Detecting balling defects using multisource transfer learning in wire arc additive manufacturing.
J. Comput. Des. Eng.(2023)
摘要
Wire arc additive manufacturing (WAAM) has gained attention as a feasible process in large-scale metal additive manufacturing due to its high deposition rate, cost efficiency, and material diversity. However, WAAM induces a degree of uncertainty in the process stability and the part quality owing to its non-equilibrium thermal cycles and layer-by-layer stacking mechanism. Anomaly detection is therefore necessary for the quality monitoring of the parts. Most relevant studies have applied machine learning to derive data-driven models that detect defects through feature and pattern learning. However, acquiring sufficient data is time- and/or resource-intensive, which introduces a challenge to applying machine learning-based anomaly detection. This study proposes a multisource transfer learning method that generates anomaly detection models for balling defect detection, thus ensuring quality monitoring in WAAM. The proposed method uses convolutional neural network models to extract sufficient image features from multisource materials, then transfers and fine-tunes the models for anomaly detection in the target material. Stepwise learning is applied to extract image features sequentially from individual source materials, and composite learning is employed to assign the optimal frozen ratio for converging transferred and present features. Experiments were performed using a gas tungsten arc welding-based WAAM process to validate the classification accuracy of the models using low-carbon steel, stainless steel, and Inconel.
更多查看译文
关键词
wire arc additive manufacturing, anomaly detection, transfer learning, convolutional neural networks, domain adaptation
AI 理解论文
溯源树
样例
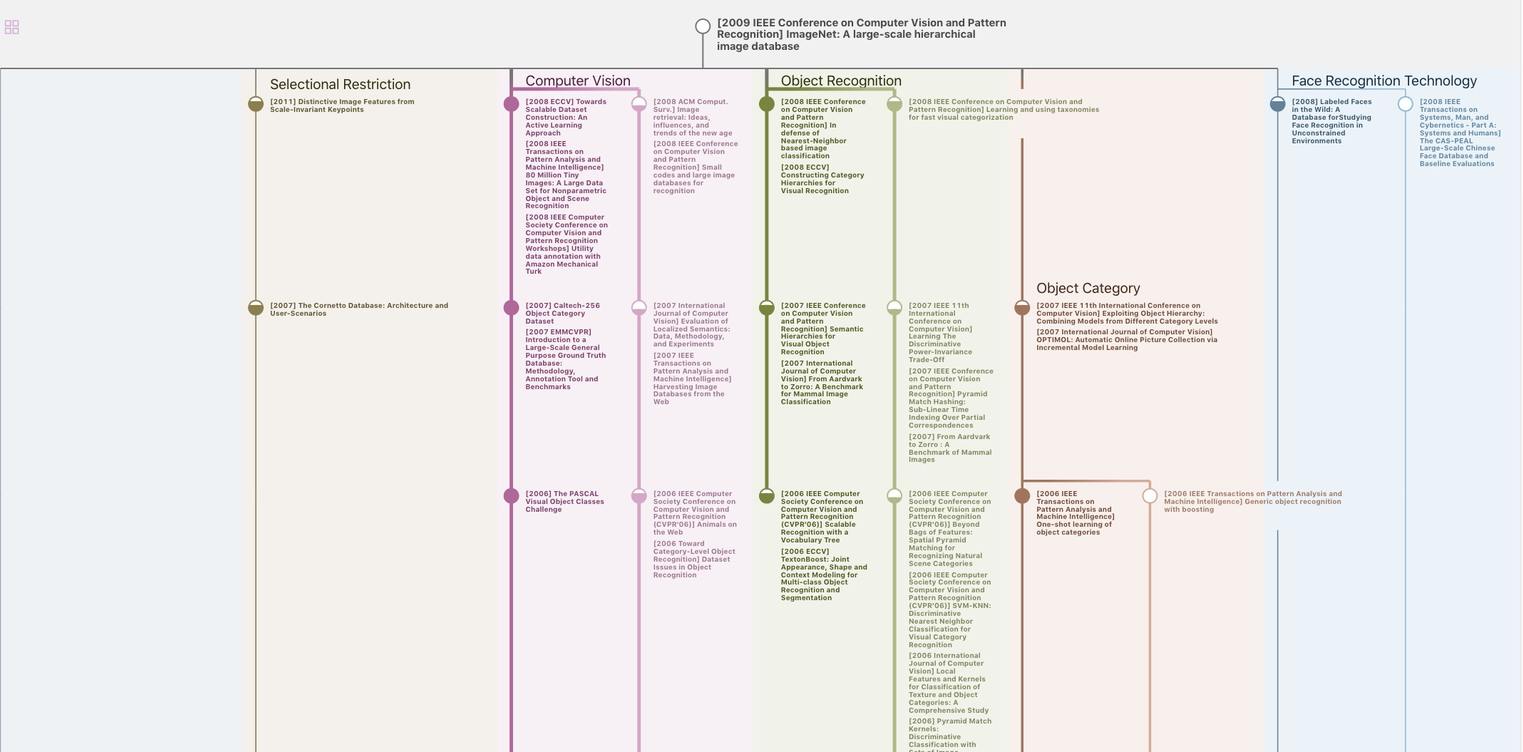
生成溯源树,研究论文发展脉络
Chat Paper
正在生成论文摘要