Enhancing Heart Disease Prediction through Ensemble Learning Techniques with Hyperparameter Optimization.
Algorithms(2023)
摘要
Heart disease is a significant global health issue, contributing to high morbidity and mortality rates. Early and accurate heart disease prediction is crucial for effectively preventing and managing the condition. However, this remains a challenging task to achieve. This study proposes a machine learning model that leverages various preprocessing steps, hyperparameter optimization techniques, and ensemble learning algorithms to predict heart disease. To evaluate the performance of our model, we merged three datasets from Kaggle that have similar features, creating a comprehensive dataset for analysis. By employing the extra tree classifier, normalizing the data, utilizing grid search cross-validation (CV) for hyperparameter optimization, and splitting the dataset with an 80:20 ratio for training and testing, our proposed approach achieved an impressive accuracy of 98.15%. These findings demonstrated the potential of our model for accurately predicting the presence or absence of heart disease. Such accurate predictions could significantly aid in early prevention, detection, and treatment, ultimately reducing the mortality and morbidity associated with heart disease.
更多查看译文
关键词
heart disease prediction,ensemble learning techniques
AI 理解论文
溯源树
样例
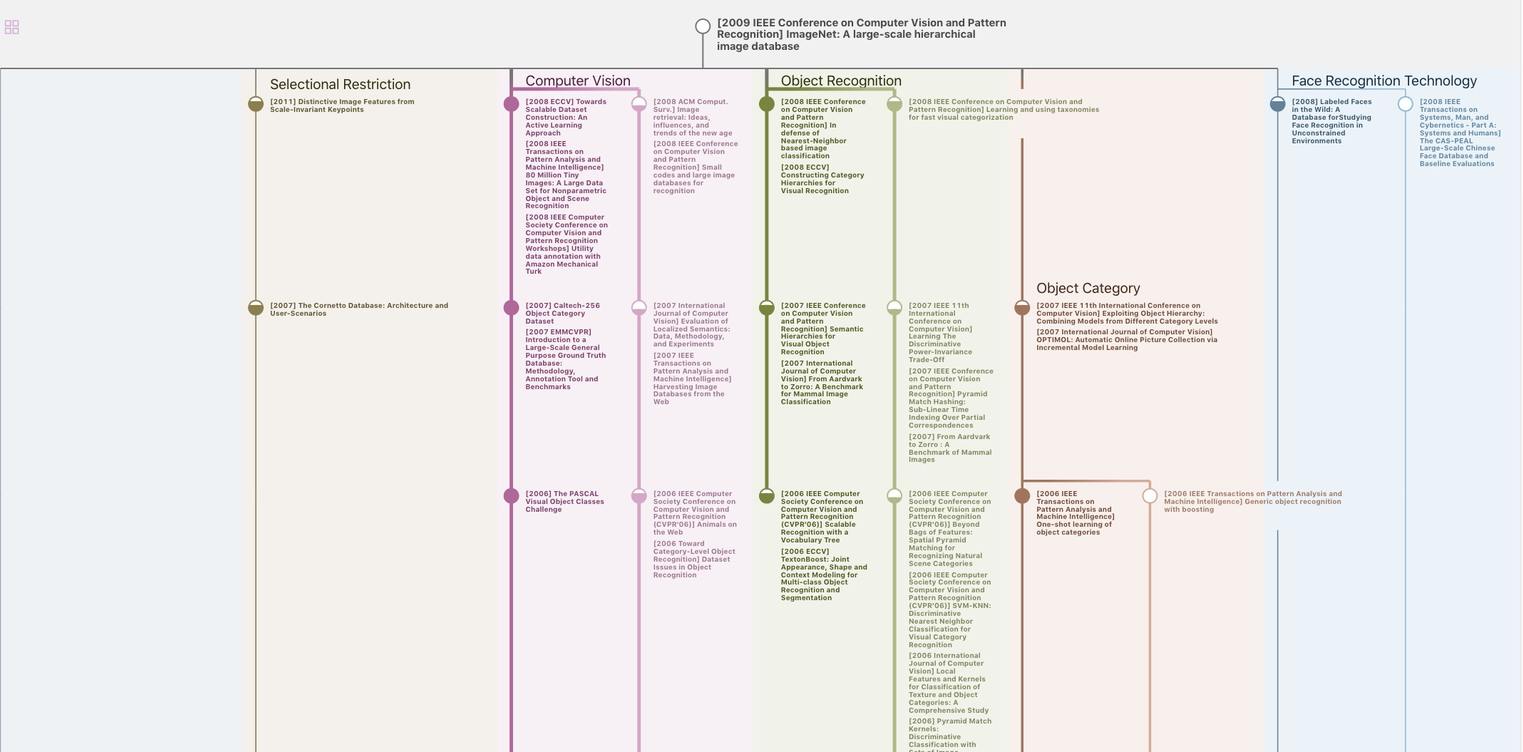
生成溯源树,研究论文发展脉络
Chat Paper
正在生成论文摘要