A Novel Competitive Temporal Convolutional Network for Remaining Useful Life Prediction of Rolling Bearings.
IEEE Transactions on Instrumentation and Measurement(2023)
摘要
Deep learning has been wildly utilized in the remaining useful life (RUL) prediction of rolling bearings. Extracting valuable features effectively is a challenging task in this field. In this article, we propose a novel competitive temporal convolutional network (CTCN) to predict the RUL of rolling bearings. First, a novel dual competitive attention (DCA) is proposed to enhance the feature extraction of the deep learning model. In DCA, a unique global competition (GC) module is designed to gather the global large-pooled features as the benchmark of attention calculation, and a new multidimensional competition (MC) module is proposed to fuse the attention in two dimensions. Then, a competitive temporal convolutional block (CTCB) based on the DCA and temporal convolutional network (TCN) is designed to extract high-level features from the input sequences. Finally, a CTCN is constructed to map the original vibration signals to the RUL. The experimental results illustrate that the proposed CTCN is effective, and DCA can significantly improve the accuracy of RUL prediction. The ablation experiments show that the proposed GC and MC can improve the performance of the proposed attention. They reduce the root mean square error (RMSE) on average by 0.0095/8.76% and 0.0031/2.95% and the mean absolute error (MAE) on average by 0.0074/8.46% and 0.0033/3.82%.
更多查看译文
关键词
Competitive temporal convolutional network (CTCN),dual competitive attention (DCA),global large-pooled feature,remaining useful life (RUL) prediction,rolling bearing
AI 理解论文
溯源树
样例
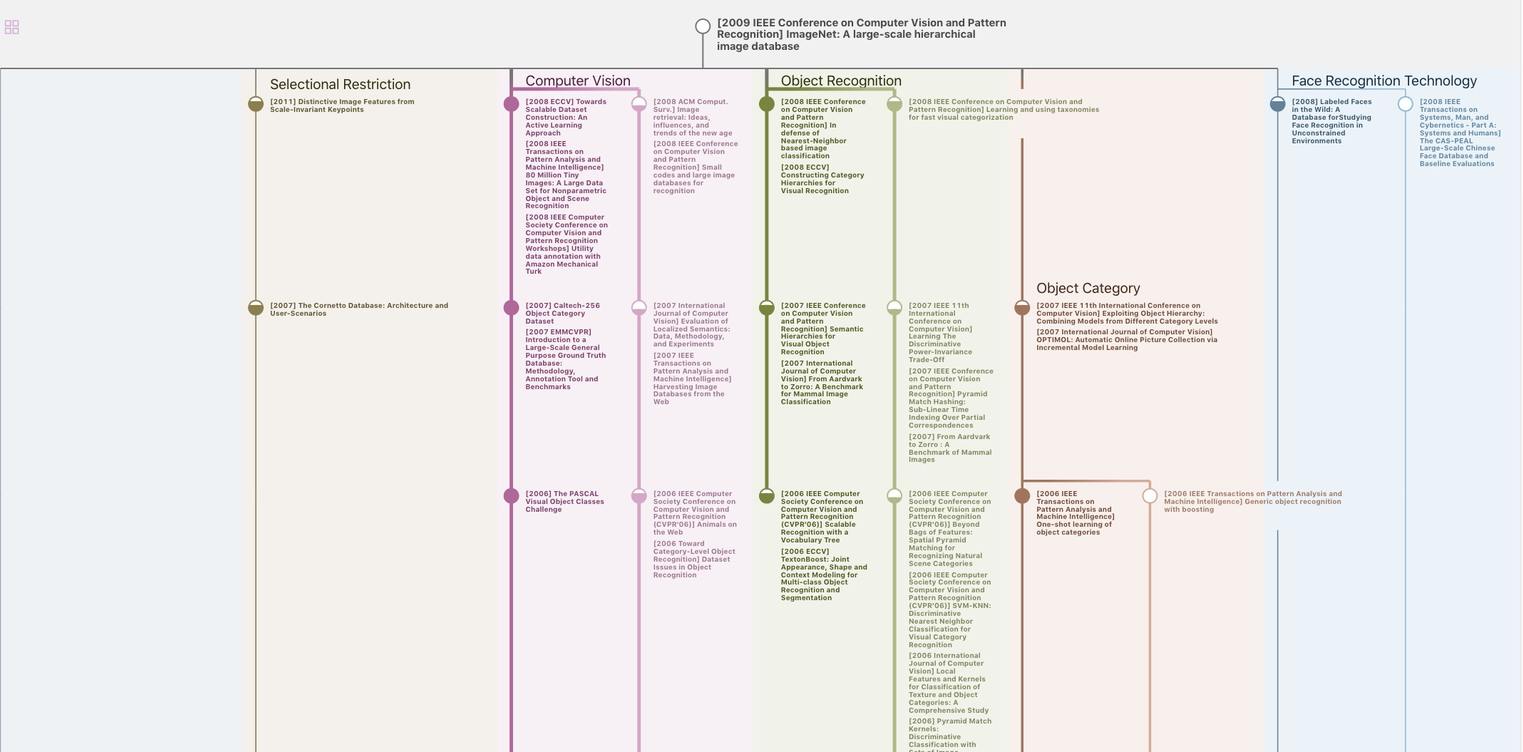
生成溯源树,研究论文发展脉络
Chat Paper
正在生成论文摘要