Efficient ML Lifecycle Transferring for Large-Scale and High-Dimensional Data via Core Set-Based Dataset Similarity.
IEEE Access(2023)
摘要
Developing an end-to-end machine learning (ML) lifecycle for an ML task can be costly and time-consuming. It involves exploring multiple configurations of ML pipelines, encompassing data preparation, ML model design, training, and deployment. While automated ML (AutoML) can assist in automatically searching and training an optimized ML pipeline, it is computationally intensive and lacks reusability for high-dimensional datasets. Transfer learning has emerged as a popular technique for fine-tuning pre-trained models on related datasets, yet it still requires manual tuning to achieve optimal results. To overcome these challenges, we present a version management system for the end-to-end ML lifecycle, enabling the transfer of lifecycle versions from similar datasets to new ML tasks. Specifically, we introduce an algorithm that leverages core sets to compute similarities for large-scale and high-dimensional datasets efficiently. To the best of our knowledge, we are the first to investigate ML lifecycle transfer for similar high-dimensional datasets. We conducted experiments on real-world datasets comprising computer vision and spatiotemporal sensor data. The experimental results demonstrate the effectiveness of our dataset similarity algorithm and the ML lifecycle version transferring procedure, reducing dataset similarity computation time by up to 60x while improving model accuracy compared to transfer learning. Furthermore, in a practical case study, our solution exhibited up to 3.5x greater efficiency in training time and memory consumption and 9% better model accuracy than manual tuning approaches.
更多查看译文
关键词
End-to-end ML lifecycle,high-dimensional dataset similarity,lifecycle transferring
AI 理解论文
溯源树
样例
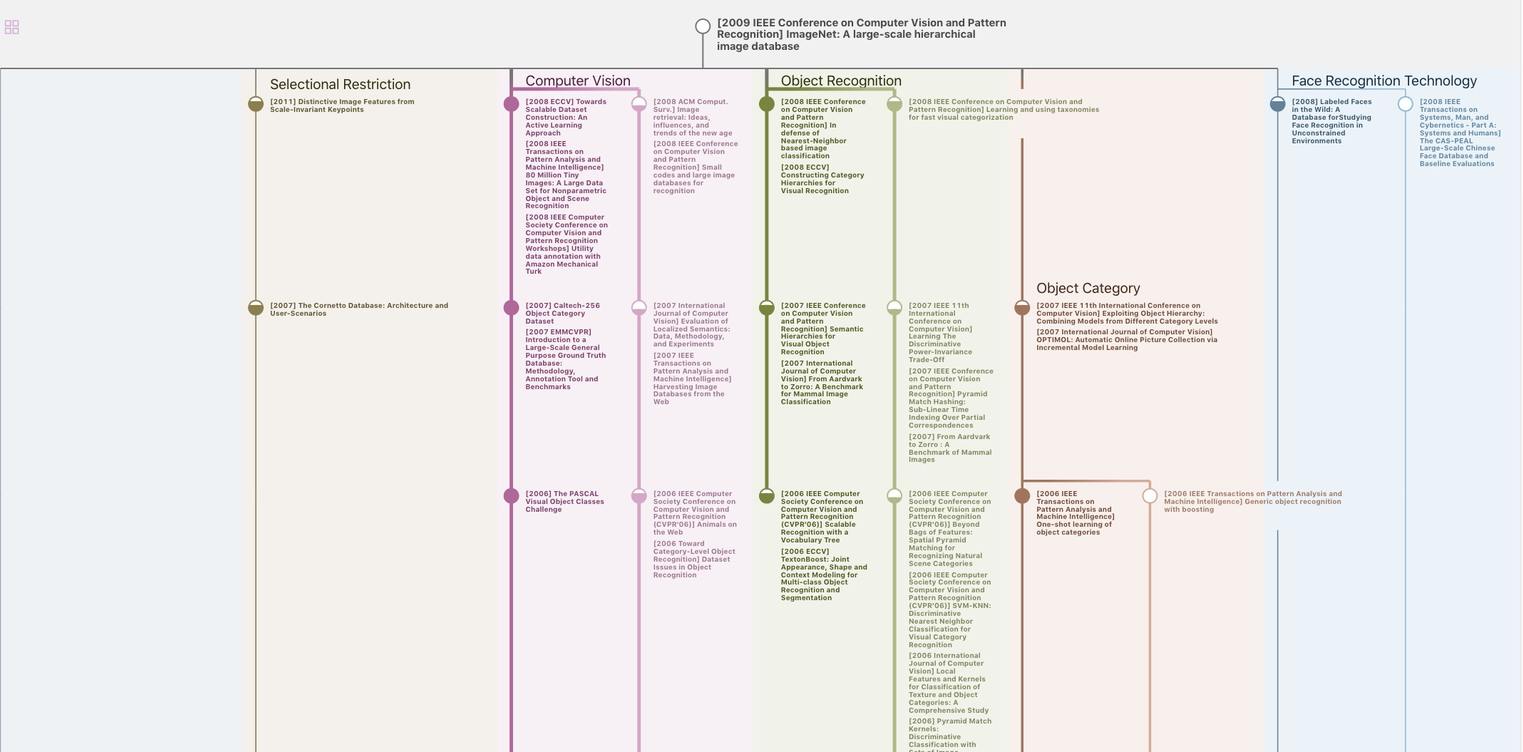
生成溯源树,研究论文发展脉络
Chat Paper
正在生成论文摘要