A GPS Distance Error Forecast Model Based on IIR Filter Denoising and LSTM.
IEEE Trans. Instrum. Meas.(2023)
摘要
Wireless channel prediction has attracted significant attention in recent years but is still considered an open topic. Global positioning system (GPS) error prediction is one such example. Conventional mathematical and statistical models cannot predict time-varying channel variables within coherent time windows. Their mechanisms are geared toward minimizing mean square errors over all datasets rather than time-sequential prediction within coherent windows. On the other hand, artificial neural network (ANN)-based approaches have been introduced and studied for this purpose. ANNs have the capability to learn and identify underlying patterns in data. However, extracting and predicting wireless channel variables are a nontrivial task that presents challenges to researchers. Moreover, the widely applied long short-term memory (LSTM) does not demonstrate satisfactory results because of unavoidable interferences, outliers, and noise. To address these concerns, a novel LSTM with infinite impulse response (IIR) filtering is proposed, which performs preprocessing of data smoothing. The proposed model reconfigures LSTM cells by adding new IIR gates, dedicated to removing noise and interferences for each cell in the hidden layers of ANN, and is self-adaptive through backpropagation during the training phase to achieve optimization. The model is evaluated with several commonly used time-series forecast models, and the GPS error prediction results demonstrate that the proposed model is able to well adapt to the datasets with the best performance. The average prediction accuracy at the 10th step is increased by 34% compared to the second-best model.
更多查看译文
关键词
Accuracy, backpropagation, global position systems error, infinite impulse response (IIR), long short-term memory (LSTM), neural network (NN), noise, self-adaptive, time-series prediction, wireless channel
AI 理解论文
溯源树
样例
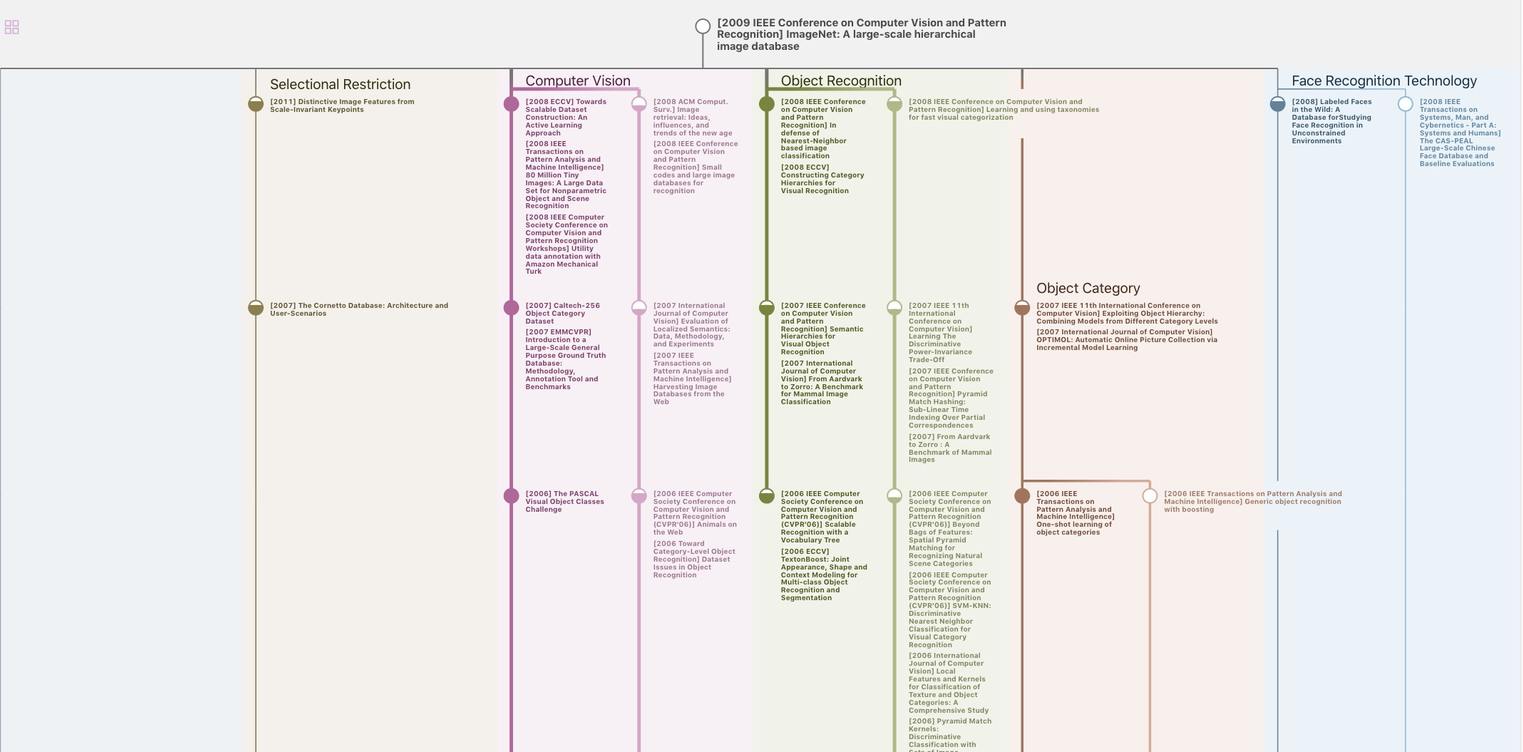
生成溯源树,研究论文发展脉络
Chat Paper
正在生成论文摘要