An Intelligent Hybrid Neural Collaborative Filtering Approach for True Recommendations.
IEEE Access(2023)
摘要
Recommendation services become a critical and hot research topic for researchers. A recommendation agent that automatically suggests products to users according to their tastes or preferences instead of wandering in a huge corpus for a product. Social data such as Reviews play an important role in the recommendation of products. Improvement was achieved by neural network methods for capturing user and product information from a short text. However, such approaches do not fairly and efficiently incorporate users' preferences and product characteristics. We are proposing the novel Hybrid Neural Collaborative Filtering (HNCF) model that combines deep learning capabilities and deep interaction modelling for recommender systems with a rating matrix. To overcome the cold start problem, we use the new overall rating by aggregating the Deep Multivariate Rating DMR (votes, likes, stars and sentiment scores of reviews) from different external data sources because different sites have different rating scores about the same product. The propose novel model consists of four major modules HUAPA-DCF+NSC+DMR (Hierarchical User Attention and Hierarchical Product Attention, Deep Collaborative Filtering, Neural Sentiment Classifier, and Deep Multivariant Rating) to solve the addressed problems. Initially, the HUAPA module is based on BiLSTM's hierarchical user attention (HUA) and hierarchical product attention (HPA) to embed the user preferences and product characteristics respectively. Further, these are combined nonlinear representations and fed as input to the interaction module. Secondly, the deep collaborative filtering module is implemented to find the explicit interaction between user and product. Thirdly, NSC module will extract the user's semantic about products by incorporating the user's preferences and product characteristics. Finally, the module uses explicit information (Deep Multivariant Rating) to the maximum extent for final classification. Experimental results demonstration that our novel model is outperforming than state-of-the-art at IMDb, Yelp2013 and Yelp2014 datasets for the true recommendation of top-n products using HNCF (HUAPA+DCF+NSC+DMR) to increase the accuracy, confidence, and trust on recommendation services.
更多查看译文
关键词
true recommendations
AI 理解论文
溯源树
样例
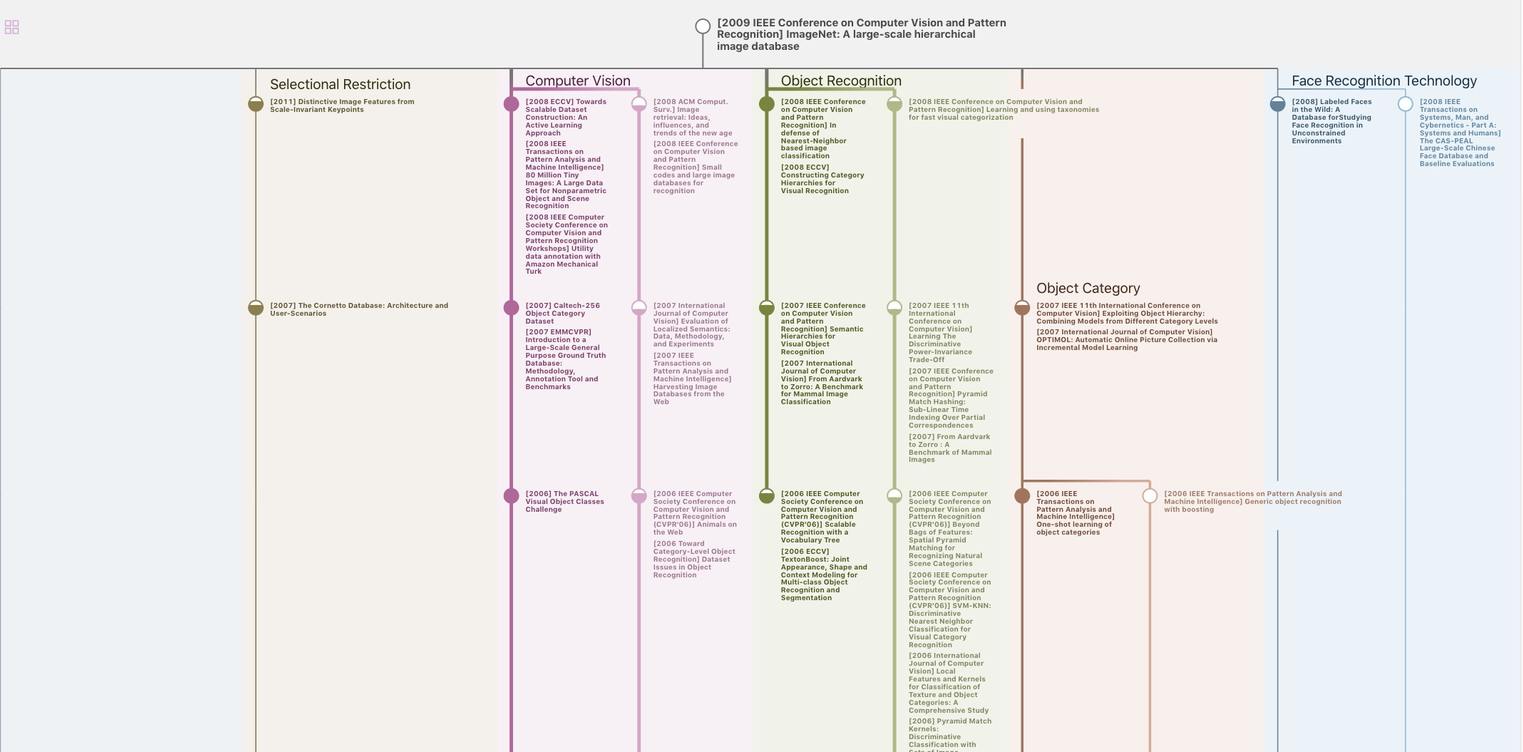
生成溯源树,研究论文发展脉络
Chat Paper
正在生成论文摘要