Implementing Privacy-Preserving and Collaborative Industrial Artificial Intelligence.
IEEE Access(2023)
摘要
Despite the growing connectivity and availability of sensor data boosted by the Industry 4.0 paradigm, data scarcity remains one of the biggest challenges for the widespread adoption of industrial AI, particularly regarding failure or defect data required for automated quality inspection solutions. Data sharing among different stakeholders is difficult due to data privacy, ownership, and cybersecurity concerns. Traditional centralized Machine Learning is already an essential part of several sectors, including smart manufacturing; however, it may lead to several issues regarding security and performance over time. In response to these problems, federated learning can be seen as a potential catalyst and enabler for the adoption of collaborative industrial artificial intelligence. It is an innovative and decentralized approach to machine learning, focused on collaboration and data privacy, shifting the information flow from the data to the models. Although considerable research efforts have been put forth towards this topic, there are still many unresolved issues concerning the application of federated learning approaches in smart manufacturing scenarios, including the lack of a common holistic reference framework and implementation. In this light, the present work aims to provide the foundation for a common framework for collaborative Industrial AI in smart manufacturing, addressing not only the collaboration aspect but also the main challenges of data privacy, ownership, and security. Additionally, we present and make publicly available a curated dataset focused on a manufacturing quality inspection scenario to further promote research on this topic.
更多查看译文
关键词
artificial intelligence,collaborative,privacy-preserving
AI 理解论文
溯源树
样例
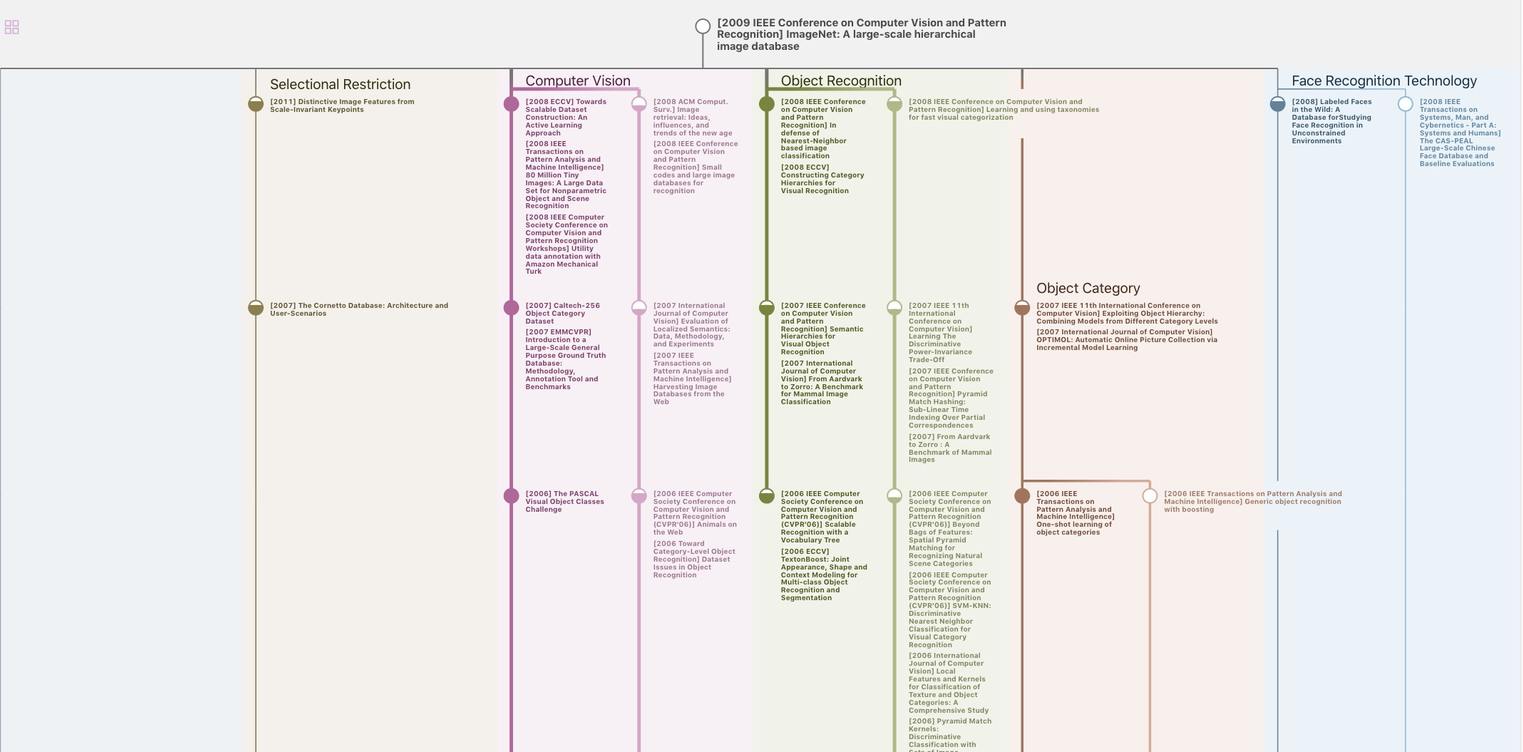
生成溯源树,研究论文发展脉络
Chat Paper
正在生成论文摘要