SS-VPE: Semi-Supervised Variational Prototyping Encoder With Student's-t Mixture Model.
IEEE Trans. Instrum. Meas.(2023)
摘要
As humans can identify new targets with one or a few images, machine learning can use a few images to generalize effective representations so as to achieve few-shot learning. Previous research has established relationships between visual prototypes in input space and feature representations in latent space to capture data distributions. However, such visual prototypes that can compactly represent class semantics are often lacking in most datasets. This study is aimed at distinguishing samples by using a latent prototype that represents class information in latent space. However, a latent prototype is mostly sensitive to outliers; therefore, a general method using a semi-supervised variational prototyping encoder (SS-VPE) is proposed. The contributions of this study are threefold. First, a generative unsupervised learning is employed to optimize the prototypes in latent space, such that the data distribution can be captured accurately. Second, the Student's-t mixture model (STMM) is applied to the above clustering in the latent space, resulting in high robustness against outliers. Finally, the conventional variational autoencoder (VAE) is advanced by few-shot semi-supervised learning. Intensive experiments are conducted on four popular benchmark datasets, and the experimental results show that our proposed SS-VPE outperforms the state-of-the-art methods, significantly improving both the clustering and classification ability of few-shot learning tasks.
更多查看译文
关键词
mixture,model,ss-vpe,semi-supervised
AI 理解论文
溯源树
样例
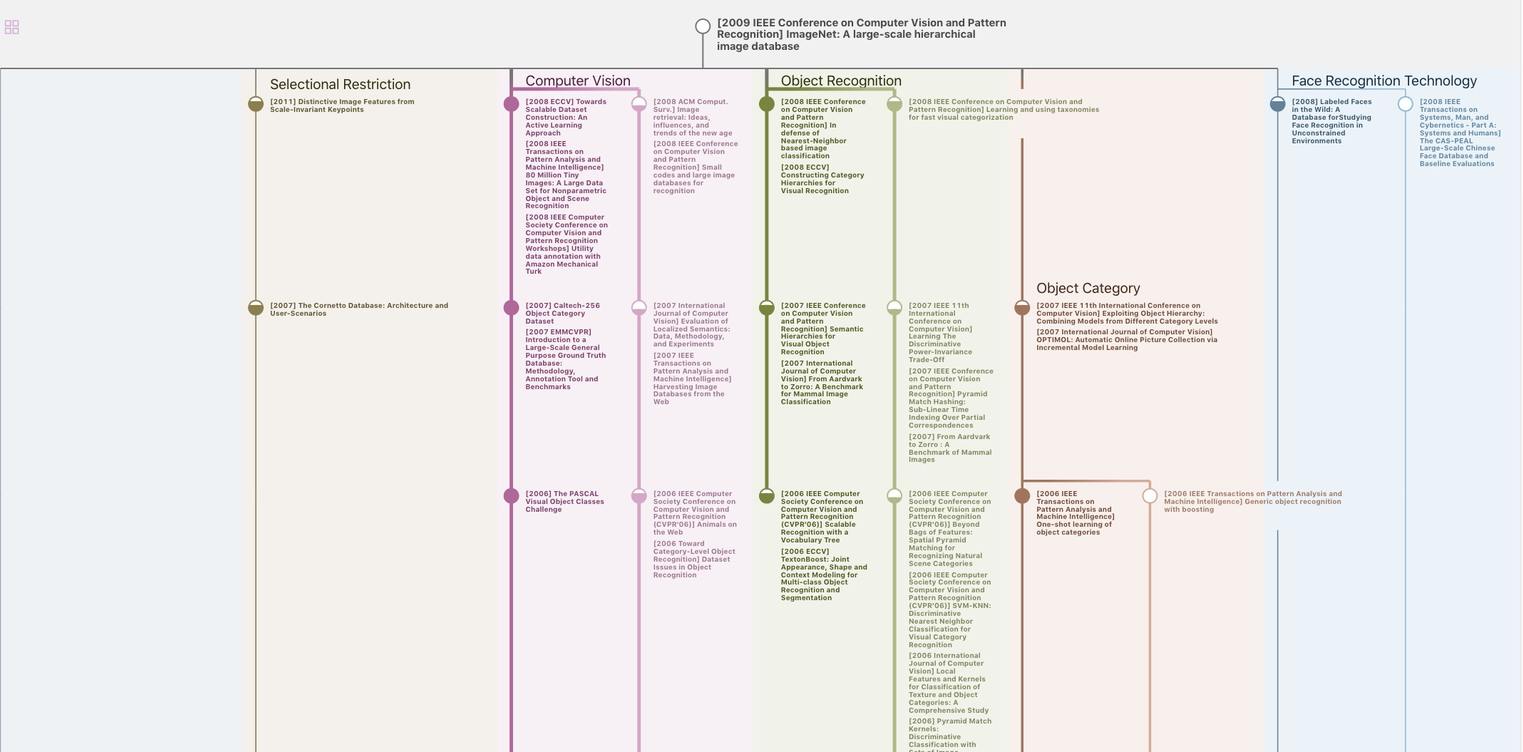
生成溯源树,研究论文发展脉络
Chat Paper
正在生成论文摘要