Darknet Traffic Analysis: Investigating the Impact of Modified Tor Traffic on Onion Service Traffic Classification.
IEEE Access(2023)
摘要
Classifying network traffic is important for traffic shaping and monitoring. In the last two decades, with the emergence of privacy concerns, the importance of privacy-preserving technologies has risen. The Tor network, which provides anonymity to its users and supports anonymous services known as Onion Services, is a popular way to achieve online anonymity. However, this anonymity (especially with Onion Services) is frequently misused, encouraging governments and law enforcement agencies to de-anonymise them. Therefore, in this paper, we try to identify the classifiability of Onion Service traffic, focusing on three main contributions. First, we try to identify Onion Service traffic from other Tor traffic. The techniques we have used can identify Onion Service traffic with >99% accuracy. However, there are several modifications that can be done to the Tor traffic to obfuscate its information leakage. In our second contribution, we evaluate how our techniques perform when such modifications have been done to the Tor traffic. Our experimental results show that these conditions make the Onion Service traffic less distinguishable (in some cases, the accuracy drops by more than 15%.) In our final contribution, we identify the most influential feature combinations for our classification problem and evaluate their impact.
更多查看译文
关键词
Traffic classification, machine learning, onion services, tor, anonymity, feature selection
AI 理解论文
溯源树
样例
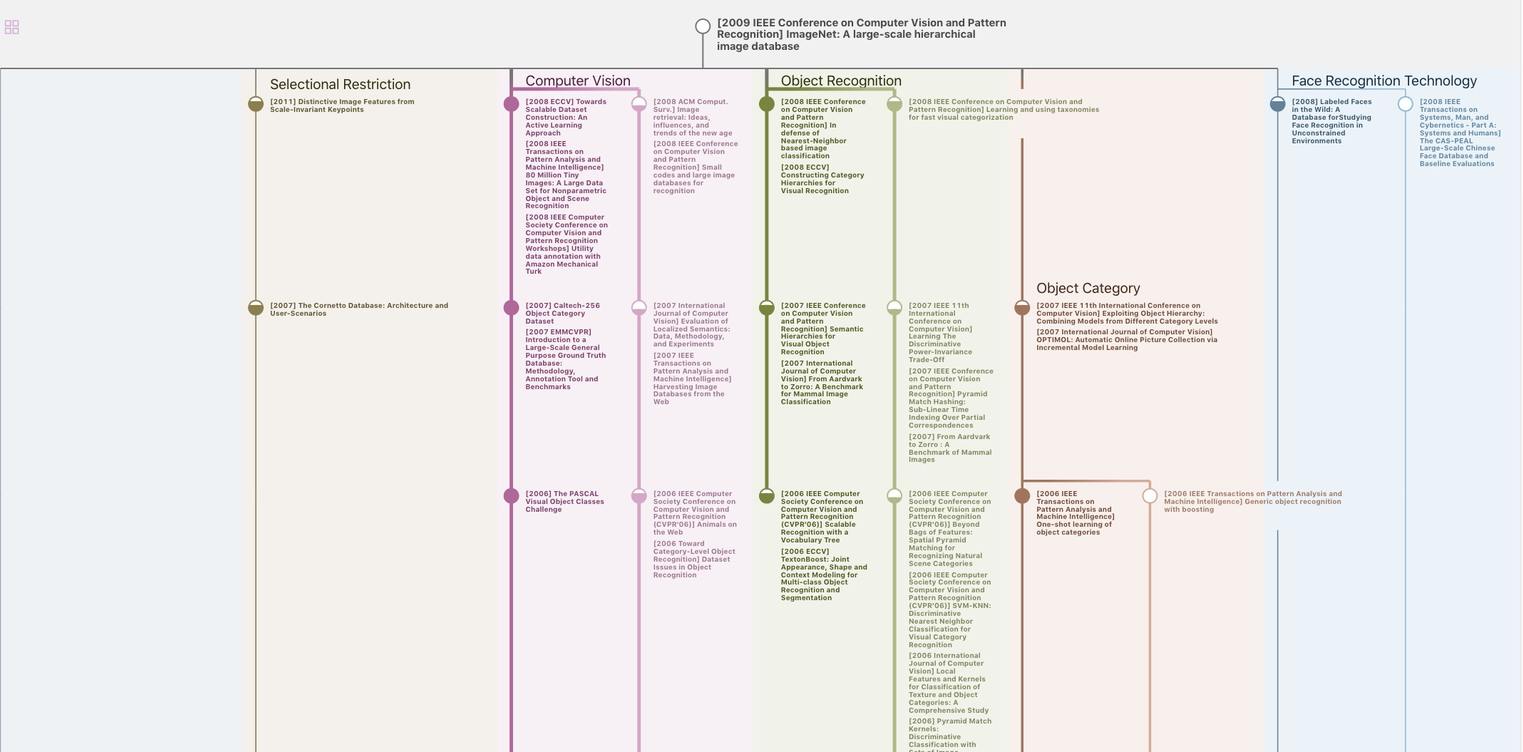
生成溯源树,研究论文发展脉络
Chat Paper
正在生成论文摘要