Tabular and latent space synthetic data generation: a literature review
J. Big Data(2023)
摘要
The generation of synthetic data can be used for anonymization, regularization, oversampling, semi-supervised learning, self-supervised learning, and several other tasks. Such broad potential motivated the development of new algorithms, specialized in data generation for specific data formats and Machine Learning (ML) tasks. However, one of the most common data formats used in industrial applications, tabular data, is generally overlooked; Literature analyses are scarce, state-of-the-art methods are spread across domains or ML tasks and there is little to no distinction among the main types of mechanism underlying synthetic data generation algorithms. In this paper, we analyze tabular and latent space synthetic data generation algorithms. Specifically, we propose a unified taxonomy as an extension and generalization of previous taxonomies, review 70 generation algorithms across six ML problems, distinguish the main generation mechanisms identified into six categories, describe each type of generation mechanism, discuss metrics to evaluate the quality of synthetic data and provide recommendations for future research. We expect this study to assist researchers and practitioners identify relevant gaps in the literature and design better and more informed practices with synthetic data.
更多查看译文
关键词
Synthetic Data,Tabular data,Data privacy,Regularization,Oversampling,Active Learning
AI 理解论文
溯源树
样例
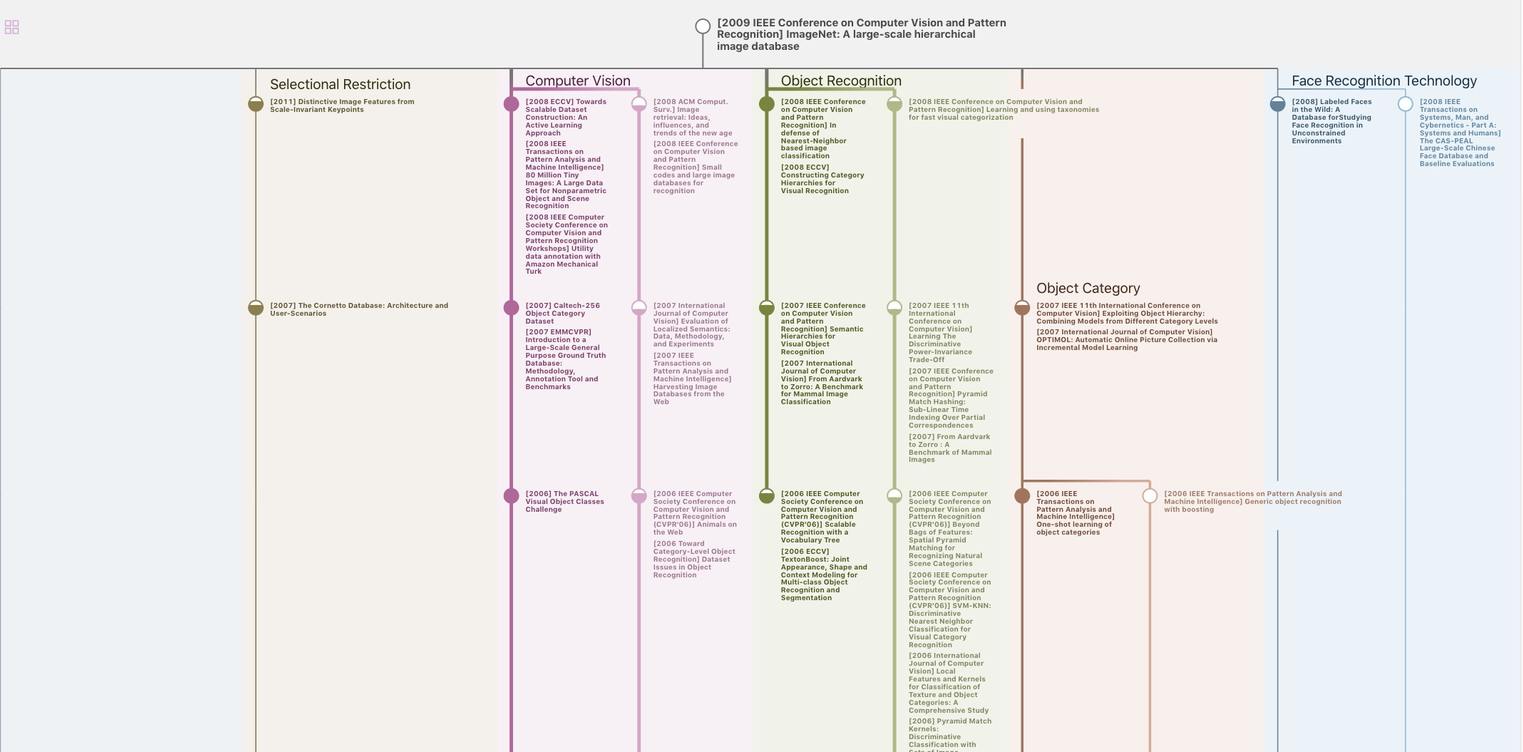
生成溯源树,研究论文发展脉络
Chat Paper
正在生成论文摘要