Colliding Bodies Optimization With Deep Belief Network Based Robust Pedestrian Detection.
IEEE Access(2023)
摘要
Pedestrian detection is a significant research topic in the computer vision (CV) domain for a longer period. Recently, deep learning (DL) and specifically convolutional neural network (CNN) exhibit significant improvement in the computer vision tasks such as object detection, segmentation, image classification, etc. With this motivation, this study develops a novel Colliding Bodies Optimization with Deep Learning based Robust Pedestrian Detection (CBODL-RPD) model. The goal of the CBODL-RPD approach is to identify the occurrence of pedestrians and non-pedestrians via object detection process. For object detection process, YOLO v4 with Adagrad optimizer is applied. In addition, the CBODL-RPD technique employs SqueezeNet model to generate feature vectors, and the hyperparameter tuning process is performed via the CBO algorithm. At last, deep belief network (DBN) model is applied for accurate pedestrian detection. A comprehensive experimental analysis is made to demonstrate the significant pedestrian detection results of the CBODL-RPD technique. The comparative outcome study reported the improved outcomes of the CBODL-RPD method over other recent methods.
更多查看译文
关键词
Pedestrian detection, video analysis, computational intelligence, deep learning, parameter optimization
AI 理解论文
溯源树
样例
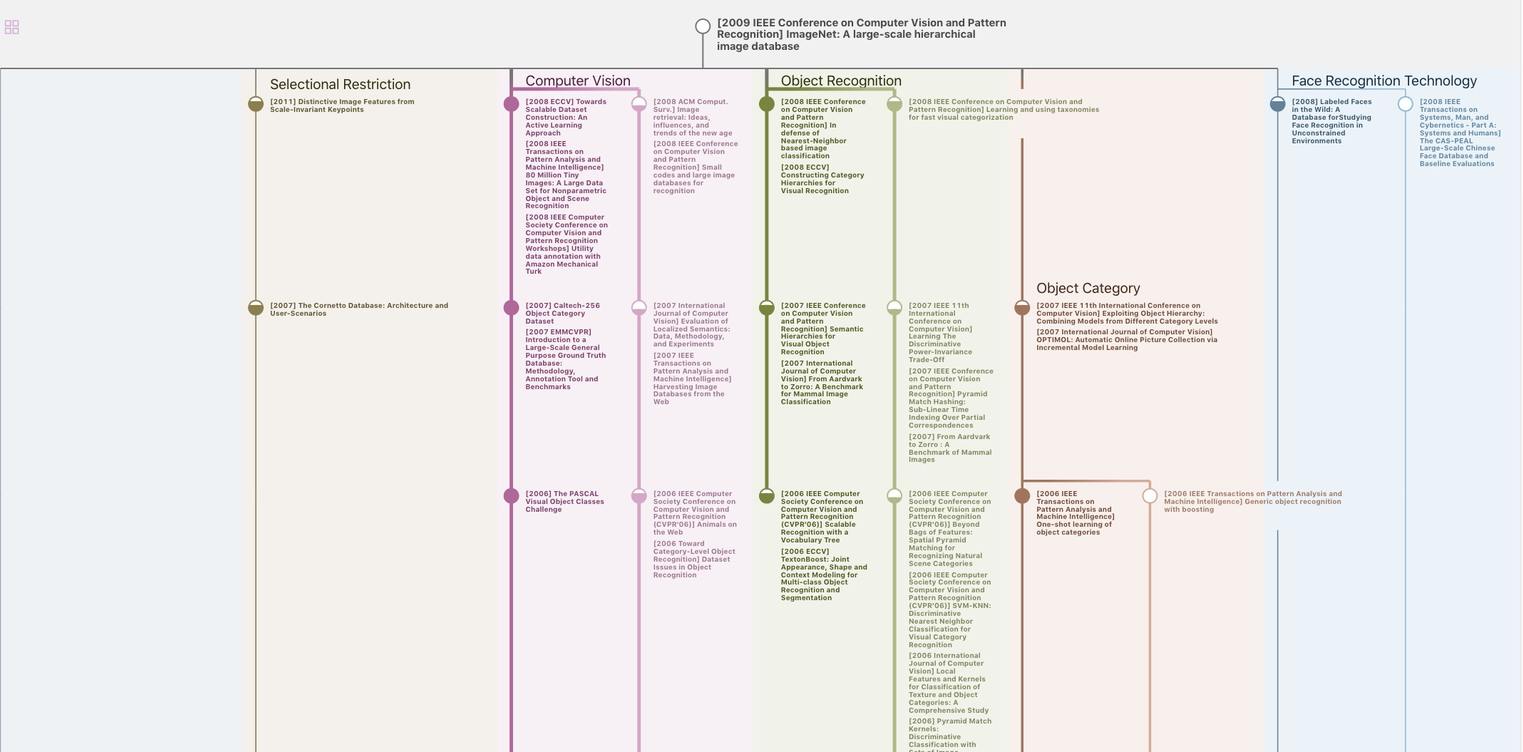
生成溯源树,研究论文发展脉络
Chat Paper
正在生成论文摘要