FTMF-Net: A Fourier Transform-Multiscale Feature Fusion Network for Segmentation of Small Polyp Objects.
IEEE Trans. Instrum. Meas.(2023)
摘要
The detection and resection of small polyp objects in colonoscopy images are of great significance for the prevention of colorectal cancer (CRC). At present, blurred edges, variable lesion shapes, and intraclass dissimilarity pose challenges for accurately segmenting small polyp objects. In recent years, many deep learning methods based on convolutional neural networks (CNNs) have been proposed and successfully applied to polyp segmentation tasks. However, these methods still have three limitations: 1) limited ability to mine boundary detail information; 2) insufficient ability to capture rich global context information; and 3) introduced additional complex feature extraction operations. To alleviate these challenges, we propose a Fourier transform-multiscale feature fusion network (FTMF-Net) for segmentation of small polyp objects. The core idea includes two points: 1) Fourier transform (FT) module extracts more detailed boundary information and 2) multiscale feature fusion (MFF) module enriches global semantic feature information. FTMF-Net mainly has the following advantages: 1) the proposed model has excellent performance for small polyp object segmentation; 2) this method greatly reduces the complexity of the model without significantly increasing the number of network parameters; and 3) the network is relatively simple and easy to understand. Extensive experiments with 11 state-of-the-art (SOTA) methods on five small polyp object datasets show that our proposed FTMF-Net has superior segmentation performance.
更多查看译文
关键词
Dilated convolution, Fourier transform (FT), multiscale feature fusion (MFF), small polyp object segmentation
AI 理解论文
溯源树
样例
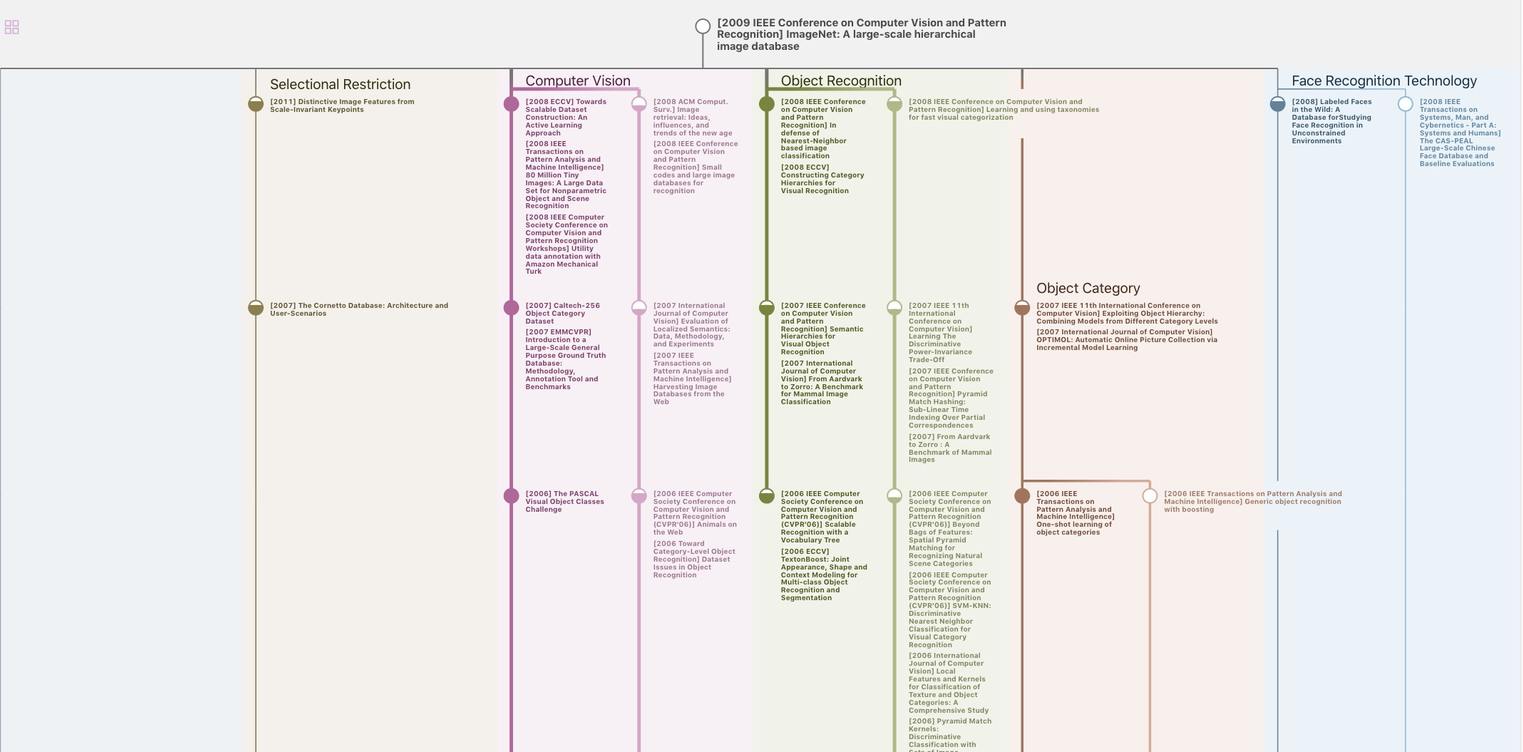
生成溯源树,研究论文发展脉络
Chat Paper
正在生成论文摘要