located at different latitudes were used for comparison during maximum (2014) and minimum (2018) of solar cycle 24. Sample data for >27 days were used for learning, and then, ionospheric changes for the whole year were predicted using the sliding window method. The results showed that the standard deviation for the global ionospheric prediction products could be guaranteed to be within 2 TECU. The proximity station constraint method proposed in this article and the Dst high-order polynomial correction method during geomagnetic disturbance control the prediction error within -4.4 TECU, while the change of adjacent days is -51.4 TECU in 2014. The new algorithm reduced the TEC jump effect in predictions, and the prediction of ionospheric products provided by this algorithm can meet the basic requirements of ionospheric delay correction in high-precision positioning.
更多查看译文
关键词
Data fusion, Dst, F107, global navigation satel-lite system (GNSS) total electron content (TEC), neural network
AI 理解论文
溯源树
样例
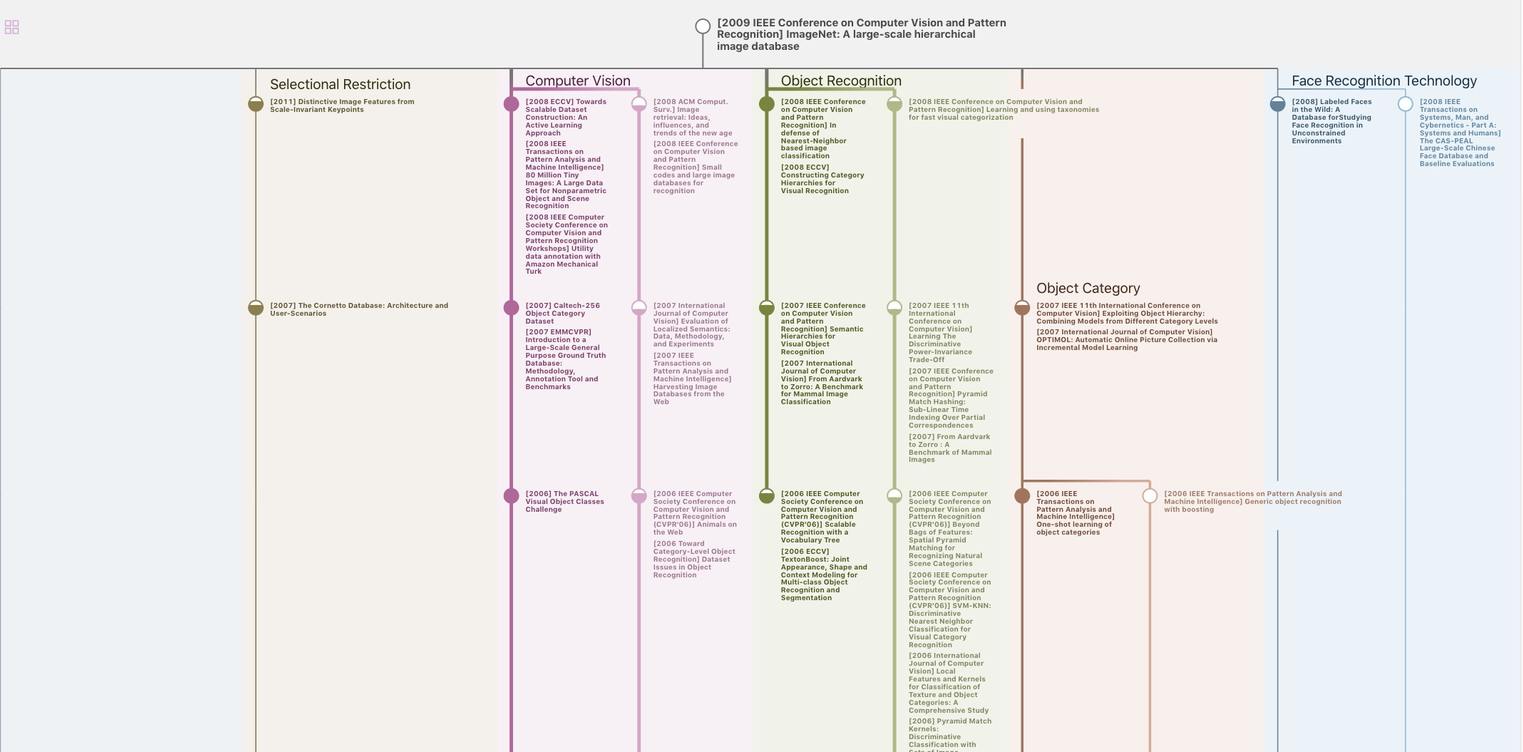
生成溯源树,研究论文发展脉络
Chat Paper
正在生成论文摘要