A Flexible Monitoring Framework via Dynamic-Multilayer Graph Convolution Network
IEEE Trans. Instrum. Meas.(2023)
摘要
Due to the continuous technological innovation in industrial processes, many deep learning-based methods have shown powerful capability in handling equipment status monitoring, but most of them ignore the temporal features and the dynamic changes of the diverse spatial structure of the raw data. Meanwhile, these methods usually focus on handling a single downstream task but rarely consider different tasks simultaneously. To solve these issues, this article proposes a more flexible monitoring framework based on a dynamic-multilayer graph convolution network (GCN), which can be adapted to different downstream tasks simultaneously by agile combinations of the modules according to the different industrial scenarios. First, the time series of fault samples are segmented and constructed into a feature matrix to extract the temporal information by the temporal module. At the same time, to fully characterize the dynamic change of different spatial structures among samples, the dynamic graph of each moment is expanded into a multilayer graph through various composition indexes. Then, the spatial information is extracted by the intralayer and interlayer convolution operations in the spatial module, and the fused features are applied to different downstream tasks. Finally, the experiments are performed in two different downstream tasks, namely, three datasets for fault diagnosis and one dataset for remaining useful life (RUL) prediction. The results of both diagnosis and prediction are better than those of the comparison algorithms, so the effectiveness and flexibility of the proposed framework are proven.
更多查看译文
关键词
~Dynamic-multilayer graph, fault diagnosis, graph convolution network (GCN), remaining useful life (RUL) prediction. the tical
AI 理解论文
溯源树
样例
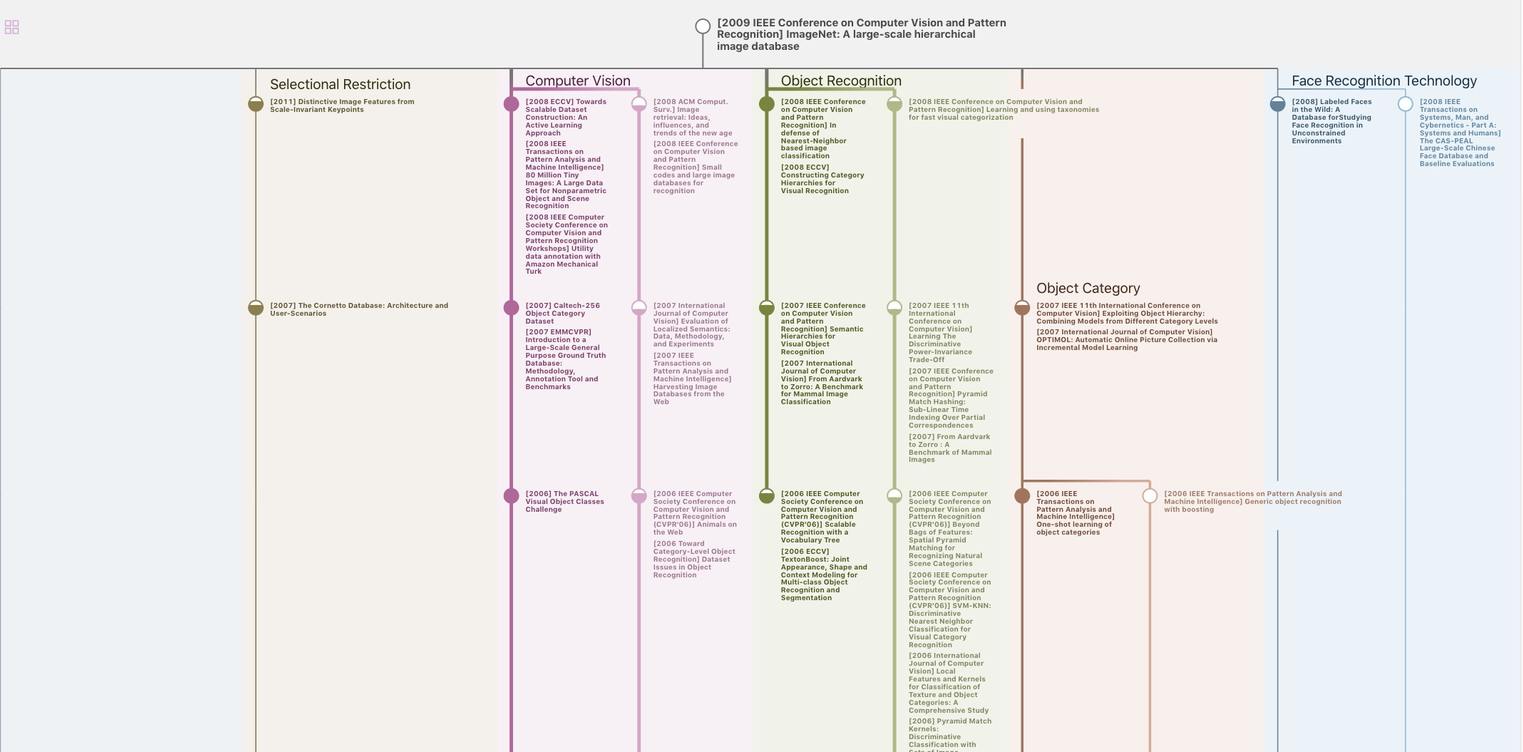
生成溯源树,研究论文发展脉络
Chat Paper
正在生成论文摘要